Analytics is all about using data to make informed decisions. But what do you do when the data doesn’t give you a clear answer? What if you have a problem that seems unsolvable?
That’s where analytics problem solving techniques come in. By using certain methods and frameworks, you can break down complex problems and find effective solutions.
In this article, we’ll explore some of the most common analytics problem solving techniques, and give you practical tips on how to apply them to your own data challenges.
What is analytics problem-solving?
Analytics problem solving involves using data and statistical methods to identify, analyze, and solve complex business problems. It involves using various tools and techniques to collect, clean, and analyze data to gain insights and make informed decisions.
Analytics problem solving is used across different industries, such as healthcare, finance, retail, and marketing, to improve operational efficiency, reduce costs, increase revenue, and enhance customer experience.
To effectively solve problems using analytics, it is essential to clearly understand the problem statement, define key performance indicators (KPIs), and identify the relevant data sources.
This involves using various analytical models, such as regression, clustering, and decision trees, to analyze data and identify patterns and trends. By doing so, organizations can make data-driven decisions that result in improved outcomes and better business performance.
Importance of effective decision-making in analytics
Effective decision-making is critical in analytics because it allows organizations to make informed and data-driven decisions. Organizations generate large volumes of data from various sources in today’s fast-paced business environment. However, having data alone is insufficient; organizations need to effectively use that data to derive insights and make informed decisions.
Effective decision-making in analytics involves understanding the problem, defining the business objectives, and identifying the relevant data sources. It also requires analyzing and interpreting the data using statistical methods and tools. By doing so, organizations can identify patterns, trends, and insights to help them make informed decisions.
Making informed decisions using analytics can help organizations reduce costs, increase revenue, improve customer experience, and gain a competitive advantage. For instance, organizations can use analytics to optimize their marketing campaigns, improve supply chain management, or predict customer behavior.
By leveraging analytics for effective decision-making, organizations can transform data into a strategic asset that drives business growth and success.
The benefits of using analytics problem-solving techniques
Using analytics problem-solving techniques offers several benefits to organizations across different industries. Some of the key benefits of using analytics problem-solving techniques include:
- Improved decision-making: Analytics problem-solving techniques help organizations make data-driven decisions based on insights derived from data analysis. This improves the accuracy and reliability of decision-making.
- Increased efficiency and productivity: Analytics problem-solving techniques help organizations streamline processes and identify areas for optimization, resulting in improved efficiency and productivity.
- Cost savings: By identifying areas for optimization, organizations can reduce costs and improve profitability.
- Competitive advantage: Organizations that leverage analytics problem-solving techniques gain a competitive advantage by making informed decisions and identifying growth opportunities.
- Improved customer experience: By analyzing customer data, organizations can identify customer needs, preferences, and behavior patterns, resulting in improved customer experience
- Risk mitigation: Analytics problem-solving techniques can help organizations identify and mitigate risks, such as fraud or cybersecurity threats.
- Innovation: Analytics problem-solving techniques can help organizations identify new opportunities and areas for innovation, resulting in new products or services.
Using analytics problem-solving techniques offers several benefits to organizations, making it an essential tool for businesses looking to improve performance, profitability, and growth.
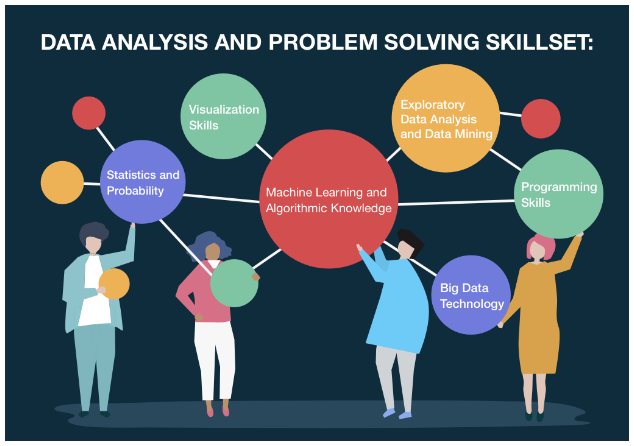
Define the Problem in analytics problem solving
Defining the problem is the first step in analytics problem-solving. It involves clearly understanding the business problem, identifying the root cause of the problem, and defining the problem statement. Defining the problem is essential for identifying the right data sources and analytics techniques.
To define the problem, it is important to ask questions that clarify the problem statement, such as “What is the problem?”, “Why is it a problem?” and “Who is affected by the problem?”. This helps ensure that all stakeholders clearly define and understand the problem.
