Mastering Accurate Revenue Forecasting Strategies for Business Success
Accurate revenue forecasting is a vital component for achieving business success. Companies can make informed decisions, optimize resource allocation, and drive growth by mastering effective strategies in this area.Â
- One key approach is to analyze historical data to identify trends and patterns. For instance, examining revenue fluctuations over different periods can help uncover seasonal trends or identify specific factors influencing sales.
- Another strategy is to leverage market research and industry data. Businesses can make more accurate revenue projections by understanding market dynamics, customer preferences, and competitor behavior. This can involve analyzing market size, growth rates, and market share data. Additionally, staying updated on industry trends and consumer insights can provide valuable information for forecasting revenue.
- Furthermore, incorporating predictive analytics techniques can enhance forecasting accuracy. Leveraging advanced algorithms, such as regression analysis or time series forecasting, businesses can make data-driven predictions based on historical performance, market conditions, and other relevant variables.
- It is also essential to regularly monitor and adjust forecasts based on real-time data. By comparing actual results with projected figures, companies can identify any discrepancies and make necessary adjustments to their strategies. This iterative approach ensures that revenue forecasts remain aligned with market realities.
Mastering accurate revenue forecasting strategies involves analyzing historical data, leveraging market research, incorporating predictive analytics, and continuously monitoring and adjusting forecasts. By adopting these practices, businesses can make informed decisions, allocate resources effectively, and drive sustainable growth.
Understanding Revenue Forecasting
Revenue forecasting is a critical aspect of business planning and decision-making. It involves predicting future revenue streams based on various factors and data sources. Understanding revenue forecasting is essential for effective resource allocation, budgeting, and overall business strategy.
To begin with, historical data analysis plays a crucial role in revenue forecasting. By examining past revenue patterns, trends, and seasonality, businesses can identify growth drivers and factors that impact revenue fluctuations. This historical perspective provides a foundation for predicting future revenue.
Market research is another critical component. Businesses can gain insights into market conditions, customer preferences, and competitive dynamics by gathering market data, including industry reports, customer surveys, and competitor analysis. These insights enable more accurate revenue projections and help identify opportunities and challenges.
Furthermore, internal factors like marketing campaigns, product launches, pricing strategies, and sales pipelines should be considered. By aligning these factors with market conditions, businesses can estimate the potential impact on revenue.
Technology and data analytics also play a significant role in revenue forecasting. Leveraging advanced tools and techniques like predictive analytics, machine learning, and statistical modeling, businesses can analyze large volumes of data to identify patterns and predict future outcomes. This data-driven approach enhances forecasting accuracy and helps companies to make informed decisions.
Regular monitoring and updating of revenue forecasts are essential. By comparing actual results with projected figures, businesses can evaluate the accuracy of their projections and make adjustments as needed. This iterative process ensures that revenue forecasts remain up-to-date and aligned with changing market dynamics.
Understanding revenue forecasting involves analyzing historical data, conducting market research, considering internal factors, leveraging technology and data analytics, and continuously monitoring and updating forecasts. By employing these practices, businesses can make more accurate predictions, mitigate risks, and optimize their strategies for sustainable revenue growth.
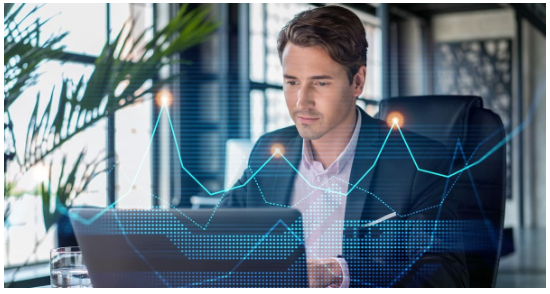
Define revenue forecasting and its significance in business planning and financial management.
Revenue forecasting refers to estimating and predicting the future revenue a business is expected to generate over a specific period. It involves analyzing historical data, market trends, industry insights, and internal factors to make informed projections about future income.
