Data-Driven Success Unleashed: The Analytics Operating Model
In the era of data-driven decision-making, organizations are increasingly leveraging analytics to gain valuable insights and drive success. The Analytics Operating Model provides a structured framework for effectively harnessing organizational data analytics capabilities. It enables the seamless integration of people, processes, data, and technology to deliver actionable insights and foster a data-driven culture. Here, we explore the key components and benefits of the Analytics Operating Model.
Components of the Analytics Operating Model:
- Strategy and Governance: Establishing a clear analytics strategy aligned with the organization’s overall objectives is crucial. Define the goals, priorities, and desired outcomes of the analytics initiatives. Formulate a governance structure to oversee analytics activities, ensure accountability, and make informed decisions.
- Data Management: Data is the foundation of analytics. Implement robust data management practices that encompass data collection, integration, quality assurance, storage, and accessibility. Establish data governance mechanisms to ensure data consistency, security, and compliance.
- Analytics Capabilities: Develop a skilled analytics workforce equipped with the necessary knowledge and tools. This involves hiring and training data analysts, data scientists, and data engineers. Foster a culture of data literacy, promote cross-functional collaboration, and provide resources for continuous learning and skill development.
- Technology Infrastructure: Leverage appropriate technologies to support analytics initiatives. This may include data storage systems, data integration platforms, data visualization tools, and advanced analytics software. Ensure the infrastructure is scalable, flexible, and aligned with the organization’s needs.
- Analytics Processes and Methodologies: Define standardized processes and methodologies for executing analytics projects. This includes data exploration, modeling, analysis, and visualization techniques. Adopt agile or iterative approaches to enable faster iterations, experimentation, and adaptation.
- Insights Delivery and Communication: Establish mechanisms for delivering insights to key stakeholders promptly and promptly. This includes data visualization, dashboards, reports, and presentations that cater to different user needs. Promote effective communication channels to facilitate collaboration and knowledge sharing.
- Performance Measurement and Monitoring: Define metrics and key performance indicators (KPIs) to measure the effectiveness and impact of analytics initiatives. Regularly monitor and track progress against these metrics to evaluate the success of the analytics program. Use data-driven insights to drive continuous improvement and inform decision-making.
Benefits of the Analytics Operating Model:
- Data-Driven Decision-Making: By implementing the Analytics Operating Model, organizations can leverage data-driven insights to make informed decisions. This improves accuracy, efficiency, and effectiveness in strategic planning, operational management, and customer engagement.
- Enhanced Performance and Efficiency: The model enables organizations to identify and address inefficiencies, optimize processes, and uncover hidden growth opportunities. Organizations can drive performance improvement, cost reduction, and operational excellence by leveraging analytics capabilities.
- Competitive Advantage: Analytics-driven organizations gain a competitive edge by leveraging data to identify trends, patterns, and opportunities. They can make agile and proactive decisions based on insights derived from robust analytics capabilities.
- Improved Customer Experience: Analytics helps organizations understand customer behavior, preferences, and needs more accurately. By leveraging customer data, organizations can personalize experiences, deliver targeted marketing campaigns, and enhance customer satisfaction and loyalty.
- Innovation and Agility: The Analytics Operating Model fosters a culture of innovation and experimentation. It enables organizations to identify new business models, develop data-driven products and services, and adapt quickly to changing market dynamics.
The Analytics Operating Model serves as a roadmap for organizations to unleash the power of data and drive success. By integrating people, processes, data, and technology effectively, organizations can harness the transformative potential of analytics. By making informed, data-driven decisions, organizations can gain a competitive advantage, enhance performance, and propel themselves into the future of data-driven
Importance of an analytics operating model in driving data-driven success
An analytics operating model is crucial in driving data-driven success for organizations. Here are the key reasons why an analytics operating model is important:
- Alignment with Business Objectives: An analytics operating model ensures that data analytics initiatives are closely aligned with the overall business objectives of an organization. By defining the goals, priorities, and desired outcomes of analytics activities, organizations can focus on driving insights and actions that directly contribute to business success.
- Clear Governance and Accountability: The operating model establishes a governance structure that defines analytics initiatives’ roles, responsibilities, and decision-making processes. This ensures clear accountability and ownership of data analytics projects, enabling effective execution and coordination across teams and departments.