Once the problem is defined, it is important to identify the business objectives and key performance indicators (KPIs) that will be used to measure the solution’s success. This involves identifying the desired outcomes and defining the metrics that will be used to track progress and evaluate the solution’s effectiveness.
Defining the problem is a crucial step in analytics problem-solving, laying the foundation for the rest of the process. By clearly defining the problem and identifying the business objectives, organizations can focus on the right data sources and analytics techniques to solve the problem and achieve the desired outcomes.
Techniques for defining the problem
Several techniques can be used to define the problem in analytics problem-solving. Some of these techniques include:
- Stakeholder analysis: This technique involves identifying all stakeholders who are affected by the problem and understanding their perspectives, needs, and priorities. By doing so, organizations can ensure that the problem is defined in a way that meets the needs of all stakeholders.
- Root cause analysis: This technique involves identifying the underlying causes of the problem by asking “why” questions. By identifying the root cause of the problem, organizations can develop solutions that address the underlying issues rather than just the symptoms.
- Problem statement templates: Using problem statement templates can help ensure that the problem is defined in a structured and concise manner. Templates can help organizations identify the key components of the problem, such as the issue, the impact, and the stakeholders affected.
- Brainstorming: Brainstorming involves generating ideas and solutions to the problem by encouraging open and creative thinking. This technique can help organizations identify new perspectives and potential solutions to the problem.
- Data analysis: Data analysis can help organizations identify patterns and trends contributing to the problem. By analyzing data, organizations can gain insights into the problem and identify potential solutions.
By using these techniques to define the problem, organizations can ensure that they clearly understand the problem statement, the business objectives, and the key performance indicators. This can help organizations develop effective solutions that address the underlying issues and achieve the desired outcomes.
Common mistakes to avoid when defining the problem
Defining the problem is a critical step in analytics problem-solving, and there are several common mistakes that organizations should avoid. Some of these mistakes include the following:
- Assuming the problem is well-defined: Organizations may think that the problem is well-defined and jump straight into data collection and analysis without fully understanding the problem statement. This can lead to accurate insights and effective solutions.
- Defining the problem too broadly or too narrowly: Defining the problem too broadly can make it difficult to identify the root cause while defining the problem too narrowly can result in solutions that only address the symptoms of the problem rather than the underlying issues.
- Focusing on the wrong problem: Organizations may focus on a symptom of the problem rather than the underlying issue, resulting in solutions that must address the root cause.
- Not involving stakeholders: Organizations may only affect some stakeholders in the problem definition process, resulting in solutions that do not meet the needs of all stakeholders.
- Not identifying the business objectives and KPIs: Organizations may need to identify the business objectives and KPIs that will be used to measure the solution’s success, making it difficult to evaluate the solution’s effectiveness.
- Using biased language: Organizations may use biased language when defining the problem, which can result in solutions that are not inclusive or equitable.
By avoiding these common mistakes and using effective problem definition techniques, organizations can ensure that they have a clear understanding of the problem statement, the business objectives, and the key performance indicators. This can help organizations develop effective solutions that address the underlying issues and achieve the desired outcomes.
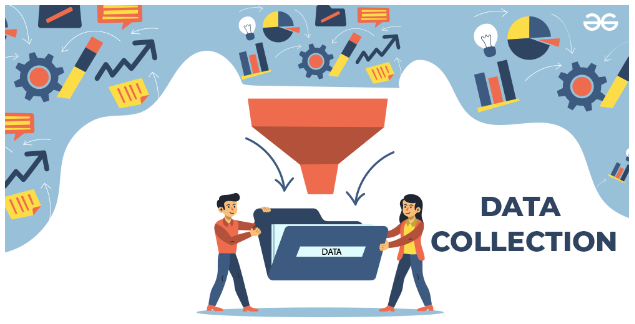
Importance of gathering data in analytics problem solving
Gathering data is a critical step in analytics problem-solving. It involves collecting relevant data from various sources and analyzing it to gain insights to inform decision-making. Gathering data is important for several reasons:
- Identify patterns and trends: Data analysis can help identify patterns and trends that may not be immediately apparent, providing insights to help organizations make informed decisions.
- Identify root causes: Data analysis can help organizations identify the root causes of a problem, allowing them to develop effective solutions that address the underlying issues.
- Measure progress: By collecting and analyzing data, organizations can measure progress towards their business objectives and KPIs, allowing them to evaluate the effectiveness of their solutions.
