Safeguarding Your Business: Preventing Fraud with Big Data Analytics Techniques
In today’s rapidly evolving digital landscape, businesses face an increasing threat of fraud. Fortunately, leveraging big data analytics techniques can be a powerful tool in safeguarding your organization. You can uncover patterns and anomalies that indicate fraudulent activities by harnessing the vast amounts of data your operations generate.
One such technique is anomaly detection, which involves analyzing data to identify deviations from expected behavior. By establishing baseline patterns, anomalies can be detected and flagged for further investigation. For example, if your business typically receives a certain number of online transactions daily, a sudden spike or drop in activity could indicate fraudulent behavior.
Another powerful technique is predictive modeling, which uses historical data to forecast potential fraudulent events. By analyzing past instances of fraud and identifying common characteristics or patterns, predictive models can be developed to identify future fraudulent activities. This proactive approach enables businesses to take preventive measures and minimize potential losses.
Additionally, social network analysis can detect fraudulent networks or collusion. Suspicious associations can be identified by examining relationships and connections between entities, such as customers, employees, or suppliers. For instance, having multiple customer accounts linked to the same address or phone number may indicate fraudulent activity.
Implementing these big data analytics techniques requires a robust infrastructure and advanced analytics tools. By investing in state-of-the-art technology and skilled data analysts, businesses can significantly enhance their ability to prevent and detect fraud. This not only protects financial assets but also safeguards the reputation and trust of the organization.
Leveraging big data analytics techniques is essential for safeguarding your business against fraud. Anomaly detection, predictive modeling, and social network analysis can provide valuable insights and early warnings. By staying vigilant and harnessing the power of data, organizations can stay one step ahead of fraudsters and protect their interests effectively.
The Role of Big Data Analytics in Fraud Prevention
In the battle against fraud, big data analytics plays a pivotal role in preventing and combating fraudulent activities. Organizations can proactively identify and mitigate potential risks by harnessing the power of vast and diverse data sets. Here’s how big data analytics is revolutionizing fraud prevention:
- Detection of Anomalies: Big data analytics enables the identification of unusual patterns and outliers in large data sets. By establishing baseline behavior, sophisticated algorithms can detect deviations that may indicate fraudulent activities. For instance, if a customer suddenly exhibits unusual purchasing behavior or multiple transactions originate from suspicious locations, these anomalies can raise red flags for further investigation.
- Real-time Monitoring: With the ability to process and analyze data in real-time, big data analytics empowers organizations to monitor transactions and activities as they occur. This real-time monitoring immediately detects suspicious events, such as fraudulent credit card transactions or unauthorized access attempts. By promptly identifying and responding to such incidents, organizations can minimize potential damages.
- Predictive Analytics: Big data analytics leverages historical data to develop predictive models to forecast potential fraudulent behavior. Organizations can proactively identify high-risk transactions or entities by analyzing past fraud patterns and identifying common indicators. These predictive models enable businesses to take preventive measures, such as implementing additional security measures or flagging suspicious accounts for manual review.
- Network Analysis: Fraudsters often operate in intricate networks and conspire with others to carry out fraudulent activities. Big data analytics helps uncover these networks by analyzing connections and relationships between entities. By examining data such as customer interactions, financial transactions, or employee affiliations, organizations can identify suspicious links and take appropriate action.
- Enhanced Risk Assessment: Big data analytics provides a comprehensive view of risk factors by integrating various data sources. Organizations can gain insights into potential risks by combining internal and external data, such as transactional data, social media activity, and public records. This holistic risk assessment helps identify emerging fraud trends, adapt fraud prevention strategies, and strengthen security.
Big data analytics is a game-changer in fraud prevention. By leveraging its capabilities for anomaly detection, real-time monitoring, predictive analytics, network analysis, and risk assessment, organizations can stay ahead of fraudsters and safeguard their assets. Embracing big data analytics as an integral part of the fraud prevention strategy is crucial to protect businesses and maintaining trust in an increasingly complex digital landscape.
