Unleashing the Power of Data Analytics: Fraud Detection and Risk Management
Unleashing the power of data analytics is paramount in fraud detection and risk management. With the exponential growth of digital transactions, organizations face increasing threats of fraudulent activities and associated risks. By harnessing data analytics, companies can gain valuable insights into patterns, anomalies, and trends that help identify potentially fraudulent behavior and mitigate risks effectively.
Statistics reveal the magnitude of the issue. According to a recent report, global losses due to fraud reached a staggering $5.8 trillion in 2020 alone. This highlights the urgent need for robust fraud detection systems. Leveraging advanced analytics techniques, such as machine learning algorithms and predictive modeling, organizations can analyze vast volumes of data, including transactional records, customer behavior patterns, and external data sources.
For instance, a leading financial institution utilized data analytics to combat credit card fraud. By implementing real-time monitoring systems and anomaly detection algorithms, they achieved a remarkable 30% reduction in fraudulent transactions within just six months. Such examples demonstrate the potential impact of data analytics in fortifying fraud prevention strategies.
Moreover, data analytics also plays a crucial role in risk management. By analyzing historical data and market trends, organizations can identify potential risks, anticipate market shifts, and make informed decisions to minimize exposure. For instance, a multinational corporation used predictive analytics to evaluate supply chain risks, resulting in a 15% reduction in inventory holding costs and improved operational efficiency.
The power of data analytics is instrumental in fraud detection and risk management. Organizations can proactively detect and prevent fraudulent activities by leveraging advanced analytics techniques, safeguarding their financial interests. Furthermore, data analytics empowers businesses to manage risks effectively, make informed decisions, and stay ahead in an ever-evolving marketplace.
Significance of data analytics in fraud detection and risk management
Data analytics plays a significant role in fraud detection and risk management, offering invaluable insights and tools to combat emerging threats. Let’s explore the key significance of data analytics in these domains.
- Early Fraud Detection: By leveraging data analytics, organizations can identify patterns, trends, and anomalies within vast volumes of data, enabling early detection of fraudulent activities. Advanced techniques like machine learning and predictive modeling can help detect suspicious transactions, unauthorized access attempts, or abnormal behavior, allowing prompt action to mitigate losses.
- Improved Fraud Prevention: Data analytics enables the development of robust fraud prevention strategies. By analyzing historical data and identifying common fraud indicators, organizations can build algorithms and models to flag potentially fraudulent activities automatically. These systems continuously learn and adapt to new fraud patterns, enhancing prevention measures.
- Real-time Monitoring: With data analytics, real-time monitoring becomes feasible, providing immediate alerts for suspicious activities. By integrating analytics tools into transactional systems, organizations can detect and block fraudulent transactions in real time, preventing financial losses and reducing the impact of fraudulent activities.
- Enhanced Risk Assessment: Data analytics facilitates comprehensive risk assessment by analyzing various data sources, including internal records, market data, and external information. This allows organizations to identify and prioritize potential risks, assess their impact, and allocate resources accordingly. By understanding risk exposure, businesses can make informed decisions to mitigate risks effectively.
- Regulatory Compliance: Data analytics aids in ensuring regulatory compliance by monitoring transactions and activities for potential non-compliance. By analyzing large volumes of data, organizations can identify irregularities, suspicious patterns, or deviations from regulatory requirements, enabling timely corrective actions and avoiding penalties.
- Continuous Improvement: Data analytics provides a feedback loop for continuous fraud detection and risk management improvement. Organizations can refine their models and strategies by analyzing data on successful and unsuccessful fraud cases, adapting to evolving fraud techniques, and staying ahead of fraudsters.
Data analytics is of immense significance in fraud detection and risk management. It empowers organizations to proactively identify and prevent fraudulent activities, assess risks comprehensively, and comply with regulatory requirements. By leveraging data analytics, businesses can protect their financial interests, enhance operational efficiency, and maintain trust in an increasingly digital world.