- Improve decision-making: Data analysis can help organizations make data-driven decisions, reducing the risk of biases and errors in decision-making.
- Identify new opportunities: Data analysis can help organizations identify new opportunities for growth and innovation, providing a competitive advantage.
- Enhance customer experience: By collecting and analyzing customer data, organizations can identify customer needs and preferences, improving the customer experience.
Gathering data is essential for effective analytics problem-solving. By collecting and analyzing data, organizations can gain insights that inform decision-making, identify root causes, measure progress, improve decision-making, identify new opportunities, and enhance the customer experience.
Different ways for gathering data
There are several ways to gather data in analytics problem-solving, including:
- Surveys: Surveys are a common method of gathering data and can be conducted in various formats, such as online surveys, phone surveys, or in-person interviews. Surveys can provide valuable information on customer preferences, behavior, and opinions.
- Focus groups: Focus groups involve bringing together a group of individuals to discuss a particular topic. This method can provide in-depth insights into customer needs and preferences.
- Data mining: Data mining involves analyzing large datasets to identify patterns and trends. This method can provide valuable insights into customer behavior, market trends, and operational efficiency.
- Social media monitoring: Social media monitoring involves monitoring social media platforms for mentions of a particular brand or topic. This method can provide insights into customer sentiment and preferences.
- Web analytics: Web analytics involves analyzing website traffic to gain insights into customer behavior and preferences. This method can provide insights into customer demographics, behavior, and preferences.
- Customer feedback: Customer feedback can be gathered through various methods, such as customer service surveys, online reviews, or feedback forms. This method can provide valuable insights into customer satisfaction and preferences.
- Sales data: Sales data can provide insights into customer behavior, preferences, market trends, and sales performance.
There are several ways to gather data in analytics problem-solving. Organizations should choose the most appropriate methods for their business objectives and KPIs and use them to collect relevant and reliable data. By doing so, organizations can gain insights that inform decision-making and lead to improved business outcomes.
Common challenges to avoid when gathering data
Gathering data is a critical step in analytics problem-solving, and there are several common challenges that organizations should avoid. Some of these challenges include:
- Collecting irrelevant or inaccurate data: Organizations may collect data unrelated to the problem they are trying to solve, or the data collected may be inaccurate, leading to wrong insights.
- Lack of data governance: Organizations may not have a proper data governance framework, leading to data quality issues and silos.
- Incomplete data: Organizations may need to collect more data to get a complete picture of the problem, leading to incomplete insights.
- Privacy and security concerns: Organizations may face privacy and security concerns when collecting data, especially when dealing with sensitive data, such as personal information.
- Lack of resources: Organizations may need more resources, such as budget or personnel, to collect and analyze data effectively.
- Resistance to change: Organizations may face opposition from stakeholders when collecting data, especially when it involves changes to existing processes or systems.
To overcome these challenges, organizations should focus on collecting relevant and accurate data, implementing a robust data governance framework, ensuring data completeness, addressing privacy and security concerns, allocating sufficient resources, and addressing resistance to change through effective communication and stakeholder engagement.
By addressing these challenges, organizations can ensure that they are collecting and analyzing data effectively, leading to better insights and improved decision-making.
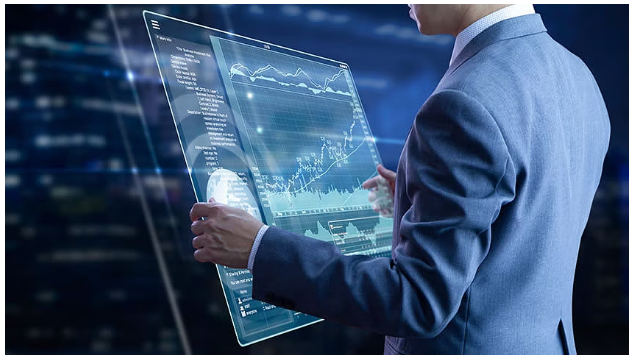
Analyze the Data
Analyzing data is a critical step in analytics problem-solving. It involves using statistical methods and tools to identify data patterns, trends, and insights. Analyzing data is important for several reasons:
- Identify patterns and trends: Data analysis can help identify patterns and trends that may not be immediately apparent, providing insights to help organizations make informed decisions.
- Identify root causes: Data analysis can help organizations identify the root causes of a problem, allowing them to develop practical solutions that address the underlying issues.
- Measure progress: By analyzing data, organizations can measure progress towards their business objectives and KPIs, allowing them to evaluate the effectiveness of their solutions.