- Efficient Data Management: Data is the fuel for analytics. An analytics operating model helps organizations implement efficient data management practices, including data collection, integration, quality assurance, storage, and accessibility. This ensures that high-quality and reliable data is available for analysis, leading to more accurate insights and better decision-making.
- Skilled Workforce and Collaboration: Developing an analytics operating model involves building a skilled analytics workforce equipped with the necessary expertise and tools. It fosters a culture of data literacy and promotes cross-functional collaboration between business stakeholders and analytics teams. This enables organizations to leverage diverse perspectives and expertise to drive insights and actions.
- Scalable and Agile Analytics Processes: The operating model defines standardized analytics processes and methodologies. This ensures consistency and efficiency in executing analytics projects. It also encourages the adoption of agile or iterative approaches, allowing organizations to quickly adapt to changing business needs and incorporate feedback for continuous improvement.
- Insights Delivery and Communication: An analytics operating model establishes effective mechanisms for delivering insights to key stakeholders in a timely and actionable manner. This includes data visualization, dashboards, reports, and presentations tailored to the needs of different users. Effective communication channels foster collaboration, knowledge sharing, and data-driven decision-making.
- Performance Measurement and Monitoring: The operating model emphasizes measuring the impact and effectiveness of analytics initiatives through defined metrics and key performance indicators (KPIs). Regular monitoring and tracking of these metrics enable organizations to evaluate their analytics program’s success, identify improvement areas, and make data-driven decisions to drive continuous innovation.
Organizations can establish a structured framework that optimizes their data analytics capabilities by implementing an analytics operating model. This enables them to uncover valuable insights, make informed decisions, drive innovation, and gain a competitive advantage in the data-driven business landscape.
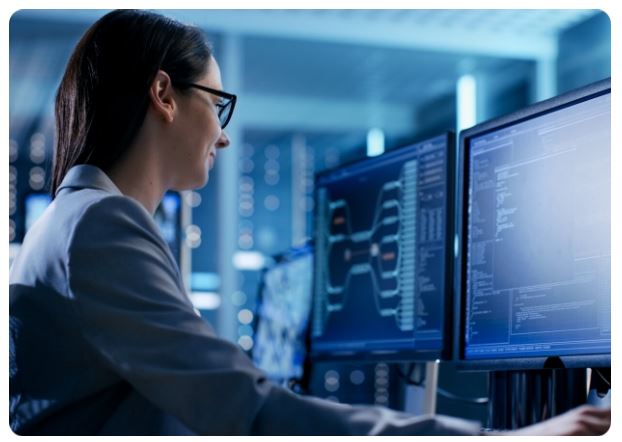
Understanding the Analytics Operating Model
The Analytics Operating Model is a framework that outlines the structure, processes, and capabilities required to harness data analytics within an organization effectively. It provides a roadmap for organizations to strategically leverage their data assets, build analytics capabilities, and drive data-driven decision-making. Here are the key components and concepts that help in understanding the Analytics Operating Model:
- Strategy and Governance: This component defines the overall analytics strategy, objectives, and priorities aligned with the organization’s goals. It also establishes a governance framework to ensure accountability, decision-making processes, and alignment with regulatory and compliance requirements.
- Data Management: This component involves the collection, integration, quality assurance, storage, and accessibility of data assets. It includes data governance practices, architecture, security, and compliance with data privacy regulations. Effective data management ensures that reliable and high-quality data is available for analytics initiatives.
- Analytics Capabilities: This component addresses the skills, resources, and tools required to perform analytics tasks. It involves building a team of data analysts, data scientists, and data engineers with the necessary expertise. Analytics capabilities include data exploration, modeling, analysis, visualization, and statistical techniques.
- Technology Infrastructure: This component focuses on the technological foundation required to support analytics initiatives. It involves selecting and implementing appropriate tools, platforms, and infrastructure to store, process, and analyze data effectively. This may include data warehouses, data lakes, cloud platforms, and advanced analytics software.
- Analytics Processes and Methodologies: This component defines the processes, methodologies, and workflows for executing analytics projects. It includes data preparation, modeling techniques, analysis methodologies, and the deployment of analytics insights. Agile or iterative approaches may be adopted to enable flexibility, experimentation, and continuous improvement.