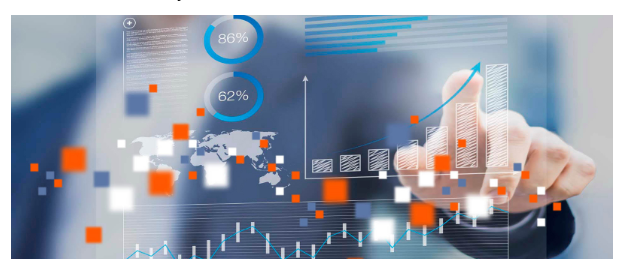
Role of Data Analytics in Fraud Detection
Data analytics is crucial in fraud detection, providing organizations with powerful tools and insights to identify and combat fraudulent activities. Let’s explore the key roles of data analytics in this domain:
- Pattern Recognition: Data analytics helps identify patterns and trends within large volumes of data. Organizations can establish baseline patterns of legitimate activity by analyzing historical transactional data, customer behavior, and other relevant variables. Any deviations from these patterns can be flagged as potential fraud indicators, enabling timely intervention.
- Anomaly Detection: Data analytics enables the detection of anomalies that may indicate fraudulent behavior. Organizations can automatically identify outliers and unusual patterns within data sets using statistical modeling and machine learning algorithms. These anomalies can range from suspicious transactions with unusual amounts, frequencies, or locations to irregular behavior in customer activities.
- Predictive Modeling: Data analytics allows the development of predictive models to forecast the likelihood of fraudulent events. By training these models on historical data, organizations can identify common fraud patterns and generate risk scores or probabilities for new transactions or activities. This helps prioritize resources and focus on high-risk cases for further investigation.
- Real-time Monitoring: Data analytics enables real-time monitoring of transactions and activities, providing immediate alerts for potential fraud. By integrating analytics tools into transactional systems, organizations can analyze data in real-time, comparing incoming transactions against predefined fraud patterns. Suspicious activities can be flagged and acted upon promptly, minimizing potential losses.
- Network Analysis: Data analytics helps uncover complex fraud networks and relationships by analyzing connections between individuals, organizations, and transactions. By leveraging graph analysis techniques, organizations can identify hidden links, common points of compromise, and collusive activities that may not be apparent through traditional methods. This network-centric approach enhances the effectiveness of fraud detection efforts.
- Continuous Improvement: Data analytics enables organizations to improve their fraud detection capabilities continuously. By analyzing data on detected fraud cases and feedback from investigations, organizations can refine their models, update fraud rules, and adapt to emerging fraud techniques. This iterative process ensures that fraud detection systems evolve to stay ahead of sophisticated fraudsters.
Data analytics is pivotal in fraud detection by leveraging advanced techniques to identify patterns, detect anomalies, build predictive models, and enable real-time monitoring. By harnessing the power of data analytics, organizations can enhance their ability to detect and prevent fraudulent activities, safeguarding their assets and maintaining trust with customers.
Leveraging data to identify patterns and anomalies indicative of fraud
Leveraging data to identify patterns and anomalies is a powerful approach to fraud detection. Organizations can uncover indicators that point to potentially fraudulent activities by analyzing large volumes of data. Here are key ways data analysis helps in this process:
- Historical Data Analysis: By examining historical data, organizations can establish patterns of normal behavior and transactional patterns. This serves as a baseline against which current data can be compared. Any deviations from these patterns could indicate fraudulent activities, such as unusual spending patterns, frequent account information changes, or unexpected transaction volume spikes.
- Statistical Analysis: Statistical techniques are crucial in identifying patterns and anomalies. Data analysis methods like regression analysis, clustering, and classification helps identify statistical outliers and unusual data points. These outliers can be potential indicators of fraudulent activities that warrant further investigation.