- Improve decision-making: Data analysis can help organizations make data-driven decisions, reducing the risk of biases and errors in decision-making.
- Identify new opportunities: Data analysis can help organizations identify new opportunities for growth and innovation, providing a competitive advantage.
- Enhance customer experience: By analyzing customer data, organizations can identify customer needs and preferences, improving the customer experience.
Organizations should use various statistical methods and tools to analyze data effectively, such as regression analysis, clustering, and decision trees. These methods can help identify data patterns, trends, and insights.
Analyzing data is essential for effective analytics problem-solving. By analyzing data, organizations can gain insights that inform decision-making, identify root causes, measure progress, improve decision-making, identify new opportunities, and enhance the customer experience.
Why is analyzing the data important in analytics problem-solving?
Data analysis is important in analytics problem-solving because it helps organizations make informed and data-driven decisions. Data analysis involves using statistical methods and tools to identify patterns, trends, and insights in the data. It is an essential step in the analytics process because it allows organizations to:
- Identify the root causes of the problem: Data analysis can help organizations identify the underlying causes of a pain, allowing them to develop practical solutions that address the underlying issues rather than just the symptoms.
- Measure progress towards business objectives: By analyzing data, organizations can measure progress towards their business objectives and KPIs, allowing them to evaluate the effectiveness of their solutions.
- Identify new opportunities: Data analysis can help organizations identify new opportunities for growth and innovation, providing a competitive advantage.
- Enhance customer experience: By analyzing customer data, organizations can identify customer needs and preferences, improving the customer experience.
- Make data-driven decisions: Data analysis helps organizations make informed and data-driven decisions, reducing the risk of biases and errors in decision-making.
Organizations generate large volumes of data from various sources in today’s fast-paced business environment. However, having data alone is insufficient; organizations need to effectively use that data to derive insights and make informed decisions. Organizations can transform data into a strategic asset that drives business growth and success by analyzing data effectively.
What are some techniques for analyzing the data in analytics?
There are several techniques for analyzing the data in analytics. Some of these techniques include:
- Regression analysis: Regression analysis is a statistical technique used to identify the relationship between a dependent variable and one or more independent variables. It helps organizations understand how changes in one variable impact the other variables.
- Cluster analysis: Cluster analysis is a technique used to group data points into clusters based on their similarities. It helps organizations identify patterns in the data and segment their customers based on their behavior.
- Decision trees: Decision trees are a visual representation of a decision-making process. They help organizations identify the key factors influencing decisions and their relative importance.
- Time series analysis: Time series analysis is a technique used to analyze data that is collected over time. It helps organizations identify trends and patterns in the data and predict future trends.
- Text analytics: Text analytics involves analyzing unstructured data, such as text data from social media, to gain insights into customer sentiment and opinions.
- Data visualization: Data visualization involves presenting data in a visual format, such as charts or graphs, to help organizations identify patterns and trends in the data.
- Machine learning: Machine learning is a technique used to train algorithms to learn from data and make predictions. It helps organizations identify patterns in the data and make predictions about future trends.
By using these techniques to analyze data effectively, organizations can gain insights that inform decision-making, identify root causes, measure progress, improve decision-making, identify new opportunities, and enhance the customer experience.
What are some common mistakes to avoid when analyzing the data in analytics?
Analyzing the data in analytics is a critical step in the decision-making process, and there are several common mistakes that organizations should avoid. Some of these mistakes include the following:
- Using incorrect or irrelevant data: Organizations may use inaccurate or irrelevant data, leading to accurate insights and effective solutions.
- Focusing too much on statistical significance: Organizations may focus too much on statistical significance and ignore the practical importance of the results. This can result in solutions that could be more feasible and practical.
- Not considering the context: Organizations may need to consider the context in which the data was collected, leading to misinterpretation of the results.
- Overfitting the data: Organizations may overfit the data by using complex models that fit the data too closely. This can lead to inaccurate predictions and overconfidence in the results.
- Ignoring outliers: Organizations may need to pay more attention to outliers in the data, leading to inaccurate insights and ineffective solutions.
- Not validating the results: Organizations may not validate them by testing them against new data or using different analysis methods. This can result in solutions that do not generalize to new situations.
To avoid these mistakes, organizations should ensure that they are using correct and relevant data, consider the practical significance of the results, consider the context in which the data was collected, avoid overfitting the data, address outliers, and validate the results. By doing so, organizations can ensure that they analyze the data effectively and make informed decisions based on accurate insights.