- Insights Delivery and Communication: This component involves delivering analytics insights to stakeholders in a meaningful and actionable manner. It includes data visualization, dashboards, reports, and presentations catering to different users’ needs. Effective communication channels are established to foster collaboration, knowledge sharing, and data-driven decision-making.
- Performance Measurement and Monitoring: This component focuses on defining metrics, key performance indicators (KPIs), and monitoring processes to assess the effectiveness and impact of analytics initiatives. Regular monitoring and tracking enable organizations to evaluate their analytics program’s success, identify improvement areas, and drive continuous innovation.
The Analytics Operating Model provides a structured approach for organizations to build a data-driven culture, optimize analytics capabilities, and generate valuable insights. By leveraging this framework, organizations can unlock the full potential of their data assets and drive strategic decision-making that leads to improved performance, operational efficiency, and competitive advantage.
Definition and components of an analytics operating model
An analytics operating model refers to the framework and structure within an organization that enables effective and efficient data analytics processes and activities. It defines the key components, roles, responsibilities, and processes required to deliver analytics capabilities and drive data-driven decision-making.Â
The specific components of an analytics operating model may vary depending on the organization’s size, industry, and objectives, but they typically include the following:
- Strategy and Vision: This component outlines the organization’s overall analytics strategy and vision, aligning it with the business objectives. It defines the analytics function’s goals, priorities, and desired outcomes.
- Governance: Governance establishes the decision-making framework, policies, and guidelines for managing data and analytics initiatives. It includes data governance, ensuring data quality, privacy, compliance, and analytics governance, which encompasses guidelines for model development, validation, and deployment.
- Organization and Talent: This component focuses on the organizational structure and the required talent to support analytics initiatives. It includes defining roles and responsibilities, establishing cross-functional teams, and developing the necessary skill sets within the organization.
- Data Management: Data management involves processes and technologies to collect, store, integrate, and manage data across the organization. It encompasses data acquisition, quality management, integration, storage, and access controls.
- Analytics Capabilities: This component covers the tools, technologies, and data analysis and modeling methodologies. It includes data exploration, descriptive analytics, diagnostic analytics, predictive analytics, prescriptive analytics, and data visualization techniques.
- Processes and Workflow: Processes and workflow define the end-to-end lifecycle of analytics initiatives, from data collection to insights generation and decision-making. It involves defining data workflows, project management methodologies, collaboration mechanisms, and iterative feedback loops.
- Performance Measurement: Performance measurement establishes metrics and key performance indicators (KPIs) to evaluate the effectiveness and impact of analytics initiatives. It includes tracking and reporting on data quality, model accuracy, business outcomes, and return on investment (ROI).
- Change Management: Change management focuses on driving organizational adoption and cultural transformation to embrace data-driven decision-making. It involves communication, training, and stakeholder engagement to ensure buy-in and support for analytics initiatives.
By establishing a robust analytics operating model, organizations can create a structured and scalable approach to leverage data effectively, derive insights, and drive business value through analytics initiatives.
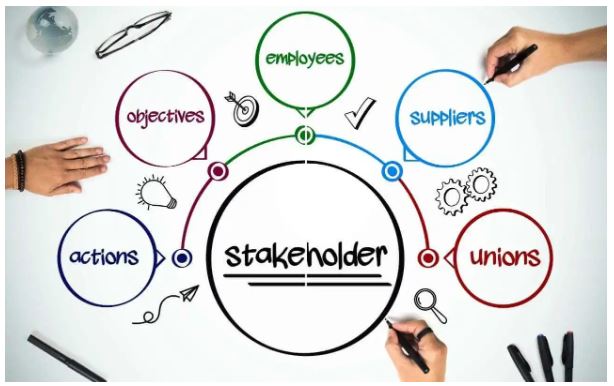
Roles and responsibilities of different stakeholders in the model
In an analytics operating model, several stakeholders are involved, each with their own roles and responsibilities. Here are the key stakeholders and their typical roles:
- Executive Leadership: The executive leadership team, including the C-suite and senior executives, is responsible for setting the strategic direction for analytics initiatives. They define the organization’s analytics vision, allocate resources, and ensure alignment between analytics goals and business objectives. They also provide support and advocacy for data-driven decision-making across the organization.
- Chief Data Officer (CDO): The CDO or the equivalent role oversees the organization’s data management strategy. They establish data governance policies, ensure data quality and privacy, and facilitate data integration across systems. The CDO also collaborates with business leaders to identify data-driven opportunities and support decision-making processes.