- Machine Learning Algorithms: Machine learning algorithms excel at detecting complex patterns and anomalies in data. Organizations can develop predictive models that automatically flag suspicious activities by training models on labeled data. These models can analyze multiple variables simultaneously, considering transaction amount, location, time, and customer behavior to detect fraud patterns that may not be apparent through manual analysis.
- Behavior Analysis: Analyzing individual and collective behavior patterns is crucial in fraud detection. Organizations can identify deviations from standard practices by monitoring and analyzing customer behavior data. For example, sudden changes in purchasing habits, unusual login times, or accessing accounts from atypical locations could signal fraudulent behavior.
- Data Visualization: Visualizing data can aid in identifying patterns and anomalies. Data visualization techniques, such as charts, graphs, and heat maps, clearly represent data patterns. Visualization helps analysts identify clusters, trends, or unexpected relationships that may indicate fraudulent activities.
- Real-time Monitoring: Implementing real-time monitoring systems allows organizations to analyze data as it is generated, enabling immediate identification of suspicious activities. This proactive approach helps detect and prevent fraud in real-time, minimizing potential losses.
By leveraging data to identify patterns and anomalies, organizations can improve fraud detection capabilities and take timely action to mitigate risks. These data-driven approaches help build robust fraud detection systems that adapt to evolving fraud techniques, protecting organizations and their customers from financial harm.
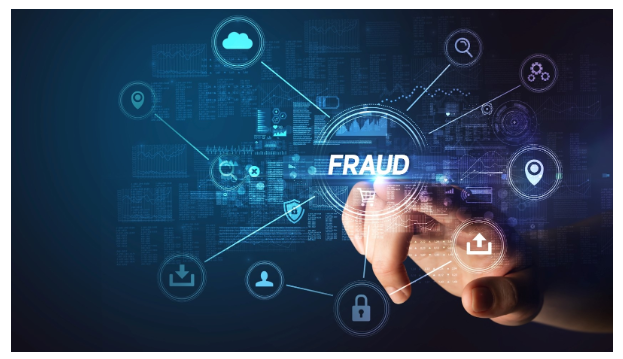
Utilizing predictive modeling and machine learning algorithms for fraud detection
Utilizing predictive modeling and machine learning algorithms is a highly effective approach to fraud detection. These techniques leverage the power of data to identify patterns, predict fraudulent activities, and enhance fraud prevention efforts. Here’s how predictive modeling and machine learning algorithms contribute to fraud detection:
- Anomaly Detection: Predictive models built using machine learning algorithms can identify anomalies within data. By training models on historical data, the algorithms learn normal behavior patterns and can detect unusual or fraudulent activities. Any deviations from expected patterns can be flagged as potential fraud, enabling timely intervention.
- Fraud Risk Scoring: Machine learning algorithms can assign risk scores to individual transactions, accounts, or customers based on their likelihood of being associated with fraudulent behavior. Predictive models can calculate risk scores by analyzing various features such as transaction amount, location, time, user behavior, and historical fraud data. Higher risk scores indicate a greater likelihood of fraud, allowing organizations to prioritize the investigation and take preventive measures.
- Real-time Fraud Detection: Machine learning algorithms can be deployed in real-time fraud detection systems, enabling immediate identification of suspicious activities. By continuously monitoring incoming transactions and comparing them to learned patterns, algorithms can flag potentially fraudulent transactions for further investigation or hold them for additional verification steps. Real-time detection helps prevent fraudulent transactions from being processed, minimizing financial losses.
- Adaptive Learning: Predictive models and machine learning algorithms can adapt and improve over time. The algorithms can learn and incorporate emerging fraud patterns by continually updating the models with new data, including information on newly identified fraud cases. This adaptive learning capability enhances the effectiveness of fraud detection systems and helps stay ahead of evolving fraud techniques.
- Pattern Recognition: Machine learning algorithms recognize complex patterns and relationships within data. By analyzing vast amounts of historical and real-time data, these algorithms can identify hidden patterns, connections, and trends that may not be apparent through traditional rule-based systems. This enables the detection of sophisticated fraud schemes that involve multiple variables and intricate relationships.