The significance of revenue forecasting in business planning and financial management cannot be overstated. Here are some key reasons why it is crucial:
- Planning and budgeting: Accurate revenue forecasts are the foundation for business planning and budgeting processes. They help set realistic financial goals, allocate resources effectively, and determine operational and investment priorities. By understanding expected revenue streams, businesses can align their expenditures and growth strategies accordingly.
- Resource allocation: Revenue forecasts aid in efficient resource allocation. Businesses can make informed decisions regarding staffing levels, inventory management, production capacity, marketing budgets, and other critical resources by estimating future revenue. This helps optimize resource utilization, minimize wastage, and ensure smooth operations.
- Financial management: Revenue forecasts play a vital role in financial management. They assist in cash flow planning, enabling businesses to anticipate high or low revenue periods and take appropriate measures to maintain adequate liquidity. Accurate revenue projections also contribute to effective financial reporting, financial statement analysis, and evaluating overall business performance.
- Risk management: Revenue forecasts help identify potential risks and uncertainties. Businesses can anticipate revenue fluctuations and mitigate associated risks by considering market volatility, changing customer behavior, and competitive landscape. This enables proactive risk management and helps businesses adapt to market dynamics.
- Decision-making: Reliable revenue forecasts provide critical information for decision-making. They guide strategic choices like product development, market expansion, pricing strategies, and investment decisions. With accurate revenue projections, businesses can evaluate various initiatives’ financial feasibility and profitability, enabling informed and confident decision-making.
- Performance evaluation: Revenue forecasts serve as benchmarks for evaluating business performance. Businesses can assess their accuracy by comparing actual revenue against projected figures, identifying gaps, and making necessary adjustments. This feedback loop facilitates continuous improvement and ensures business strategies align with financial goals.
Revenue forecasting is a fundamental aspect of business planning and financial management. It enables effective resource allocation, supports decision-making, assists in risk management, and serves as a basis for evaluating business performance. By conducting accurate and insightful revenue forecasts, businesses can navigate market uncertainties, achieve financial objectives, and drive sustainable growth.
Discuss the key components and factors involved in revenue forecasting
Revenue forecasting involves considering various components and factors to make accurate projections. Here are the key elements to consider when conducting revenue forecasting:
- Historical data: Analyzing revenue data is essential for identifying trends, patterns, and seasonality. It helps to understand past performance and establish a baseline for future projections. Historical data can reveal growth rates, cyclical fluctuations, and the impact of external factors on revenue.
- Market research: Conducting comprehensive market research provides insights into market dynamics, customer behavior, and competitive landscape. It involves studying industry reports, customer surveys, market size, and growth trends. Market research helps identify potential opportunities, threats, and market-specific factors that may impact revenue.
- Sales pipelines and leads: Evaluating the sales pipelines and leaders is crucial for revenue forecasting. Understanding the number of charges, conversion rates, and average deal values helps estimate potential revenue from new and existing customers. Analyzing the sales funnel and customer acquisition process provides visibility into future revenue streams.
- Internal factors: Internal factors that impact revenue forecasting include marketing strategies, pricing decisions, product launches, and sales initiatives. These factors directly influence customer demand and revenue generation. Evaluating the impact of internal initiatives on revenue is essential for accurate forecasting.
- External factors: External factors such as economic conditions, industry trends, regulatory changes, and technological advancements can significantly impact revenue. Considering macroeconomic indicators, industry-specific developments, and potential disruptions helps forecast revenue more accurately.
- Customer behavior: Understanding customer preferences, buying patterns, and shifts in demand is crucial for revenue forecasting. Changing consumer trends, demographic shifts, and evolving market needs can impact revenue generation. Analyzing customer behavior and incorporating it into forecasting models improves accuracy.
- Competitor analysis: Assessing the competitive landscape and monitoring competitors’ activities helps anticipate market dynamics and potential impacts on revenue. Understanding competitors’ pricing strategies, market share, and product positioning assists in forecasting market demand and estimating competitive pressures.