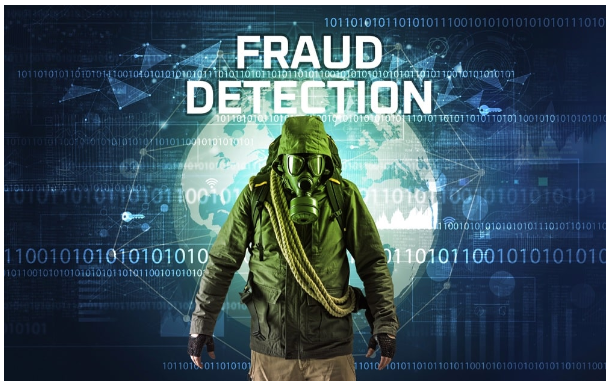
Understanding Big Data Analytics for Fraud Detection
Big data analytics plays a crucial role in detecting and preventing fraud, leveraging the power of large and complex datasets to uncover hidden patterns, anomalies, and indicators of fraudulent activities. Here’s a breakdown of key concepts in understanding big data analytics for fraud detection:
- Data Collection and Integration: Big data analytics begins with the collection and integration of diverse data sources, including transactional data, customer information, device logs, and external data feeds. This process involves aggregating and organizing data from various structured and unstructured sources to form a comprehensive view of potential fraudulent activities.
- Data Preprocessing and Cleansing: Data preprocessing steps ensure data quality before analysis. This includes removing duplicates, handling missing values, standardizing formats, and normalizing data. Cleansing the data helps improve the accuracy and reliability of subsequent studies.
- Exploratory Data Analysis (EDA): EDA involves exploring and visualizing the data to gain insights and identify potential patterns or outliers. Visualization techniques like charts, graphs, and heatmaps help understand data distributions, relationships, and anomalies.
- Anomaly Detection: Anomaly detection techniques aim to identify data points or events that deviate significantly from expected or normal behavior. Statistical methods, machine learning algorithms, and pattern recognition techniques are applied to detect outliers or suspicious activities that may indicate fraud.
- Predictive Modeling: Predictive models use historical data to forecast future fraudulent events. Machine learning algorithms, such as logistic regression, decision trees, or neural networks, are trained on labeled datasets to identify patterns and predict the likelihood of fraud for new instances. These models help prioritize investigation efforts and allocate resources effectively.
- Social Network Analysis: Fraudsters often operate in networks or conspire with others. Social network analysis examines relationships and connections between entities, such as customers, employees, or suppliers, to detect suspicious associations. Organizations can uncover fraudulent activities by mapping the network structure and identifying clusters or unusual linkages.
- Real-time Monitoring and Alerting: Big data analytics enables real-time monitoring of transactions and activities to identify and respond promptly to potential fraud. Automated systems analyze streaming data and apply rules, algorithms, or machine learning models to detect suspicious patterns or behaviors. Alerts are generated for immediate investigation and intervention.
- Continuous Improvement: Big data analytics for fraud detection is an iterative process. As new data becomes available and fraud techniques evolve, models and algorithms must be continuously updated and refined. Ongoing monitoring, feedback loops, and learning from past experiences help improve the effectiveness of fraud detection systems.
By harnessing the power of big data analytics, organizations can stay proactive in detecting and preventing fraud. The combination of advanced analytics techniques, real-time monitoring, and continuous improvement helps identify fraudulent activities early, mitigate risks, and safeguard business operations and assets.
The Impact of Big Data Analytics on Fraud Mitigation
The impact of big data analytics on fraud mitigation is significant, revolutionizing how organizations combat fraudulent activities. By leveraging advanced analytics techniques and processing vast amounts of data, big data analytics offers several critical benefits for fraud mitigation:
- Early Detection: Big data analytics enables the early detection of fraudulent activities by analyzing large volumes of data in real-time. By monitoring transactions, behaviors, and patterns, organizations can identify suspicious activities and intervene before significant losses occur. This proactive approach minimizes financial damage and reduces the impact of fraud.
- Improved Accuracy: Traditional fraud detection methods often rely on manual processes and rule-based systems, which can be limited in detecting complex fraud patterns. Big data analytics, on the other hand, leverages machine learning algorithms and predictive models that continuously learn and adapt to evolving fraud techniques. This improves fraud detection accuracy, reducing false positives and negatives.