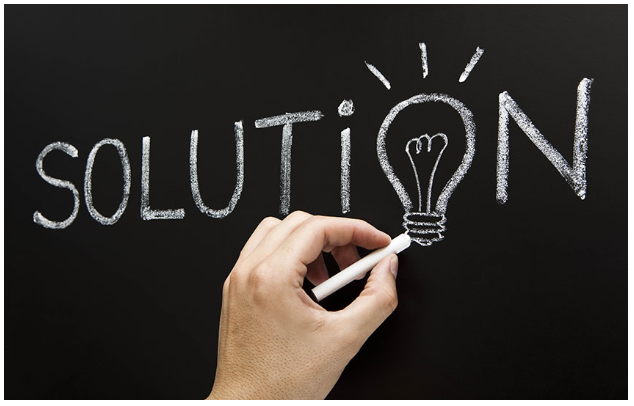
Generate Solutions
Generating solutions is a critical step in analytics problem-solving. It involves using the insights gained from data analysis to develop potential solutions to the problem. Generating solutions is important for several reasons:
- Develop effective solutions: By generating multiple potential solutions, organizations can evaluate each option and choose the most effective one to address the problem.
- Consider all perspectives: By involving stakeholders in the solution-generation process; organizations can consider all perspectives and ensure that the solution meets the needs of all stakeholders.
- Innovate: Generating solutions can lead to innovation and creativity, providing a competitive advantage.
- Improve decision-making: By generating solutions based on data analysis, organizations can make informed and data-driven decisions, reducing the risk of biases and errors in decision-making.
To generate solutions effectively, organizations should use a structured approach, such as brainstorming or decision trees, to evaluate potential solutions. They should involve all relevant stakeholders in the process and consider the feasibility, impact, and cost-effectiveness of each solution. Organizations should also set clear business objectives and KPIs to measure the success of the solutions.
Generating solutions is a critical step in analytics problem-solving. By using insights gained from data analysis and involving stakeholders in the process, organizations can develop effective solutions that address the problem, meet the needs of all stakeholders, and provide a competitive advantage.
Why is generating solutions important in analytics problem solving?
Generating solutions is critical in analytics problem-solving because it helps organizations develop effective strategies to address the problem. By using insights gained from data analysis, organizations can generate potential solutions to the problem and evaluate each option to determine the most effective course of action. Developing solutions is important for several reasons:
- Develop effective solutions: By generating multiple potential solutions, organizations can evaluate each option and choose the most effective one to address the problem.
- Consider all perspectives: By involving stakeholders in the solution-generation process; organizations can consider all perspectives and ensure that the solution meets the needs of all stakeholders.
- Innovate: Generating solutions can lead to innovation and creativity, providing a competitive advantage.
- Improve decision-making: By generating solutions based on data analysis, organizations can make informed and data-driven decisions, reducing the risk of biases and errors in decision-making.
- Increase accountability: By setting clear business objectives and KPIs, organizations can measure the success of the solutions and increase accountability for achieving the desired outcomes.
In today’s fast-paced business environment, generating effective solutions is critical to success. By using insights gained from data analysis to develop potential solutions, organizations can make informed and data-driven decisions that address the underlying issues and lead to improved business outcomes.
What are some techniques for generating solutions in analytics?
There are several techniques for generating solutions in analytics. Some of these techniques include:
- Brainstorming: Brainstorming is a technique used to generate a large number of potential solutions in a short amount of time. It involves bringing together a group of individuals to generate ideas and solutions.
- Decision trees: Decision trees are a visual representation of a decision-making process. They help organizations identify the key factors influencing a decision and their relative importance.
- SWOT analysis: SWOT analysis is a technique used to evaluate the strengths, weaknesses, opportunities, and threats of a potential solution. It helps organizations evaluate the potential impact of a solution and identify potential risks and challenges.
- Cost-benefit analysis: Cost-benefit analysis is a technique used to evaluate the costs and benefits of a potential solution. It helps organizations evaluate the financial impact of a solution and identify potential cost savings.
- Scenario planning: Scenario planning involves developing multiple scenarios for the future and evaluating the potential impact of each scenario on the organization. It helps organizations prepare for potential challenges and identify potential opportunities.
- Root cause analysis: Root cause analysis involves identifying the underlying causes of a problem and developing solutions that address the root causes. It helps organizations develop effective solutions that address the underlying issues rather than just the symptoms.
By using these techniques to generate solutions effectively, organizations can develop effective strategies to address the problem, meet the needs of all stakeholders, and provide a competitive advantage.