- Seasonality and trends: Identifying seasonal patterns and trends specific to the industry or business is critical for revenue forecasting. Many companies experience fluctuations in revenue due to factors like holidays, weather conditions, or industry-specific cycles. Accounting for seasonality and trends improves the accuracy of revenue projections.
- Data analytics and modeling: Leveraging advanced data analytics techniques and statistical models enhances revenue forecasting accuracy. Predictive analytics, regression analysis, time series forecasting, and machine learning algorithms can be applied to analyze historical data and make data-driven projections.
- Continuous monitoring and adjustment: Revenue forecasting is an iterative process that requires constant monitoring and adjustment. Regularly comparing actual revenue against projected figures helps evaluate the accuracy of forecasts and identify any discrepancies. This enables making necessary adjustments to improve future predictions.
Businesses can make more informed projections and enhance their decision-making processes by considering these critical components and factors in revenue forecasting.
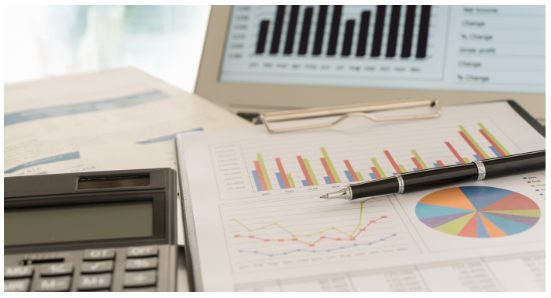
Introduce different methods and approaches for revenue forecasting, such as historical data analysis, market trends assessment, and predictive modeling
Regarding revenue forecasting, several methods and approaches can be utilized to improve accuracy and reliability. Here are three commonly used methods:
- Historical Data Analysis: This method examines past revenue performance to identify patterns, trends, and seasonality. By analyzing historical data, businesses can understand how revenue has changed, recognize recurring patterns, and make predictions based on past performance. Historical data analysis provides a valuable foundation for forecasting, especially when historical patterns are expected to continue.
- Market Trends Assessment: Analyzing market trends involves analyzing external factors and market dynamics influencing revenue. This approach incorporates market research, industry reports, customer insights, and competitor analysis to understand market conditions and behavior. By studying market trends, businesses can anticipate shifts in demand, changes in consumer preferences, and competitive factors that may impact revenue. This information helps to make informed projections and adjust strategies accordingly.
- Predictive Modeling: Predictive modeling involves leveraging statistical techniques, data analytics, and machine learning algorithms to forecast revenue. It uses historical data, market variables, and other relevant factors to build models predicting future revenue outcomes. Regression analysis, time series forecasting, and machine learning algorithms can be applied to analyze patterns, identify relationships, and make data-driven predictions. Predictive modeling provides a more sophisticated and data-driven approach to revenue forecasting, considering multiple variables and their interdependencies.
Each of these methods has its strengths and considerations. Historical data analysis is valuable when historical patterns are expected to repeat, but it may not account for dynamic market changes. Market trends assessment helps capture external factors and market dynamics, but it relies on current market conditions and may need to be more accurate for long-term forecasting. Predictive modeling offers a more comprehensive and analytical approach, incorporating multiple variables and relationships, but it requires sufficient data and expertise in modeling techniques.
In practice, combining these methods may yield the most reliable revenue forecasts. By considering historical data and market trends and employing predictive modeling techniques, businesses can gain a holistic view of revenue projections, minimize risks, and make informed decisions to drive business success.
Best Practices for Accurate Revenue Forecasting
Accurate revenue forecasting is crucial for effective business planning and decision-making. To ensure the reliability of revenue forecasts, here are some best practices to follow:
- Gather and analyze high-quality data: Collect comprehensive and reliable data from multiple sources, including historical sales data, customer data, market research, and industry reports. Ensure the data is accurate, consistent, and relevant to your forecasting objectives. Clean and preprocess the data to eliminate any inconsistencies or outliers that could skew the forecast results.