- Analytics Center of Excellence (CoE): The Analytics CoE is a dedicated team responsible for driving analytics initiatives and best practices within the organization. The CoE typically consists of data scientists, analysts, and other analytics professionals. Their responsibilities include developing and maintaining analytics capabilities, conducting advanced analytics projects, creating models, and providing insights and recommendations to business stakeholders.
- Business Units/Departments: Different business units and departments within the organization play a crucial role in leveraging analytics. They collaborate with the analytics team to define business objectives, identify data needs, and provide domain expertise. Business stakeholders articulate their requirements and priorities to the analytics team and use the insights generated to make data-driven decisions.
- IT Department: The IT department supports the technical infrastructure required for analytics initiatives. They provide data storage and management systems, ensure data security and access controls, and maintain the necessary hardware and software infrastructure. The IT team collaborates with the analytics team to ensure data integration, data quality, and seamless functioning of analytics tools and platforms.
- Data Stewards: Data stewards ensure data quality, integrity, and compliance within their respective domains. They work closely with the CDO and the data governance team to define data standards, establish data quality metrics, resolve data-related issues, and enforce data governance policies. Data stewards play a crucial role in maintaining the accuracy and reliability of data used for analytics.
- Data Consumers: Data consumers are individuals or teams utilizing analytics insights in their daily decision-making processes. This includes business managers, operational teams, marketing teams, finance teams, and other stakeholders. Data consumers rely on the analytics team to provide actionable insights and recommendations based on data analysis.
- Change Management Team: The change management team drives organizational adoption and cultural transformation toward data-driven decision-making. They facilitate communication and training initiatives to educate stakeholders about the benefits of analytics and help them embrace data-driven practices. The change management team also works with the analytics team to identify potential barriers to adoption and develop strategies to overcome them.
These stakeholders work collaboratively within the analytics operating model to ensure the effective implementation of analytics initiatives, from strategy development to data analysis and decision-making, ultimately driving value and competitive advantage through data-driven insights.
Key Principles and Processes for effective data-driven Decision-making
Effective data-driven decision-making involves following key principles and processes to ensure decisions are based on reliable, relevant, and actionable insights. Here are some key principles and processes for effective data-driven decision-making:
- Define Clear Objectives: Clearly define the objectives and goals of the decision-making process. This includes identifying the problem or opportunity to be addressed and understanding desired outcomes or results.
- Identify Relevant Data: Determine the data that is needed to address the objectives. This involves identifying the relevant data sources, both internal and external, and ensuring data quality and integrity. Consider the data’s relevance, accuracy, completeness, and timeliness.
- Data Collection and Integration: Collect and integrate the identified data from various sources. This may involve data extraction, transformation, and loading (ETL) processes to bring the data into a suitable format for analysis. Ensure data privacy and compliance during the data collection and integration process.
- Data Exploration and Analysis: To derive insights and patterns, explore and analyze the data. This can involve various techniques such as descriptive, diagnostic, predictive, and prescriptive analytics. Apply statistical methods, data mining techniques, and visualization tools to uncover patterns, trends, and relationships within the data.
- Interpretation and Insight Generation: Interpret the analyzed data to generate meaningful insights. Translate the findings into actionable insights that can inform decision-making. Consider the context, business objectives, and constraints when interpreting the data to ensure relevance and practicality.
- Collaborative Decision-Making: Foster collaboration among relevant stakeholders in the decision-making process. Involve individuals with domain expertise, data analysts, and decision-makers to collectively analyze the insights, discuss potential courses of action, and evaluate the potential outcomes.
- Risk Assessment and Mitigation: Evaluate the potential risks associated with different decision options. Consider uncertainties, assumptions, and potential unintended consequences. Assess the potential impact of each decision option and develop strategies to mitigate risks.
- Monitor and Evaluate: Implement the chosen decision and establish mechanisms to monitor its outcomes. Continuously evaluate the decision’s effectiveness and impact, and track the key performance indicators (KPIs) to measure success. This enables iterative learning and improvement in future decision-making processes.
- Communication and Visualization: Communicate the insights and decision outcomes effectively to stakeholders. Use data visualization techniques to present complex information in a clear and understandable manner. Tailor the communication to the target audience, ensure the information is actionable, and support decision-making.