What are some common mistakes to avoid when generating solutions in analytics?
Generating solutions in analytics is a critical step in the decision-making process, and there are several common mistakes that organizations should avoid. Some of these mistakes include:
- Not involving all relevant stakeholders: Organizations may only involve some relevant stakeholders in the solution generation process, leading to solutions that do not meet the needs of all stakeholders.
- Not considering all potential solutions: Organizations may not consider all potential solutions, leading to missed opportunities and ineffective solutions.
- Not evaluating the feasibility of the solutions: Organizations may need to evaluate the feasibility of the solutions, leading to solutions that are not practical or cost-effective.
- Not setting clear business objectives and KPIs: Organizations may need to set clear business objectives and KPIs, leading to solutions that do not address the underlying issues or provide the desired outcomes.
- Not considering the potential risks and challenges: Organizations may need to consider the potential risks and challenges associated with the solutions, leading to ineffective solutions or unexpected consequences.
- Not validating the solutions: Organizations may not validate them by testing them against new data or using different analysis methods. This can result in solutions that do not generalize to new situations.
To avoid these mistakes, organizations should involve all relevant stakeholders in the solution generation process, consider all potential solutions, evaluate the feasibility of the solutions, set clear business objectives and KPIs, consider the potential risks and challenges, and validate the solutions. By doing so, organizations can generate effective solutions that address the underlying issues, meet the needs of all stakeholders, and provide the desired outcomes.
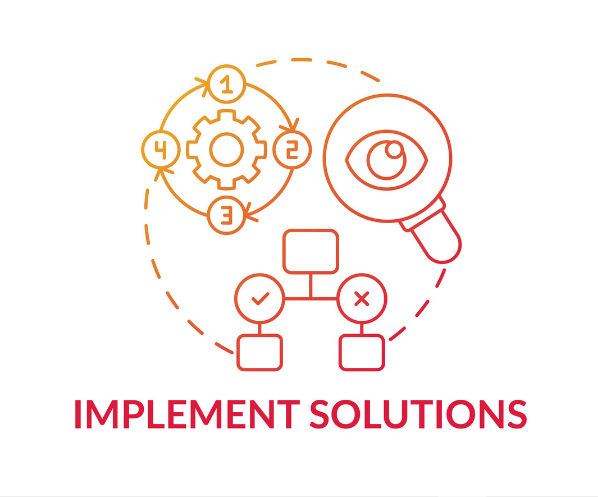
Test and Implement Solutions
Testing and implementing solutions is a critical step in analytics problem-solving. It involves testing the effectiveness of the proposed solutions and implementing the most effective solution to address the problem. Testing and implementing solutions is essential for several reasons:
- Ensure effectiveness: Testing and implementing solutions ensures that the proposed solution effectively addresses the problem.
- Identify potential issues: Testing solutions can help organizations identify potential issues and make necessary adjustments before implementing the solution.
- Minimize risk: Testing solutions before implementation helps minimize the risk of unforeseen consequences or negative impacts.
- Optimize the solution: Testing solutions allow organizations to optimize the solution to maximize its impact and effectiveness.
- Improve decision-making: By testing solutions based on data analysis, organizations can make informed and data-driven decisions, reducing the risk of biases and errors in decision-making.
Organizations should use a structured approach to test and implement solutions effectively, including defining the testing criteria, testing the solutions in a controlled environment, and evaluating the results against the testing criteria. Once the most effective solution has been identified, organizations should develop a detailed implementation plan that includes timelines, resource allocation, and stakeholder engagement.
Testing and implementing solutions is a critical step in analytics problem-solving. By testing solutions before implementation, organizations can ensure their effectiveness, minimize risk, optimize the solution, and make informed and data-driven decisions. By implementing the most effective solution, organizations can address the underlying issues, meet the needs of all stakeholders, and provide the desired outcomes.
Importance of testing and implementing solutions in analytics problem solving
Testing and implementing solutions is a critical step in analytics problem-solving because it ensures that the proposed solution effectively addresses the problem. Testing and implementing solutions is important for several reasons:
- Ensure effectiveness: Testing and implementing solutions ensures that the proposed solution effectively addresses the problem. It helps organizations verify that the solution meets the needs of all stakeholders and achieves the desired outcomes.
- Identify potential issues: Testing solutions can help organizations identify potential issues and make necessary adjustments before implementing the solution. This helps minimize the risk of unforeseen consequences or negative impacts.
- Minimize risk: Testing solutions before implementation helps minimize the risk of unexpected outcomes or negative impacts on the organization or stakeholders.