- Use multiple forecasting methods: Relying on a single forecasting method can limit the accuracy of your predictions. Instead, employ a combination of historical data analysis, market trend assessment, and predictive modeling methods. Each technique brings unique insights and can help validate and cross-validate the forecasted figures.
- Segment your data: Analyze your revenue data based on different segments, such as customer segments, product categories, geographical regions, or sales channels. This allows for more granular analysis and helps capture variations and trends specific to each piece. By understanding the dynamics within each segment, you can improve the accuracy of your forecasts.
- Incorporate feedback loops: Regularly compare actual revenue figures with your forecasted numbers. Monitor and evaluate the accuracy of your forecasts and identify any discrepancies. Use this feedback to refine your forecasting models, adjust assumptions, and improve future projections. Incorporating feedback loops creates a continuous improvement cycle and enhances the accuracy of your predictions over time.
- Consider external factors: Revenue forecasts should account for external factors that can impact your business, such as changes in the economy, industry trends, regulatory developments, or competitive activities. Stay informed about these external factors through market research, industry analysis, and monitoring news and trends. Incorporate their potential impact into your forecasting models.
- Involve cross-functional collaboration: Revenue forecasting should not be limited to a single department. Involve key stakeholders from different functional areas, including sales, marketing, finance, and operations. Collaborative input can provide diverse perspectives and valuable insights and ensure the forecasts align with overall business strategies.
- Regularly review and update forecasts: Revenue forecasts should not be a one-time exercise. Continuously review and update your forecasts as new data becomes available or market conditions change. Regularly reassess assumptions, monitor emerging trends, and refine your models to maintain accuracy.
- Document assumptions and uncertainties: Document the assumptions made during the forecasting process and highlight any uncertainties or risks associated with the forecasts. This helps in understanding the limitations and potential deviations from the projected figures. It also lets you communicate your estimates’ reliability and confidence level to stakeholders.
By following these best practices, businesses can improve the accuracy of their revenue forecasts, make more informed decisions, and drive sustainable growth.
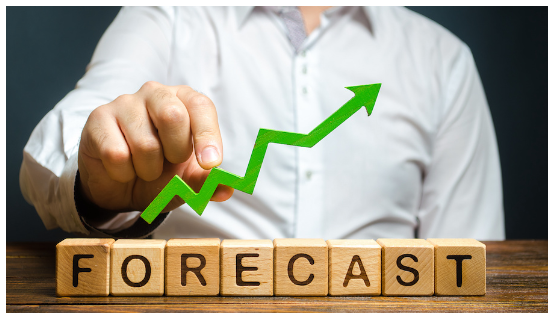
Identify and explain the essential steps for creating a robust revenue forecasting strategy.
Creating a robust revenue forecasting strategy involves several essential steps. Here are the key steps to follow:
- Define the Forecasting Period: Determine the timeframe for your revenue forecast, whether monthly, quarterly, or annually. The forecasting period should align with your business objectives and the accuracy required for decision-making.
- Establish Clear Objectives: Define the purpose and objectives of your revenue forecasting strategy. Are you aiming to support budgeting, resource allocation, strategic planning, or investor communications? Clear goals help guide the forecasting process and ensure the forecast aligns with the intended use.
- Gather Relevant Data: Collect comprehensive and relevant data from various sources. This includes historical sales data, customer data, market research, industry reports, and other data points impacting revenue. Ensure the data is accurate, complete, and representative of the business’s performance.
- Clean and Validate Data: Cleanse the data to eliminate any errors, outliers, or inconsistencies that could distort the forecast. Validate the data for accuracy and completeness, and address any missing or incomplete data points. Data cleansing and validation are crucial for generating reliable forecasts.
- Select Forecasting Methods: Choose the appropriate forecasting methods based on the nature of your business, available data, and objectives. Common methods include time series analysis, regression analysis, market trend assessment, and predictive modeling. Consider using various methods to capture different aspects and validate the forecast.