- Reduced False Positives: Predictive modeling and machine learning algorithms help reduce false positives by improving fraud detection accuracy. By analyzing multiple data points and considering contextual information, these algorithms can distinguish between genuine and fraudulent transactions more effectively. This reduces the number of legitimate transactions erroneously flagged as potential fraud, improving the overall customer experience.
Predictive modeling and machine learning algorithms are instrumental in fraud detection. These techniques empower organizations to analyze data, detect anomalies, assign risk scores, and adapt to emerging fraud patterns. By leveraging data and advanced analytics, organizations can strengthen their fraud detection capabilities and protect themselves and their customers from financial losses.
Real-time monitoring and proactive fraud prevention using data analytics
Real-time monitoring and proactive fraud prevention using data analytics are crucial in today’s dynamic and fast-paced business environment. By harnessing the power of data analytics, organizations can detect and prevent fraudulent activities in real-time, mitigating risks and minimizing potential losses. Here’s how real-time monitoring and proactive fraud prevention using data analytics work:
- Continuous Data Analysis: Real-time monitoring involves analyzing incoming data streams in real-time as they occur. By leveraging data analytics tools and techniques, organizations can analyze transactional data, user behaviors, and other relevant data sources in real-time. This allows for immediately detecting suspicious activities, enabling proactive fraud prevention.
- Rule-based Systems: Organizations can implement rules that trigger alerts or actions based on predefined rules and thresholds. These rules can be set to flag transactions or activities that meet certain criteria associated with fraudulent behavior. Real-time data analysis against these rules helps identify potential fraud instances as they happen.
- Machine Learning Algorithms: Proactive fraud prevention also involves using machine learning algorithms that learn from historical data and can detect patterns and anomalies in real-time. By training models on labeled data, organizations can build predictive models that can identify and flag suspicious activities in real-time. These algorithms continuously adapt and improve as they process new data, enhancing fraud detection accuracy.
- Behavioral Analytics: Real-time monitoring enables the analysis of user behavior patterns and the detection of anomalies. By establishing baselines of normal behavior, organizations can identify deviations that may indicate fraudulent activities. Real-time behavioral analytics can identify unusual transaction patterns, login anomalies, or account access from suspicious locations, triggering immediate intervention.
- Automated Alerts and Responses: Real-time monitoring allows for automated alerts and responses when suspicious activities are detected. Organizations can configure systems to generate instant alerts to relevant stakeholders or trigger automated actions such as blocking transactions, freezing accounts, or initiating additional verification steps. These proactive measures help prevent further fraudulent activities in real-time.
- Fraud Pattern Recognition: Real-time data analytics enables identifying and recognizing emerging fraud patterns. By analyzing data in real-time, organizations can spot new fraud techniques, schemes, or trends that traditional methods might not capture. This knowledge empowers organizations to proactively update their fraud prevention strategies and stay ahead of evolving threats.
By leveraging real-time monitoring and proactive fraud prevention using data analytics, organizations can detect and respond to fraudulent activities as they happen. This approach minimizes financial losses, protects customer interests, and strengthens overall security measures. Organizations can continuously improve their fraud prevention systems by leveraging the power of data analytics to identify patterns, adapt to new threats, and enhance their overall fraud detection capabilities.