- Enhanced Fraud Risk Assessment: Big data analytics enables organizations to comprehensively understand fraud risks by analyzing diverse data sources. Organizations can identify hidden relationships, emerging fraud patterns, and potential vulnerabilities by integrating structured and unstructured data, such as transactional data, customer information, external feeds, and social media data. This holistic view allows for targeted mitigation strategies.
- Real-time Monitoring and Response: With big data analytics, organizations can monitor transactions and activities in real-time, applying advanced analytics algorithms to detect anomalies and suspicious behaviors. This real-time monitoring enables immediate response and intervention, preventing fraudulent activities and minimizing losses. Automated alerts and notifications facilitate prompt action.
- Network Analysis and Linkage Detection: Big data analytics helps identify fraud networks and collusion by analyzing relationships and connections between entities. Social network analysis techniques can uncover hidden links, common attributes, or clustering patterns that indicate fraudulent activities. By mapping these networks, organizations can identify high-risk individuals or groups and disrupt fraudulent operations.
- Scalability and Efficiency: Big data analytics provides scalability to handle the massive volumes of data generated in real-time transactions. It allows organizations to process and analyze data quickly, uncovering fraud patterns across millions of transactions. Organizations can efficiently detect and investigate fraud by automating the analytics process, saving time and resources.
- Continuous Improvement: Big data analytics enables continuous improvement in fraud mitigation efforts. Organizations can refine their models, algorithms, and rules by analyzing historical data and outcomes to adapt to new fraud techniques. Continuous monitoring, feedback loops, and learning from past experiences help organizations stay one step ahead of fraudsters.
Big data analytics has a transformative impact on fraud mitigation. It enables early detection, improves accuracy, provides a comprehensive view of fraud risks, facilitates real-time monitoring and response, detects fraud networks, and promotes continuous improvement. By leveraging big data analytics, organizations can strengthen their fraud mitigation strategies, protect their assets, and safeguard their reputation.
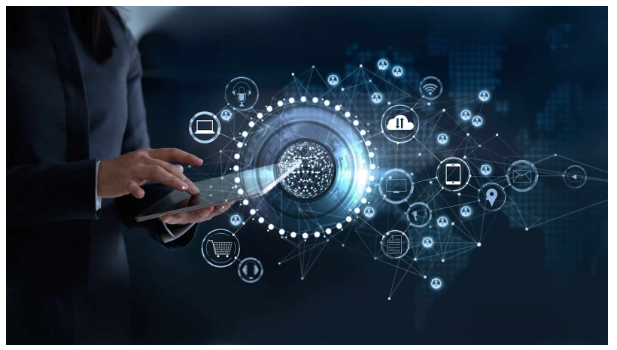
Leveraging Big Data Analytics Techniques for Fraud Prevention
Leveraging big data analytics techniques is instrumental in fraud prevention, enabling organizations to identify and mitigate fraudulent activities proactively. Here are some key techniques for using big data analytics in fraud prevention:
- Anomaly Detection: Big data analytics can identify anomalies in vast amounts of data by establishing standard patterns and detecting deviations. Statistical analysis, machine learning algorithms, and data visualization help identify unusual patterns, outliers, or suspicious behaviors that may indicate fraud. By flagging these anomalies, organizations can investigate further and take necessary actions to prevent fraud.
- Predictive Analytics: Predictive analytics uses historical data and machine learning algorithms to forecast potential fraudulent events. By analyzing past fraud patterns, transactional data, and customer behavior, predictive models can identify high-risk transactions or individuals. These models help organizations prioritize investigation efforts, allocate resources effectively, and prevent fraud before it occurs.
- Link Analysis and Social Network Analysis: Big data analytics techniques, such as link and social network analysis, help uncover hidden connections and relationships between entities involved in fraudulent activities. By analyzing transactional data, communication records, and online interactions, organizations can identify networks, clusters, or patterns of cooperation. This information is valuable for identifying and disrupting fraudulent operations.