- Build Forecasting Models: Develop forecasting models that incorporate the selected methods. This involves using statistical software, spreadsheets, or specialized forecasting tools. Structure your models to account for historical data, market trends, seasonality, and specific drivers influencing revenue.
- Validate and Refine Models: Validate the forecasting models by comparing their output with actual historical data. Assess the accuracy and reliability of the forecasts and identify any gaps or discrepancies. Refine the models by adjusting assumptions, incorporating feedback, and iterating the process to improve accuracy.
- Involve Cross-Functional Collaboration: Collaborate with key stakeholders from various departments, such as sales, marketing, finance, and operations. Seek their input, insights, and expertise to ensure the forecast reflects a comprehensive understanding of the business dynamics.
- Monitor and Update: Regularly monitor the actual revenue performance against the forecasted figures. Continuously update and refine the forecast as new data becomes available or market conditions change. Monitor emerging trends, market dynamics, and internal factors impacting revenue.
- Document and Communicate: Document the forecasting process, assumptions made, and uncertainties associated with the forecast. Communicate the forecasted figures, limitations, and confidence level to stakeholders. This promotes transparency, helps manage expectations, and facilitates effective decision-making.
By following these essential steps, businesses can establish a robust revenue forecasting strategy that provides reliable insights for planning, resource allocation, and overall business management.
Discuss the importance of data quality and collection in accurate revenue forecasting
Data quality and collection are paramount for accurate revenue forecasting. Here’s an explanation of their importance:
- Reliable Insights: Accurate revenue forecasting relies on reliable and high-quality data availability. Poor data quality, such as missing values, inaccuracies, or inconsistencies, can lead to flawed forecasts and unreliable insights. By ensuring data quality, businesses can generate reliable forecasts that support informed decision-making.
- Historical Performance Analysis: Historical data serves as the foundation for revenue forecasting. It enables the identification of patterns, trends, and seasonality that impact revenue. If the historical data is incomplete, inaccurate, or inconsistent, it can hinder the ability to analyze past performance effectively and extract meaningful insights. Accurate data collection ensures a comprehensive view of historical revenue, enabling better forecasting accuracy.
- Validating Forecasting Models: Data quality plays a crucial role in validating the accuracy of forecasting models. When comparing actual revenue against forecasted figures, businesses need reliable data to assess the performance of their models. Suppose the data used for validation could be of better quality. In that case, it can lead to false conclusions about the effectiveness of the forecasting models, making it difficult to refine and improve future forecasts.
- Identifying Influencing Factors: Accurate revenue forecasting requires considering various influencing factors, such as market trends, customer behavior, and external economic factors. Collecting high-quality data allows businesses to identify and incorporate these factors into the forecasting models. With reliable data, assessing the impact of different variables becomes easier, leading to more accurate forecasts.
- Adjusting for Seasonality and Trends: Revenue forecasts often need to account for seasonality and trends in the business or industry. Collecting accurate data helps identify recurring patterns, cyclical trends, and the impact of seasonal variations on revenue. This allows companies to adjust their forecasts accordingly and make more accurate predictions for different periods.
- Cross-Validation and Verification: Data quality is crucial for cross-validating and verifying forecasting models. Businesses can use different data sets, periods, or segmentation to assess the accuracy and reliability of their forecasts. Reliable data collection ensures that cross-validation provides meaningful insights and strengthens confidence in the forecasted figures.
- Building Trust and Confidence: Accurate revenue forecasts are vital for gaining the trust and confidence of stakeholders, including investors, lenders, and management. High-quality data collection and analysis demonstrate a commitment to rigorous forecasting practices, enhancing credibility and fostering trust in the accuracy of the forecasts.
Data quality and collection are foundational elements for accurate revenue forecasting. They enable reliable historical analysis, model validation, identifying influencing factors, adjusting seasonality and trends, cross-validation, and building stakeholder trust. Businesses can significantly enhance the accuracy and reliability of their revenue forecasts by prioritizing data quality and adopting robust data collection processes.