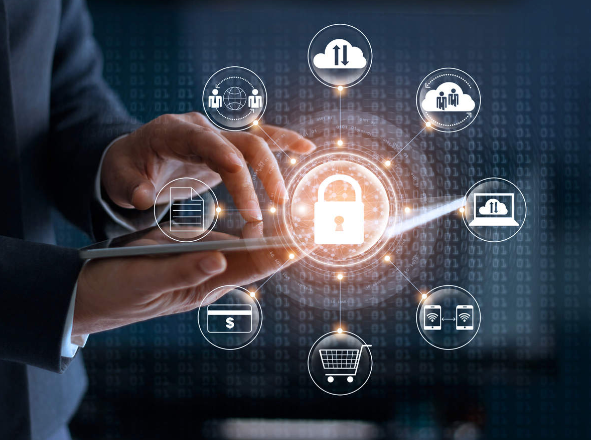
Key Techniques for Fraud Detection with Data Analytics
Regarding fraud detection, data analytics offers a range of powerful techniques that can help identify and prevent fraudulent activities. Here are some key techniques for fraud detection with data analytics:
- Anomaly Detection: Anomaly detection involves identifying unusual patterns or outliers in data that may indicate fraudulent behavior. By leveraging statistical methods and machine learning algorithms, organizations can detect deviations from normal patterns and flag potentially fraudulent activities for further investigation.
- Pattern Recognition: Data analytics enables the identification of patterns and trends within large datasets. Organizations can establish baseline patterns of legitimate activity by analyzing historical transactional data, user behavior, and other relevant variables. Any deviations from these patterns may indicate potential fraud.
- Predictive Modeling: Predictive modeling uses historical data to build models that can predict the likelihood of fraudulent events. Organizations can identify common fraud patterns by training these models on labeled data and generating risk scores or probabilities for new transactions or activities. This helps prioritize resources and focus on high-risk cases.
- Machine Learning Algorithms: Machine learning algorithms, such as decision trees, random forests, and neural networks, can be trained on historical data to detect and classify fraudulent activities automatically. These algorithms learn from past fraud instances and adapt to new patterns, improving their accuracy.
- Network Analysis: Network analysis examines connections and relationships between entities to uncover fraudulent networks or collusive behavior. By analyzing data on transactions, customer relationships, and other network attributes, organizations can identify suspicious links and uncover hidden fraud patterns.
- Text Mining and Natural Language Processing (NLP): Fraudulent activities can be detected by analyzing unstructured data such as text documents, emails, or chat logs. NLP techniques allow organizations to extract meaningful information from text data, enabling the detection of fraudulent intentions, discussions, or hidden patterns.
- Real-time Monitoring: Real-time monitoring involves continuously analyzing incoming data streams to detect and prevent fraud as it happens. By leveraging streaming data analytics platforms, organizations can process and analyze data in real-time, enabling immediate identification of suspicious activities and timely intervention.
- Behavioral Analytics: Behavioral analytics involves analyzing user behavior data to identify patterns and anomalies. Organizations can detect deviations that may indicate fraudulent activities by establishing baseline behavior for individuals or groups. For example, sudden changes in spending habits or access from unusual locations can be red flags.
- Data Visualization: Data visualization techniques help in identifying fraud patterns and outliers by presenting data in a visual format. Interactive dashboards, charts, and graphs enable analysts to explore data, uncover trends, and identify suspicious patterns more effectively.
- Collaborative Analytics: Collaborative analytics involves sharing fraud-related data and insights across organizations or industry sectors. By collaborating and sharing information, organizations can collectively detect and prevent fraud more efficiently, leveraging shared knowledge and resources.
By applying these techniques, organizations can leverage the power of data analytics to detect and prevent fraudulent activities. Each method has its strengths and can be combined to create a comprehensive fraud detection system that continuously evolves to stay ahead of emerging threats.
Benford's Law: Detecting anomalies in numerical data
Benford’s Law is a statistical phenomenon that can be used to detect anomalies in numerical data, including potential fraud. It states that in many real-life datasets, the leading digits of numbers (1, 2, 3, etc.) occur more frequently than the higher digits (8, 9). Understanding and applying Benford’s Law can help identify discrepancies and irregularities in datasets. Here’s how it can be used for fraud detection:
- Leading Digit Analysis: According to Benford’s Law, the distribution of leading digits in a naturally occurring dataset follows a predictable pattern. The digit “1” appears as the leading digit about 30% of the time, followed by “2” at around 17.6%, and so on, decreasing gradually. Deviations from this expected distribution may indicate anomalies in the data.