- Continuous Learning and Adaptation: Foster a culture of continuous learning and adaptation based on data and feedback. Encourage an environment where insights from previous decisions inform future decisions and new data and information are continuously integrated to refine decision-making processes.
By adhering to these principles and processes, organizations can enhance their ability to make informed decisions based on data, leading to better outcomes, improved performance, and a competitive advantage in the marketplace.
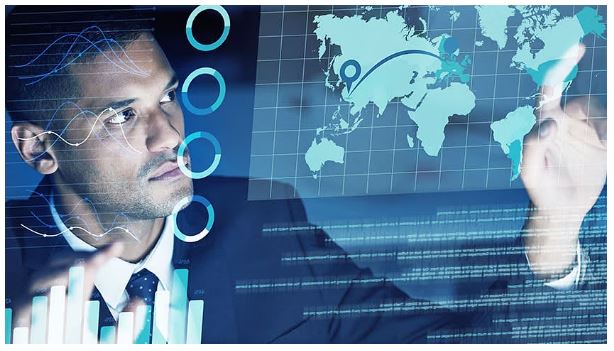
Implementing an Effective Analytics Operating Model
Implementing an effective analytics operating model requires careful planning, collaboration, and the following key steps:
- Define the Vision and Strategy: Clearly articulate the organization’s vision for analytics and align it with the overall business strategy. Define the desired outcomes, goals, and priorities for the analytics function.
- Assess Current State: Evaluate the organization’s existing analytics capabilities, infrastructure, and processes. Identify strengths, weaknesses, gaps, and areas for improvement. This assessment serves as a baseline for designing the future state.
- Design the Operating Model: Develop a robust operating model that aligns with the organization’s objectives. Determine the key components, such as governance, organization structure, data management, analytics capabilities, processes, and performance measurement.
- Establish Governance Framework: Define the governance framework to guide decision-making and ensure accountability. Establish data governance policies, standards, and processes to ensure data quality, privacy, and compliance. Implement analytics governance to oversee model development, validation, and deployment.
- Organizational Structure and Talent: Design the organizational structure that supports the analytics operating model. Define roles and responsibilities, including the analytics team, data stewards, and business stakeholders. Identify the necessary skills and competencies required and develop a talent acquisition and development strategy.
- Data Management: Implement data management processes to ensure data quality, integration, and accessibility. Develop data acquisition and integration strategies, storage and retrieval mechanisms, and security measures. Implement data governance practices to ensure data integrity and compliance.
- Analytics Capabilities and Tools: Determine the required analytics capabilities and tools based on business needs. Identify the analytical techniques, software, and infrastructure required for data analysis and modeling. Develop a roadmap for building and enhancing analytics capabilities.
- Processes and Workflow: Design processes and workflows to support end-to-end analytics initiatives. Define data collection, analysis, and reporting processes. Establish project management methodologies, collaboration mechanisms, and iterative feedback loops. Ensure effective communication and collaboration across teams.
- Performance Measurement: Establish metrics and KPIs to measure the effectiveness and impact of analytics initiatives. Define the key performance indicators that align with the organization’s goals. Develop reporting mechanisms to track and communicate performance results.
- Change Management and Adoption: Implement change management strategies to ensure the successful adoption of the analytics operating model. Communicate the benefits of data-driven decision-making and provide training to enhance data literacy across the organization. Foster a culture that values and embraces analytics and supports continuous learning.
- Pilot and Iterate: Begin with pilot projects to validate and refine the analytics operating model. Learn from the pilot initiatives, gather feedback, and iterate on the model as needed. Use the insights gained to improve the analytics capabilities and processes continuously.
- Monitor and Evaluate: Establish a system for ongoing monitoring and evaluation of the analytics operating model. Continuously assess its effectiveness, identify areas for improvement, and make necessary adjustments. Monitor the outcomes and impact of analytics initiatives to ensure they align with the organization’s goals.
By following these steps and ensuring active involvement and stakeholder collaboration, organizations can implement an analytics operating model that enables data-driven decision-making and maximizes the value derived from data and analytics capabilities.