- Text Mining and Natural Language Processing: Unstructured data, such as emails, social media posts, or customer feedback, can hold valuable insights for fraud prevention. Text mining and natural language processing techniques analyze text data to identify keywords, sentiments, or fraud-related patterns. Organizations can uncover potential fraud indicators and take appropriate measures by extracting relevant information from unstructured data sources.
- Real-time Monitoring and Alerting: Big data analytics enables real-time monitoring of transactions, activities, and system logs. Organizations can detect suspicious events in real time and generate immediate alerts by applying rule-based systems or machine-learning models. This allows prompt intervention, blocking fraudulent activities and minimizing potential losses.
- Data Visualization: Data visualization techniques help understand complex fraud-related data and present insights visually compellingly. Interactive dashboards, charts, and graphs allow fraud analysts to explore data, identify trends, and spot potential fraud patterns more effectively. Visualization enhances decision-making and facilitates communication across teams.
- Continuous Model Improvement: Big data analytics supports constantly improving fraud prevention models and algorithms. As fraudsters evolve their techniques, organizations must adapt their analytics approaches accordingly. Regularly updating and refining models based on new data and feedback ensures that fraud prevention efforts stay practical and up-to-date.
Organizations can strengthen their fraud prevention strategies by leveraging these big data analytics techniques. Anomaly detection, predictive analytics, link analysis, text mining, real-time monitoring, data visualization, and continuous model improvement empower organizations to detect, prevent, and mitigate fraud effectively.
Pattern Recognition and Anomaly Detection
Pattern recognition and anomaly detection are critical techniques for fraud prevention and detection in leveraging big data analytics. These techniques involve analyzing large datasets to identify regular behavior patterns and detect deviations or anomalies that may indicate fraudulent activities. Here’s a closer look at these techniques:
- Pattern Recognition: Pattern recognition involves identifying recurring patterns or trends within a dataset. In fraud prevention, it helps establish normal behavior based on historical data. Organizations can identify typical patterns associated with legitimate activities by analyzing transactional data, customer behavior, or system logs. This knowledge forms the basis for detecting deviations from these patterns, which may signal potential fraud.
Machine learning algorithms, such as clustering or classification algorithms, are often applied in pattern recognition. These algorithms learn from historical data to identify common characteristics or features of specific transactions or behaviors. Organizations can flag potentially fraudulent activities by recognizing patterns that differ from the norm for further investigation. - Anomaly Detection: Anomaly detection identifies instances that deviate significantly from the established behavior patterns. It helps uncover irregular or suspicious activities that may indicate fraud. Anomalies can take various forms, such as unexpected transaction amounts, unusual access patterns, or atypical user behavior.
Statistical techniques, machine learning algorithms (such as isolation forest or one-class SVM), or data visualization methods are commonly used for anomaly detection. These approaches establish baselines of normal behavior and identify data points or events that fall outside these boundaries. Anomalies are then flagged for closer scrutiny or additional investigation. - Combining Pattern Recognition and Anomaly Detection: Pattern recognition and anomaly detection are often used to enhance fraud detection capabilities. Pattern recognition establishes expected behavior, while anomaly detection identifies deviations from these patterns. By combining these techniques, organizations can effectively detect both known fraud patterns and previously unseen fraudulent activities.
Moreover, leveraging big data analytics enables organizations to process and analyze vast amounts of data, including structured and unstructured data sources. This allows more accurate and comprehensive pattern recognition and anomaly detection, improving fraud prevention outcomes.
Pattern recognition and anomaly detection are crucial components of big data analytics for fraud prevention. These techniques enable organizations to identify regular patterns of behavior, establish baselines of normalcy, and effectively detect anomalies that may indicate fraudulent activities. By leveraging these techniques, organizations can enhance their ability to detect and prevent fraud, minimizing financial losses and protecting their assets.