- Application to Financial Data: Benford’s Law can be applied to financial data, such as transaction amounts, invoice numbers, or sales figures. Analyzing the leading digits in these numbers makes it possible to identify potential anomalies or fraudulent activities. If the leading digit distribution significantly deviates from the expected Benford’s Law distribution, further investigation may be warranted.
- Flagging Potential Fraud: When applying Benford’s Law to numerical datasets, values that deviate significantly from the expected distribution can be flagged as potential anomalies or fraud indicators. These flagged data points can then be subjected to additional scrutiny or investigation to determine if fraudulent activities are occurring.
- Comparative Analysis: Benford’s Law can be used for comparative analysis across different datasets or subgroups within a dataset. For example, if multiple divisions or regions within a company are analyzed, deviations from Benford’s Law may indicate potential discrepancies or fraudulent behavior in specific areas that require further examination.
- Large-scale Data Analysis: Benford’s Law is particularly useful when analyzing large datasets where manual inspection of each data point is impractical. Organizations can efficiently scan vast amounts of numerical data and identify potential fraud-related anomalies by applying automated algorithms or scripts.
It’s important to note that while Benford’s Law is a useful tool for detecting potential anomalies, it does not provide definitive proof of fraud. Further investigation and analysis are necessary to confirm fraudulent activities. Additionally, it is worth considering that Benford’s Law is most applicable to datasets with a wide range of numbers and large sample sizes.
Benford’s Law is a statistical tool that can assist in detecting anomalies in numerical data, potentially indicating fraud. By analyzing the leading digit distribution, organizations can identify data points that deviate from the expected pattern, leading to further scrutiny and investigation to uncover potential fraudulent activities.
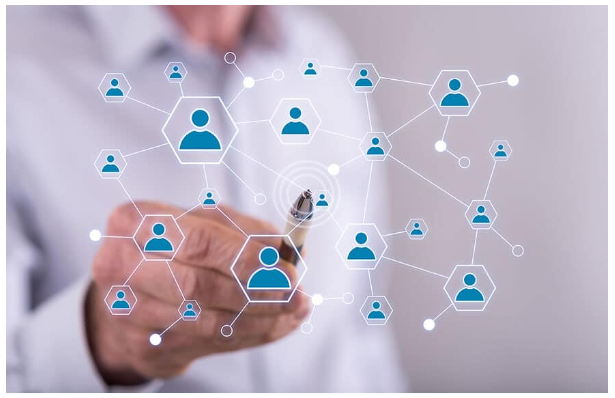
Social network analysis: Identifying connections and networks involved in fraudulent activities
Social network analysis (SNA) is a powerful technique used to identify connections and networks involved in fraudulent activities. By analyzing relationships and interactions among individuals or entities, SNA can uncover hidden patterns, collusion, and the flow of information within fraudulent networks. Here’s how social network analysis aids in detecting fraud:
- Network Mapping: SNA helps map out the connections and relationships among individuals or entities involved in fraudulent activities. By analyzing data such as transaction records, communication logs, or social media interactions, SNA can reveal the network’s structure, identifying key actors and their connections.
- Centrality Analysis: SNA measures centrality to identify influential individuals within a network. Centrality metrics, such as degree centrality, betweenness centrality, or eigenvector centrality, help identify individuals who play crucial roles in connecting different network parts or exerting significant influence. These central individuals may be key perpetrators or facilitators of fraud.
- Community Detection: SNA can identify community clusters within a network based on shared characteristics or interactions. By identifying groups of tightly connected entities within the network, SNA can uncover subgroups involved in specific fraudulent activities. Community detection helps in understanding the structure and dynamics of fraudulent networks.