Assessing organizational readiness and identifying objectives
Assessing organizational readiness and identifying objectives is important before implementing data governance and quality assurance practices. Here are some steps to help you in this process:
- Evaluate Current Data Management Practices: Assess your organization’s existing data management processes, systems, and policies. Identify any gaps, inefficiencies, or challenges that may exist. This evaluation will help you understand the starting point and identify areas for improvement.
- Understand Organizational Goals and Strategy: Gain a clear understanding of your organization’s goals, strategic objectives, and priorities. Identify how data governance and quality assurance align with these goals and how they can contribute to achieving them. This understanding will help you set objectives that are in line with the overall organizational vision.
- Engage Stakeholders: Identify key organizational stakeholders who will be impacted by data governance and quality assurance initiatives. This can include executives, department heads, data owners, IT personnel, and compliance officers. Engage with these stakeholders to understand their perspectives, concerns, and expectations regarding data management.
- Conduct a Readiness Assessment: Evaluate the readiness of your organization to implement data governance and quality assurance practices. This assessment can include evaluating organizational culture, leadership support, resource availability, technological infrastructure, and data maturity. Identify any potential barriers or challenges that need to be addressed.
- Define Objectives and Priorities: Based on the assessment conducted, define clear objectives and priorities for your data governance and quality assurance initiatives. These objectives should be specific, measurable, achievable, relevant, and time-bound (SMART). Examples of objectives could be improving data accuracy, ensuring regulatory compliance, enhancing data security, or optimizing data processes.
- Set Implementation Roadmap: Develop a roadmap that outlines the step-by-step implementation plan for data governance and quality assurance initiatives. This roadmap should include timelines, milestones, resource requirements, and dependencies. It should also consider prioritization based on the identified objectives and alignment with the organization’s strategic goals.
- Secure Leadership Support: Gain support from organizational leadership, including executives and senior management. Clearly communicate the benefits of data governance and quality assurance practices and how they contribute to achieving the organization’s goals. Secure necessary resources and budget to support the implementation efforts.
- Communicate and Create Awareness: Develop a communication plan to create awareness among employees about the importance of data governance and quality assurance. Clearly communicate the objectives, benefits, and expected outcomes of these initiatives. Foster a culture of data accountability and ownership throughout the organization.
- Establish Performance Metrics: Define key performance indicators (KPIs) to measure the progress and success of your data governance and quality assurance initiatives. These metrics should align with the objectives and priorities set earlier. Regularly monitor and report on these metrics to track the effectiveness of the implemented practices.
- Review and Adapt: Regularly review and assess the progress of your data governance and quality assurance initiatives. Seek feedback from stakeholders and identify areas for improvement. Adapt your strategies and actions as needed to ensure ongoing alignment with organizational goals and evolving data management needs.
By assessing organizational readiness and setting clear objectives, you can establish a solid foundation for implementing data governance and quality assurance practices that support your organization’s overall success.
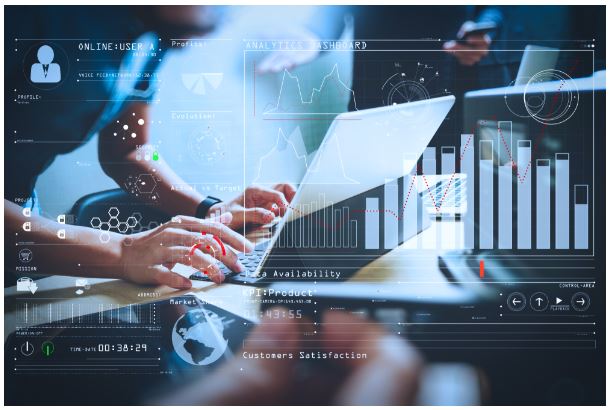
Building a data-driven culture and fostering cross-functional collaboration
Building a data-driven culture and fostering cross-functional collaboration is essential for organizations to successfully leverage data and drive innovation. Here are some key steps to help achieve these goals:
- Leadership Commitment: Leadership plays a critical role in driving a data-driven culture. Executives and managers should demonstrate a commitment to data-driven decision-making and actively promote its importance throughout the organization. They should lead by example and prioritize data-driven initiatives.
- Establish Clear Objectives and Goals: Clearly communicate the objectives and goals of becoming a data-driven organization. Ensure employees understand how data can drive decision-making, improve performance, and achieve strategic outcomes. Align individual and team goals with the organization’s data-driven objectives.