- Optimize the solution: Testing solutions allow organizations to optimize the solution to maximize its impact and effectiveness. This helps ensure the solution is efficient and effective and provides the best possible outcomes.
- Improve decision-making: By testing solutions based on data analysis, organizations can make informed and data-driven decisions, reducing the risk of biases and errors in decision-making. This helps ensure the solution is based on accurate insights and will likely achieve the desired outcomes.
Testing and implementing solutions are critical to the success of analytics problem-solving. It helps organizations ensure that the proposed solution is effective, efficient and meets the needs of all stakeholders. By implementing the most effective solution, organizations can address the underlying issues, provide the desired outcomes, and achieve business success.
Techniques for testing and implementing solutions
There are several techniques for testing and implementing solutions in analytics. Some of these techniques include
- A/B testing: A/B testing involves testing two or more solution versions to determine the most effective. It helps organizations identify and optimize the most effective solution to achieve better results.
- Pilot testing: Pilot testing involves testing a solution in a small-scale environment before implementing it on a larger scale. It helps organizations identify potential issues and make necessary adjustments before implementing the solution on a larger scale.
- Controlled experiments: Controlled experiments involve testing a solution in a controlled environment to determine its effectiveness. It helps organizations identify potential issues and optimize the solution before implementing it.
- User testing: User testing involves testing a solution with actual users to determine its effectiveness and usability. It helps organizations identify potential issues and make necessary adjustments before implementing the solution.
- Simulation testing: Simulation testing involves testing a solution in a simulated environment to determine its effectiveness. It helps organizations identify potential issues and optimize the solution before implementing it.
- Continuous monitoring: Continuous monitoring involves monitoring the solution’s performance over time to ensure it meets all stakeholders’ needs and achieves the desired outcomes. It helps organizations identify potential issues and make necessary adjustments to ensure the solution remains effective.
By using these techniques to test and implement solutions effectively, organizations can ensure that the proposed solution is effective, efficient, and meets the needs of all stakeholders.
They can optimize the solution to achieve better results and minimize the risk of unforeseen consequences or negative impacts on the organization or stakeholders. They can also make informed and data-driven decisions based on accurate insights, reducing the risk of biases and errors in decision-making.
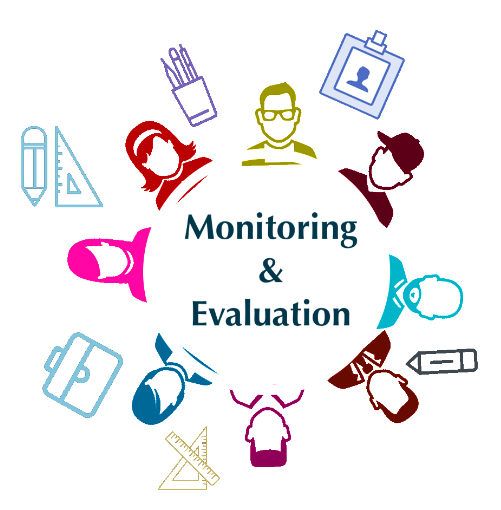
Monitor and Evaluate Results
Monitoring and evaluating the results of a solution is a critical step in analytics problem-solving. It involves tracking the solution’s performance over time to ensure that it continues to meet the needs of all stakeholders and achieve the desired outcomes. Monitoring and evaluating results is essential for several reasons:
- Measure effectiveness: Monitoring and evaluating results allows organizations to measure the solution’s effectiveness and determine whether it achieves the desired outcomes.
- Identify potential issues: Monitoring and evaluating results can help organizations identify potential issues and make necessary adjustments to the solution to improve effectiveness.
- Optimize the solution: Monitoring and evaluating results allows organizations to optimize the solution to maximize its impact and effectiveness.
- Improve decision-making: By monitoring and evaluating results based on data analysis, organizations can make informed and data-driven decisions, reducing the risk of biases and errors.
- Increase accountability: Monitoring and evaluating results increases accountability for achieving the desired outcomes and ensures that the solution remains effective over time.
To monitor and evaluate results effectively, organizations should set clear business objectives and KPIs, establish a regular reporting schedule, track the solution’s performance against the KPIs, and make necessary adjustments based on the results. Organizations should also involve all relevant stakeholders in the monitoring and evaluation process to ensure that the solution continues to meet the needs of all stakeholders.