- Role Identification: SNA helps identify different roles within a network, such as leaders, collaborators, or intermediaries. By analyzing the flow of information, transactions, or influence, SNA can identify individuals who act as key decision-makers, conduits for communication, or facilitators of fraudulent activities. Understanding the roles played by different actors aids in unraveling the network’s dynamics.
- Link Analysis: SNA examines the connections and interactions between entities in a network. Link analysis helps identify suspicious relationships, such as frequent communication or financial transactions between individuals who should not have any legitimate association. Analyzing link patterns can reveal hidden connections, enabling the identification of potential collusion or fraud schemes.
- Identifying Hidden Actors: SNA can uncover hidden actors within a network who may not be immediately obvious or known. By analyzing indirect connections and exploring secondary relationships, SNA can identify individuals who operate behind the scenes or use intermediaries to obscure their involvement in fraudulent activities.
- Predictive Analysis: SNA can be combined with predictive modeling techniques to forecast potential fraudulent activities. By analyzing historical data on fraudulent networks and their characteristics, predictive models can be trained to identify emerging patterns, anticipate new connections, or predict the likelihood of future fraudulent events.
By utilizing social network analysis, organizations can gain valuable insights into the structure, dynamics, and key actors within fraudulent networks. This knowledge aids in identifying and targeting efforts toward disrupting fraudulent activities, mitigating risks, and enhancing fraud prevention strategies.
Behavior analysis: Analyzing user behavior to identify suspicious patterns
Behavior analysis is a powerful technique that analyzes user behavior to identify suspicious patterns or anomalies that may indicate fraudulent activities. By examining how individuals interact with systems, platforms, or processes, behavior analysis can help detect unusual or suspicious behaviors. Here’s how behavior analysis contributes to fraud detection:
- Establishing Baseline Behavior: Behavior analysis establishes a baseline of normal behavior for individuals or groups. Organizations can identify typical user behavior patterns by analyzing historical data and patterns, such as transaction amounts, login times, browsing activities, or purchasing habits. This baseline is a reference point to detect deviations that may indicate fraudulent behavior.
- Deviation Detection: Behavior analysis focuses on identifying deviations from established baseline behavior. Organizations can flag activities outside the expected range by monitoring and comparing current behavior to the baseline. Unusual patterns, such as sudden changes in spending habits, a spike in transaction volume, or irregular access from different locations, can indicate potential fraud.
- Contextual Analysis: Behavior analysis considers contextual factors when analyzing user behavior. It considers the specific circumstances, user roles, and environmental factors that may influence behavior. By considering the context, organizations can differentiate between legitimate variations and suspicious activities. For example, a large purchase may be expected during the holiday season but could be suspicious at other times.
- Machine Learning and Predictive Models: Behavior analysis can leverage machine learning algorithms and predictive models to identify suspicious behavior. These models learn from historical data and can detect patterns or anomalies that may not be apparent through manual analysis. By training models on labeled data, organizations can create algorithms that can automatically flag potentially fraudulent activities based on behavioral patterns.
- Real-time Monitoring: Real-time monitoring is crucial for behavior analysis in fraud detection. Organizations can promptly detect and respond to suspicious activities by continuously analyzing user behavior in real-time. Real-time monitoring allows for immediate alerts or actions when unusual behavior is detected, reducing the potential impact of fraudulent activities.
- User Profiling and Risk Scoring: Behavior analysis contributes to user profiling and risk scoring. Organizations can develop risk scores that indicate the likelihood of fraudulent behavior by considering multiple behavioral factors, such as transaction history, interaction patterns, or user preferences. Users with high-risk scores can be subjected to additional scrutiny or verification steps.
- Cross-Channel Analysis: Behavior analysis involves analyzing user behavior across multiple channels or touchpoints. By examining interactions across platforms, devices, or channels, organizations can detect patterns that may span different systems. This cross-channel analysis helps uncover fraudulent activities involving multiple steps or channels.