- Promote Data Literacy: Foster a culture of data literacy by providing training and resources to help employees understand and interpret data. Offer workshops, seminars, and online data analysis, visualization, and interpretation courses. Encourage employees to develop their data skills and provide support for continuous learning.
- Create Data Champions: Identity individuals or teams within each department who are passionate about data and can serve as data champions. Empower them to drive data-driven initiatives, share best practices, and provide guidance to their colleagues. These champions can help bridge the gap between technical and non-technical teams.
- Cross-Functional Collaboration: Encourage collaboration between different teams and departments to leverage diverse perspectives and expertise. Foster an environment where employees from various functions can work together on data-driven projects and initiatives. This collaboration enhances the understanding and utilization of data across the organization.
- Shared Data Infrastructure: Implement a shared data infrastructure that enables easy access to data across different teams. This can include a centralized data repository or data platform where relevant data is stored, documented, and made available to authorized users. Ensure proper security and access controls to protect sensitive data.
- Communicate Data Success Stories: Highlight and celebrate success stories demonstrating data-driven decisions’ impact. Share examples of how data-driven insights have led to positive outcomes, improved processes, or innovative solutions. This helps create enthusiasm and reinforces the value of data-driven practices.
- Foster a Feedback Culture: Encourage open communication and feedback regarding data-driven initiatives. Create forums, such as regular team meetings or town halls, where employees can share their insights, challenges, and ideas related to data-driven decision-making. Actively listen to feedback and address concerns to foster a supportive environment.
- Recognize and Reward Data-driven Efforts: Recognize and reward individuals and teams that actively embrace and contribute to a data-driven culture. This can be in the form of incentives, bonuses, or public recognition. Acknowledge and celebrate data-driven achievements to motivate employees and reinforce desired behaviors.
- Continuously Improve: Building a data-driven culture is an ongoing process. Regularly assess and evaluate the effectiveness of data-driven practices and initiatives. Seek feedback from employees and make adjustments as needed. Stay updated with industry trends and best practices to improve data-driven capabilities continuously.
By fostering a data-driven culture and promoting cross-functional collaboration, organizations can unlock the full potential of their data. This leads to improved decision-making, innovation, and overall organizational performance.
Establishing data governance and quality assurance practices
Establishing data governance and quality assurance practices is essential for organizations to ensure their data’s reliability, accuracy, and consistency. Here are the key steps to help you establish these practices:
- Define Data Governance Framework: Develop a data governance framework that outlines the overall strategy, goals, and responsibilities related to data governance. This framework should define the roles and responsibilities of data owners, data stewards, and other relevant stakeholders. It should also establish processes for decision-making, issue resolution, and ongoing monitoring.
- Identify Data Owners and Stewards: Assign data owners who will have overall accountability for specific datasets or data domains. Data stewards should be designated to manage and maintain the quality and integrity of data within their respective areas. Data owners and stewards should collaborate to define data standards, policies, and procedures.
- Develop Data Standards and Policies: Establish data standards and policies that govern data management practices within the organization. These standards should cover aspects such as data quality requirements, data definitions, data classification, data privacy, data security, and data retention. Ensure that the standards and policies align with regulatory and compliance requirements.
- Implement Data Quality Assessment: Design and implement data quality assessment processes to evaluate the accuracy, completeness, consistency, and timeliness of data. This can involve data profiling, cleansing, validation, and monitoring techniques. Define data quality metrics and regularly measure and report on them.
- Establish Data Documentation and Metadata Management: Maintain comprehensive documentation of data assets, including metadata. Metadata should capture information about data sources, transformations, lineage, and dependencies. Implement metadata management practices to ensure data traceability, facilitate discovery, and support governance processes.
- Implement Data Lifecycle Management: Define and implement data lifecycle management practices encompassing data creation, storage, usage, archiving, and disposal. Establish policies and procedures for data retention, archiving, and disposal in compliance with legal, regulatory, and business requirements. Ensure that data is managed effectively throughout its lifecycle.
- Establish Data Access and Security Controls: Implement appropriate data access controls to ensure that data is accessed only by authorized individuals. Enforce role-based access controls, data encryption, data masking, and other security measures to protect data from unauthorized access, breaches, or misuse. Regularly review and update access privileges based on changing roles and responsibilities.