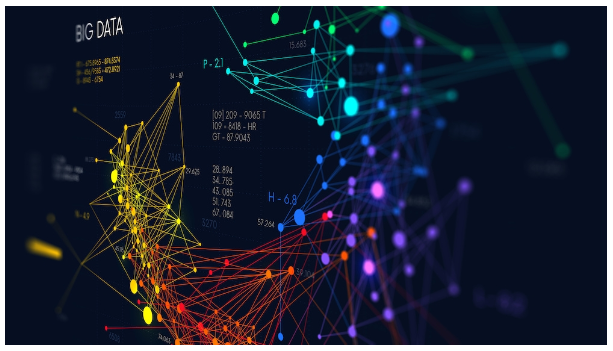
Predictive Modeling and Risk Scoring
Predictive modeling and risk scoring are powerful techniques used in big data analytics for fraud prevention and mitigation. These techniques involve leveraging historical data to develop models that predict the likelihood of fraud and assign risk scores to various entities or transactions. Here’s an overview of predictive modeling and risk scoring in the context of fraud prevention:
Predictive Modeling:
Predictive modeling involves using historical data to build models that can make predictions about future events. In the context of fraud prevention, predictive models analyze past instances of fraud and non-fraudulent transactions to identify patterns, trends, and characteristics associated with fraudulent activities. These models are trained on labeled datasets, where fraudulent transactions are identified, to learn from the data and predict the likelihood of fraud for new or unseen instances.
Machine learning algorithms, such as logistic regression, decision trees, random forests, or neural networks, are commonly employed in predictive modeling. These algorithms identify patterns and relationships between various attributes or features of transactions, customers, or entities. Predictive models assign probabilities or scores that indicate the likelihood of fraudulent activity by considering transaction amount, location, time, customer behavior, or device information.
Risk Scoring:
Risk scoring involves assigning a numerical value or score to transactions, customers, or entities to quantify risk level. These scores are typically derived from predictive models and reflect the likelihood of fraudulent behavior. Higher scores indicate a higher risk of fraud, while lower scores indicate a lower risk.
Risk scores are calculated based on the predictions made by the predictive models. The models analyze the attributes or features of a transaction or entity and generate a score representing the probability of fraud. These scores can be used to prioritize investigations, allocate resources, or trigger additional security measures for high-risk transactions or entities.
By incorporating risk scoring into fraud prevention systems, organizations can effectively focus on high-risk areas, improving operational efficiency and enhancing fraud detection capabilities.
Predictive modeling and risk scoring offer several benefits for fraud prevention:
- Early Detection: Predictive models can identify fraudulent activities early, allowing organizations to take proactive measures to prevent or minimize losses.
- Efficient Resource Allocation: Risk scores help prioritize investigations and allocate resources to high-risk transactions or entities, optimizing fraud prevention efforts.
- Reduced False Positives: Predictive models and risk scoring help minimize false favorable rates by accurately identifying high-risk transactions while reducing unnecessary scrutiny of legitimate activities.
- Adaptability: Predictive models can be continually refined and updated based on new data, allowing organizations to adapt to evolving fraud patterns and techniques.
- Integration with Real-Time Systems: Predictive models and risk scoring can be integrated into real-time fraud prevention systems, enabling immediate risk assessment and timely decision-making.
Predictive modeling and risk scoring are essential techniques in big data analytics for fraud prevention. They leverage historical data to predict the likelihood of fraud and assign risk scores to transactions, customers, or entities. By incorporating these techniques, organizations can improve fraud detection accuracy, prioritize investigations, and enhance fraud prevention strategies.
Implementing Effective Big Data Analytics for Fraud Prevention
Implementing effective big data analytics for fraud prevention involves several key steps and considerations. Here’s a roadmap to guide the implementation process:
- Define Goals and Objectives: Clearly define your fraud prevention goals and objectives. Identify the specific types of fraud you want to target, the desired outcomes, and the metrics to measure success.
- Data Collection and Integration: Identify the relevant data sources for fraud prevention, including transactional data, customer information, logs, and external feeds. Establish mechanisms to collect and integrate data from these sources into a centralized data repository or data lake.
- Data Quality and Cleansing: Ensure data quality by implementing data cleansing and preprocessing techniques. Remove duplicates, handle missing values, standardize formats, and ensure data accuracy and consistency.
- Infrastructure and Tools: Invest in a robust infrastructure that handles large-scale data processing and analytics. Implement big data platforms like Apache, Hadoop, or Spark and leverage appropriate tools, frameworks, and programming languages (e.g., Python, R) for data analysis and modeling.