Behavior analysis plays a critical role in fraud detection by identifying unusual or suspicious patterns in user behavior. By leveraging this technique, organizations can proactively detect and respond to fraudulent activities, protecting their assets, customers, and reputation.
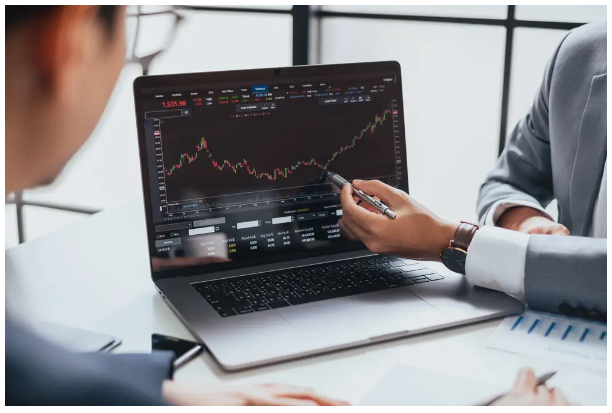
Enhancing Risk Management with Data Analytics
Data analytics is pivotal in enhancing risk management practices across various industries. By leveraging data and advanced analytics techniques, organizations can gain valuable insights, make informed decisions, and mitigate risks effectively. Here’s how data analytics enhances risk management:
- Risk Identification and Assessment: Data analytics enables organizations to identify and assess risks comprehensively. Organizations can identify potential risks and their impact on business operations by analyzing historical data, market trends, and external factors. Advanced analytics techniques, such as predictive modeling, help quantify risks and prioritize them based on their likelihood and potential impact.
- Early Warning Systems: Data analytics allows the development of early warning systems that detect emerging risks or potential issues in real-time. By continuously monitoring key risk indicators and analyzing data from various sources, organizations can receive timely alerts and proactively mitigate risks before they escalate.
- Scenario Analysis and Stress Testing: Data analytics facilitates scenario analysis and stress testing to evaluate the impact of different risk scenarios on business performance. By modeling various hypothetical situations and applying data-driven simulations, organizations can assess their resilience and preparedness to withstand adverse events, helping them make informed decisions and implement risk mitigation strategies.
- Fraud Detection and Prevention: Data analytics aids in fraud detection and prevention by analyzing transactional data, customer behaviors, and patterns. By leveraging techniques like anomaly detection, predictive modeling, and network analysis, organizations can detect fraudulent activities, identify potential fraud networks, and take preventive measures to minimize financial losses.
- Compliance and Regulatory Reporting: Data analytics helps organizations ensure compliance with regulations and reporting requirements. Organizations can take corrective actions, implement robust controls, and generate accurate reports to meet regulatory obligations by analyzing data to identify non-compliant activities, deviations, or anomalies.
- Supply Chain Risk Management: Data analytics enables organizations to assess and mitigate risks in their supply chains. Organizations can identify potential disruptions, vulnerabilities, or bottlenecks by analyzing supply chain data, including supplier performance, lead times, and market trends. This insight helps develop contingency plans, optimize supplier relationships, and enhance overall supply chain resilience.
- Continuous Monitoring and Improvement: Data analytics enables continuous monitoring of risks and their mitigation measures. By analyzing ongoing data, organizations can evaluate the effectiveness of risk controls, identify emerging risks, and adapt risk management strategies accordingly. This iterative process helps organizations stay agile and responsive to evolving risks.
- Business Intelligence and Decision Making: Data analytics gives organizations actionable insights for informed decision-making. Organizations can make data-driven decisions, allocate resources effectively, and proactively manage risks by transforming raw data into meaningful information and visualizations.
In conclusion, data analytics empowers organizations to enhance risk management by identifying risks, detecting fraud, improving compliance, and enabling data-driven decision-making. By harnessing the power of data and advanced analytics techniques, organizations can mitigate risks, seize opportunities, and gain a competitive advantage in an ever-changing business landscape.