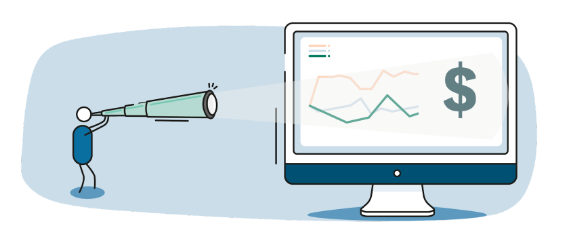
Explore techniques for analyzing historical data, market conditions, and customer behavior to improve forecasting accuracy.
Analyzing historical data, market conditions, and customer behavior is crucial for improving forecasting accuracy. Here are techniques to consider for each aspect:
Historical Data Analysis:
- Trend Analysis: Identify long-term trends and patterns in historical revenue data. Use moving averages or regression analysis to smooth out short-term fluctuations and identify underlying trends.
- Seasonality Analysis: Identify seasonal patterns and cycles in revenue data. Use seasonal decomposition or Fourier analysis to separate seasonal components from overall trends and understand the recurring patterns.
- Correlation Analysis: Examine the relationships between revenue and other relevant variables, such as marketing spend, pricing changes, or economic indicators. Conduct correlation analysis to identify variables that significantly impact revenue and use them as predictors in forecasting models.
Market Conditions Analysis:
- Market Research: Conduct thorough market research to understand industry dynamics, market size, growth rates, and competitive landscape. Analyze reports, industry publications, and market surveys to gain insights into market trends, customer preferences, and emerging opportunities or threats.
- Industry Benchmarks: Compare your revenue performance against industry benchmarks to evaluate your business’s relative performance. This analysis can help identify gaps and opportunities for improvement.
- Economic Indicators: Monitor relevant economic indicators such as GDP growth, consumer confidence, or interest rates. Understand how these indicators impact your target market and adjust revenue forecasts accordingly.
Customer Behavior Analysis:
- Customer Segmentation: Segment your customer base based on demographics, purchasing behavior, or other relevant factors. Analyze historical data within each segment to identify trends, preferences, and buying patterns specific to different customer groups.
- Cohort Analysis: Group customers based on similar characteristics or purchase periods to analyze their behavior over time. Understand customer retention rates, lifetime value, and revenue contributions by different cohorts. This analysis helps in identifying trends and predicting future revenue from existing customers.
- Customer Surveys and Feedback: Collect customer feedback through surveys, focus groups, or social media monitoring. Analyze customer sentiments, preferences, and feedback to gain insights into changing customer needs, emerging trends, and potential impacts on revenue.
It’s essential to leverage advanced analytical techniques and tools such as regression analysis, time series forecasting, and machine learning algorithms to enhance forecasting accuracy. These techniques can help uncover complex relationships, capture nonlinearities, and make data-driven predictions based on historical data, market conditions, and customer behavior.
Remember to continuously update and refine your forecasting models based on new data, market changes, and customer insights. This iterative approach ensures that your forecasts remain accurate and aligned with evolving business dynamics.
Highlight the role of collaboration between sales, marketing, finance, and other relevant teams in refining revenue forecasting.
Effective collaboration between sales, marketing, finance, and other relevant teams is vital in refining revenue forecasting. These teams can leverage their unique expertise to gather and analyze data by working together, leading to more accurate and reliable revenue projections.
Sales teams provide crucial insights into customer behavior, market trends, and emerging opportunities. Their on-the-ground experience helps identify potential revenue sources and gauge customer demand. Marketing teams, on the other hand, contribute by assessing campaign effectiveness, lead generation, and customer acquisition costs. Their data-driven approach enables them to identify key marketing channels and optimize strategies for revenue growth.