- Analytics Techniques Selection: Choose the appropriate analytics techniques based on your fraud prevention goals. This may include pattern recognition, anomaly detection, predictive modeling, social network analysis, or text mining. Select the algorithms, models, and methodologies best suit your data and objectives.
- Model Development and Training: Develop and train predictive models using historical data. Employ machine learning algorithms, such as logistic regression, decision trees, or neural networks, to identify patterns and predict fraud likelihood. Train the models using labeled datasets that include both fraudulent and non-fraudulent instances.
- Real-time Monitoring and Alerting: Implement real-time monitoring systems that analyze transactions and activities as they occur. Apply analytics models or rule-based systems to detect suspicious patterns or behaviors. Generate alerts and notifications for immediate investigation and intervention.
- Risk Scoring and Prioritization: Develop risk scoring mechanisms based on predictive models to assign risk scores to transactions, customers, or entities. Prioritize investigations based on these risk scores, focusing resources on high-risk areas.
- Continuous Monitoring and Improvement: Continuously monitor the performance of the analytics system. Collect feedback, evaluate model accuracy, and incorporate new data to refine and improve the models. Stay updated with emerging fraud trends and adapt the analytics approach accordingly.
- Collaboration and Integration: Foster collaboration between fraud prevention teams, data analysts, IT departments, and stakeholders. Ensure seamless integration between the analytics system and existing fraud prevention processes, systems, and security measures.
- Compliance and Privacy: Ensure compliance with relevant regulations and privacy guidelines when handling sensitive data. Implement proper data governance and security measures to protect data integrity and confidentiality.
- Training and Skill Development: Provide training and skill development opportunities for your teams involved in big data analytics for fraud prevention. Enhance their knowledge in data analysis, modeling, and fraud detection techniques.
Organizations can implement an effective big data analytics framework for fraud prevention by following these steps. It allows for proactive fraud detection, timely interventions, and continuous improvement to stay ahead of evolving fraud techniques and protect business operations and assets.
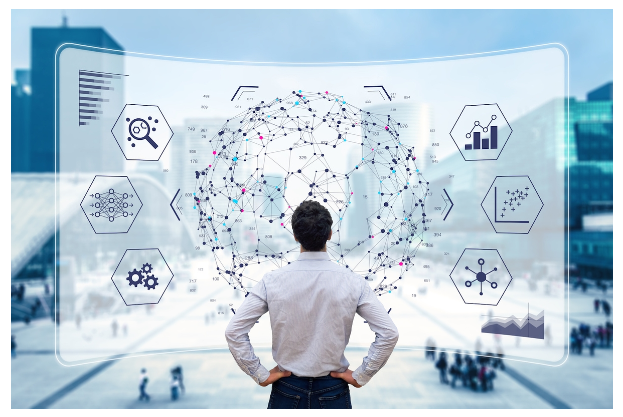
Data Integration and Data Quality Assurance
Data integration and data quality assurance are crucial aspects of implementing effective big data analytics for fraud prevention. Here’s a closer look at these two components:
Data Integration:
Data integration involves collecting and combining data from various sources into a centralized repository or data lake. It gives organizations a unified view of data for comprehensive fraud analysis. Here are some key considerations for data integration:
- Identify Data Sources: Determine the relevant data sources for fraud prevention, such as transactional data, customer information, logs, external feeds, or social media data. Ensure you have access to these sources and establish mechanisms to retrieve and integrate the data.
- Data Extraction and Transformation: Extract data from different sources and transform it into a consistent format that can be easily integrated. This may involve converting data types, standardizing units, or harmonizing data structures.
- Data Mapping and Schema Design: Develop a data mapping plan to align the data elements from various sources into a unified schema. Define the relationships and connections between data elements to enable practical analysis and querying.
- Data Integration Tools and Technologies: Select appropriate tools and technologies that suit your organizational requirements. This may include Extract, Transform, Load (ETL) tools, data integration platforms, or frameworks like Apache Kafka or Apache Nifi.
- Data Governance and Security: Implement practices to ensure data quality, consistency, and security throughout the integration process. Define data ownership, access controls, and privacy policies to protect sensitive information.