- Provide Data Governance Training and Awareness: Conduct training programs to educate employees about data governance principles, data quality best practices, and their roles and responsibilities in maintaining data integrity. Foster a culture of data governance and quality assurance by raising awareness about the importance of data management across the organization.
- Establish Data Governance Committee: Form a data governance committee or steering group comprising representatives from different business units and functions. This committee should oversee the implementation of data governance and quality assurance practices, resolve data-related issues, and provide guidance on data management initiatives.
- Continuously Monitor and Improve: Establish processes to continuously monitor and assess the effectiveness of data governance and quality assurance practices. Regularly review data quality reports, address identified issues, and take corrective actions. Keep the data governance framework and practices up to date by incorporating feedback, lessons learned, and industry best practices.
Remember that implementing data governance and quality assurance practices is an iterative process that requires ongoing commitment and collaboration across the organization. It involves people, processes, and technology working together to ensure the integrity and value of organizational data.
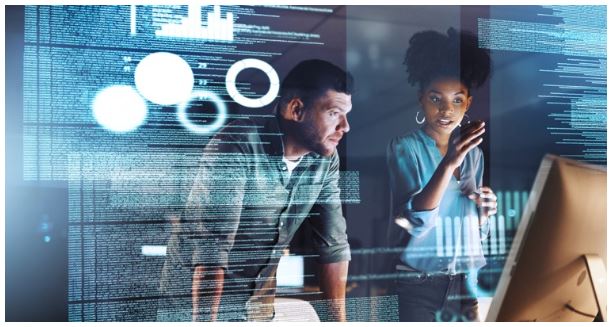
Driving Data-Driven Success with the Analytics Operating Model
Driving data-driven success with the analytics operating model involves establishing a framework that enables effective and efficient data analytics practices within an organization. Here are the key components and steps involved in implementing an analytics operating model:
- Define Objectives and Business Outcomes: Clearly define the objectives and desired business outcomes that your organization aims to achieve through data analytics. Align these objectives with the organization’s overall strategic goals to ensure a clear focus and direction.
- Establish Governance and Leadership: Develop a governance structure that defines roles, responsibilities, and decision-making processes for data analytics initiatives. This includes appointing an analytics leader or team responsible for driving the analytics strategy and ensuring alignment with organizational goals.
- Identify Data Needs and Sources: Identify the data types required to support the organization’s analytics goals. Determine the internal and external data sources available and establish data collection, integration, and storage processes. Ensure data accessibility and security.
- Build Data Infrastructure: Develop a robust infrastructure enabling efficient data storage, processing, and analysis. This may involve implementing data warehouses, data lakes, or cloud-based data platforms. Ensure scalability, reliability, and appropriate data governance mechanisms.
- Develop Analytical Capabilities: Build the necessary analytical capabilities within the organization. This includes hiring skilled data analysts, data scientists, and data engineers and providing training and development opportunities to existing staff. Foster a culture of data literacy and encourage data-driven decision-making across the organization.
- Implement Analytics Processes and Methodologies: Establish standardized processes and methodologies for data analytics projects. This includes defining data exploration, modeling, and visualization techniques. Adopt agile or iterative approaches to ensure quick iterations and continuous improvement.
- Enable Collaboration and Communication: Foster collaboration between business stakeholders and the analytics team. Encourage cross-functional collaboration to gain insights from different perspectives. Implement effective communication channels to share analytics findings and promote data-driven decision-making.
- Establish Performance Measurement and Monitoring: Define key performance indicators (KPIs) and metrics to measure the effectiveness and impact of data analytics initiatives. Regularly monitor and report on these metrics to track progress and identify areas for improvement. Use data visualization tools and dashboards to facilitate data-driven insights.
- Ensure Data Privacy and Ethics: Establish policies and practices to ensure data privacy, security, and compliance with applicable regulations. Adhere to ethical standards and guidelines in handling and analyzing data, especially when dealing with sensitive or personally identifiable information.
- Continuously Learn and Evolve: Embrace a continuous learning and improvement culture in data analytics. Stay updated with emerging technologies, industry trends, and best practices. Regularly review and refine the analytics operating model based on feedback, lessons learned, and evolving business needs.
By implementing an analytics operating model, organizations can harness the power of data to drive informed decision-making, optimize operations, and gain a competitive advantage in today’s data-driven business landscape.