Finance teams bring their financial insight, analyzing historical data, financial statements, and market trends. They provide a comprehensive view of revenue patterns, profitability, and financial health, ensuring the accuracy and reliability of forecasts. By collaborating with these teams, finance can incorporate critical insights into their forecasting models, reducing uncertainties and improving accuracy.
Additionally, cross-functional collaboration fosters a culture of knowledge sharing and alignment. Regular meetings, data exchanges, and collaborative discussions allow teams to understand each other’s perspectives, challenges, and goals. Organizations can refine revenue forecasting models by leveraging collective expertise, identifying potential risks or opportunities, and making data-driven decisions to drive growth.
Collaboration between sales, marketing, finance, and other relevant teams enhances revenue forecasting by leveraging diverse insights, data analysis, and aligning organizational goals. This collaborative approach leads to more accurate and reliable revenue projections, enabling organizations to make informed strategic decisions.
FAQ's
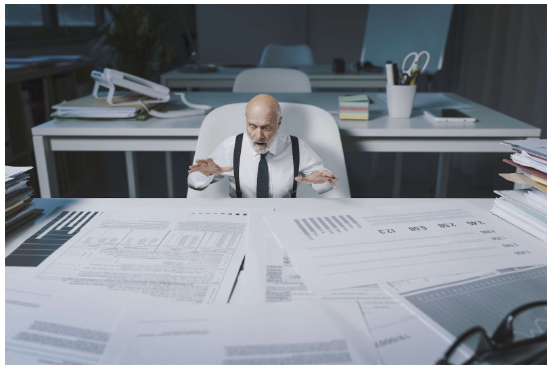
What are some common challenges in revenue forecasting, and how can they be overcome?
Revenue forecasting presents various challenges that organizations often face. By identifying and addressing these challenges, organizations can enhance the accuracy and reliability of their forecasts. Here are some common challenges and potential solutions:
- Lack of Data: More data is needed to ensure accurate revenue forecasting. Overcome this challenge by implementing data collection mechanisms like CRM systems to capture comprehensive customer and sales data. Explore external data sources, market research, and industry reports to supplement internal data.
- Seasonality and Market Volatility: Seasonal fluctuations and volatility can make revenue forecasting complex. Historical data analysis, trend identification, and seasonality adjustments can help mitigate these challenges. Consider utilizing statistical techniques like time series analysis or moving averages to account for patterns and fluctuations.
- Changing Market Dynamics: Rapidly evolving market conditions can make revenue forecasting challenging. Stay updated with industry trends, market research, and competitor analysis to gain insights into changing customer preferences and market dynamics. Regularly review and adjust forecasting models to reflect the latest market conditions.
- Lack of Collaboration and Alignment: Siloed departments and a lack of collaboration can lead to complete or accurate revenue forecasts. Foster cross-functional collaboration by facilitating regular meetings, data sharing, and joint forecasting sessions. Encourage open communication and align goals between sales, marketing, finance, and other relevant teams.
- Inaccurate Assumptions: Overly optimistic or pessimistic assumptions can lead to erroneous revenue forecasts. Validate assumptions with data and market research. Involve key stakeholders in forecasting to gain diverse perspectives and challenge assumptions. Regularly review and update beliefs as new information becomes available.
- Insufficient Forecasting Techniques: Using simplistic forecasting techniques may result in inaccurate predictions. Explore advanced forecasting models like regression analysis, predictive analytics, or machine learning algorithms to improve accuracy. Consider using qualitative and quantitative forecasting techniques to capture a holistic view.
- Lack of Continuous Monitoring and Evaluation: Revenue forecasting is an ongoing process that requires regular monitoring and evaluation. Establish performance metrics and KPIs to track forecast accuracy. Conduct regular reviews to identify gaps, adjust forecasts, and improve forecasting methodologies based on past performance.
By addressing these common challenges, organizations can enhance the accuracy and reliability of their revenue forecasting. A proactive, data-driven approach, collaboration, and continuous improvement will contribute to more informed decision-making and better financial planning.