Data Quality Assurance:
Data quality assurance ensures the data’s accuracy, completeness, and reliability for fraud prevention. Poor data quality can lead to inaccurate analysis and ineffective fraud detection. Consider the following steps for data quality assurance:
- Data Cleansing: Identify and address data quality issues, such as duplicate records, missing values, or inconsistent data formats. Apply data cleansing techniques to improve data accuracy and completeness.
- Data Validation and Verification: Implement data validation checks to verify the integrity and correctness of the data. This may involve cross-referencing data with external sources, performing data integrity checks, or applying validation rules to detect inconsistencies.
- Data Profiling and Monitoring: Profile the data to gain insights into its quality characteristics, such as completeness, uniqueness, or distribution. Continuously monitor data quality metrics and set up alerts for anomalies or deviations from expected patterns.
- Data Quality Metrics and KPIs: Define data quality metrics and key performance indicators (KPIs) to measure and track data quality over time. This helps assess the effectiveness of data quality initiatives and identifies areas for improvement.
- Data Quality Governance: Establish practices to ensure ongoing data quality management. Assign responsibilities, define data quality standards, and establish processes for data quality issue resolution and continuous improvement.
- Data Quality Feedback Loop: Establish feedback loops with data providers and data consumers to capture and address data quality issues. Encourage users to report data discrepancies or anomalies they encounter during their analysis.
By effectively integrating data from multiple sources and ensuring data quality, organizations can enhance the accuracy and reliability of their fraud prevention analytics. This, in turn, leads to more accurate fraud detection, reduced false positives, and improved overall fraud prevention outcomes.
Collaborative Approaches and Automation
Collaborative approaches and automation are key to implementing effective big data analytics for fraud prevention. They enhance the efficiency, accuracy, and agility of fraud prevention efforts. Here’s how collaborative approaches and automation contribute to the success of fraud prevention initiatives:
- Collaborative Approaches: Cross-Functional Collaboration: Foster collaboration between different teams and departments involved in fraud prevention, such as fraud analysts, data scientists, IT professionals, legal teams, and business stakeholders. Encourage regular communication, knowledge sharing, and joint problem-solving to leverage diverse expertise and perspectives.
- Information Sharing: Establish mechanisms for sharing relevant information and insights across teams. Share fraud detection techniques, emerging fraud trends, and lessons learned from past incidents. Collaborative platforms or tools can facilitate real-time information exchange and collaboration.
- Data Sharing and Collaboration: Encourage data sharing and collaboration between organizations or entities to combat fraud collectively. Collaborative networks or industry alliances can share anonymized or aggregated data to identify broader fraud patterns, detect cross-organizational fraud schemes, and enhance fraud prevention effectiveness.
- External Partnerships: Forge partnerships with external organizations, such as law enforcement agencies, industry associations, or fraud prevention service providers. Collaborate to exchange intelligence, share best practices, and leverage their expertise and resources in combating fraud.
- Automation: Real-Time Monitoring and Alerting: Implement automated systems that continuously monitor real-time transactions, activities, or system logs. Use rule-based or machine-learning algorithms to detect suspicious patterns, behaviors, or anomalies. Generate automated alerts and notifications for immediate investigation and intervention.
- Workflow Automation: Automate routine tasks and workflows in fraud prevention, such as data preprocessing, data enrichment, or repetitive analysis tasks. This saves time and allows fraud analysts to focus on more complex analysis and decision-making.
- Model Training and Deployment: Automate the model training and deployment process to ensure models are up-to-date and running efficiently. Implement workflows that periodically retrain models using new data, evaluate their performance, and deploy updated models seamlessly.
- Case Management and Incident Response: Utilize automated case management systems to handle fraud investigation processes. These systems can track and manage cases, assign tasks to relevant personnel, and provide a centralized repository for evidence and documentation.
- Reporting and Visualization: Automate the generation of fraud prevention reports and visualizations to provide timely insights to stakeholders. Interactive dashboards, automated report generation, and data visualization tools allow for the efficient and user-friendly presentation of fraud analytics findings.