Monitoring and evaluating the results of a solution is critical to the success of analytics problem-solving. By tracking the solution’s performance over time, organizations can measure its effectiveness, identify potential issues, optimize the solution, and make informed and data-driven decisions. By doing so, organizations can achieve the desired outcomes, meet the needs of all stakeholders, and provide a competitive advantage.
Why is monitoring and evaluating results important in analytics problem-solving?
Monitoring and evaluating results is a critical step in analytics problem-solving. It allows organizations to track the solution’s performance over time, measure its effectiveness, and make necessary adjustments to optimize its impact. Monitoring and evaluating results is essential for several reasons:
- Measure effectiveness: Monitoring and evaluating results allows organizations to measure the solution’s effectiveness and determine whether it achieves the desired outcomes. This helps organizations verify that the solution is effective in addressing the problem.
- Identify potential issues: Monitoring and evaluating results can help organizations identify potential issues and make necessary adjustments to the solution to improve its effectiveness. This helps organizations ensure that the solution remains effective over time.
- Optimize the solution: Monitoring and evaluating results allows organizations to optimize the solution to maximize its impact and effectiveness. By tracking the performance of the solution over time, organizations can identify areas for improvement and make necessary adjustments to improve its effectiveness.
- Improve decision-making: By monitoring and evaluating results based on data analysis, organizations can make informed and data-driven decisions, reducing the risk of biases and errors in decision-making. This helps organizations make better decisions and improve their overall business outcomes.
- Increase accountability: Monitoring and evaluating results increases accountability for achieving the desired outcomes and ensures that the solution remains effective over time. This helps organizations stay focused on achieving their goals and meeting the needs of all stakeholders.
Monitoring and evaluating results is critical to the success of analytics problem-solving. It helps organizations ensure that the proposed solution is effective, efficient and meets the needs of all stakeholders. By optimizing the solution over time, organizations can improve their business outcomes and provide a competitive advantage.
What are some techniques for monitoring and evaluating results in analytics?
There are several techniques for monitoring and assessing developments in analytics. These techniques include:
- Key performance indicators (KPIs): KPIs are metrics used to track the solution’s performance over time. They help organizations measure the solution’s effectiveness and identify improvement areas.
- Dashboards: Dashboards are visual representations of KPIs and other metrics. They allow organizations to monitor the solution’s performance in real time and identify potential issues quickly.
- Data visualization: Data visualization represents data in visual formats such as charts, graphs, and maps. It helps organizations identify trends and patterns in the data and make informed decisions based on the insights.
- Root cause analysis: Root cause analysis involves identifying the underlying causes of the problem and developing solutions that address the root causes. By monitoring and evaluating the results of the solution, organizations can verify that the root causes have been addressed and that the solution remains effective over time.
- Benchmarking: Benchmarking involves comparing the solution’s performance against industry standards or best practices. It helps organizations identify areas for improvement and optimize the solution to achieve better results.
- Surveys and feedback: Surveys and feedback from stakeholders can provide valuable insights into the solution’s effectiveness. By collecting feedback from stakeholders, organizations can identify areas for improvement and make necessary adjustments to the solution.
By using these techniques to monitor and evaluate results effectively, organizations can track the solution’s performance over time, measure its effectiveness, identify potential issues, and make necessary adjustments to optimize its impact. They can also make informed and data-driven decisions based on accurate insights, reducing the risk of biases and errors in decision-making.
Conclusion
Final thoughts on analytics problem solving for effective decision-making
Analytics problem-solving is a critical process that organizations can use to make informed and data-driven decisions. By following a structured approach that includes defining the problem, gathering data, analyzing the data, generating solutions, testing and implementing solutions, and monitoring and evaluating results, organizations can ensure that they are addressing the underlying issues, meeting the needs of all stakeholders, and providing the desired outcomes.
Analytics problem-solving is important for several reasons. It helps organizations identify potential issues, make informed decisions based on accurate insights, and optimize solutions to achieve better results. It also helps organizations increase accountability, improve decision-making, and provide a competitive advantage.
To be successful in analytics problem-solving, organizations should involve all relevant stakeholders in the process, set clear business objectives and KPIs, use data analysis to make informed decisions and monitor and evaluate the results of the solution over time. By doing so, organizations can ensure that they are making effective decisions, meeting the needs of all stakeholders, and achieving their desired outcomes.
In conclusion, analytics problem-solving is a powerful tool organizations can use to make informed, data-driven decisions. Organizations can optimize their solutions and achieve better results by following a structured approach and using the right techniques. Analytics problem-solving is critical to business success and should be a core part of any organization’s decision-making process.