- Continuous Monitoring and Adaptation: Implement automated monitoring systems that track the effectiveness of fraud prevention measures and provide insights for constant improvement. Automatically monitor key performance indicators (KPIs) and metrics to identify changes in fraud patterns, detect new threats, and adapt fraud prevention strategies accordingly.
By leveraging collaborative approaches and automation, organizations can enhance the effectiveness and efficiency of their fraud prevention initiatives. These approaches promote information sharing, collective intelligence, and streamlined processes, enabling faster detection, response, and adaptation to emerging fraud threats.
FAQ's
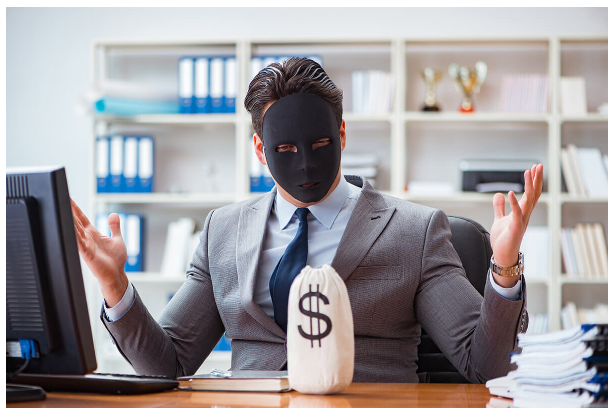
How does big data analytics contribute to fraud prevention?
Big data analytics is pivotal in fraud prevention, offering several significant contributions to detecting and mitigating fraudulent activities. Here’s how big data analytics contributes to fraud prevention:
- Data-driven Insights: Big data analytics leverages vast amounts of data, including transactional records, customer information, logs, and external feeds. By analyzing this data, patterns, trends, and anomalies can be identified, providing valuable insights into fraudulent activities. These insights enable organizations to make informed decisions and take proactive measures to prevent fraud.
- Anomaly Detection: Big data analytics techniques, such as statistical analysis and machine learning algorithms, effectively detect anomalies in large datasets. By establishing baseline patterns of normal behavior, deviations or abnormalities that may indicate fraud can be identified. For example, unusual transaction amounts, atypical customer behaviors, or suspicious network connections can be flagged for investigation.
- Predictive Modeling: Big data analytics allows organizations to build predictive models based on historical data. These models can forecast the likelihood of future fraudulent events. By identifying patterns, correlations, and risk factors associated with fraud, predictive models help prioritize investigations, allocate resources effectively, and take preventive measures to mitigate potential fraud risks.
- Real-time Monitoring: Big data analytics enables real-time monitoring of transactions and activities. By applying advanced analytics techniques and automated systems, organizations can detect and respond to suspicious events in real-time. This timely monitoring allows immediate intervention to prevent or minimize losses due to fraud.
- Link Analysis and Network Detection: Fraudsters often operate in networks or conspire with others. Big data analytics facilitates link and social network analysis, allowing organizations to uncover hidden connections and relationships among entities. By mapping these networks, organizations can identify clusters, associations, or patterns that indicate fraudulent activities, aiding in detecting and disrupting fraudulent operations.
- Text Mining and Natural Language Processing: Unstructured data, such as emails, chat logs, or social media posts, can contain valuable insights for fraud prevention. Big data analytics employs text mining and natural language processing techniques to extract relevant information and identify fraud-related keywords or sentiments. This helps in uncovering potential fraud indicators or detecting fraud-related conversations.
- Continuous Improvement: Big data analytics enables continuous improvement in fraud prevention efforts. By regularly analyzing data, evaluating the performance of models, and incorporating new data, organizations can refine their strategies, detect emerging fraud trends, and adapt to evolving fraud techniques. Continuous improvement ensures that fraud prevention measures stay practical and up-to-date.
Big data analytics significantly contributes to fraud prevention by providing data-driven insights, enabling anomaly detection, facilitating predictive modeling, supporting real-time monitoring, uncovering network connections, utilizing text mining, and promoting continuous improvement. These contributions empower organizations to proactively detect, prevent, and mitigate fraudulent activities, protecting their financial assets, reputation, and customer trust.