In today’s fast-paced world, businesses rely heavily on data to make well-informed decisions. However, with the ever-increasing amounts of data being generated, it’s becoming increasingly difficult to ensure its accuracy and quality. This is where data analytics testing comes into play.
Data analytics testing is a process of evaluating data to ensure its accuracy, completeness, and quality. It helps businesses make confident decisions by providing them with accurate and reliable data. In this article, we’ll take a closer look at data analytics testing and why it’s essential for any business that wants to leverage data to gain a competitive advantage.
Whether you’re a small startup or a large corporation, data analytics testing should be an integral part of your data management strategy. So, let’s dive in and explore the world of data analytics testing and how it can help your business thrive in today’s data-driven world.
What is data analytics testing, and why is it important?
Data analytics testing evaluates the accuracy, completeness, and quality of data used for analysis. The primary objective of data analytics testing is to ensure that the data used for analytics is reliable and trustworthy and that its results are accurate and meaningful.
Data analytics testing is necessary because inaccurate data can lead to erroneous insights and flawed decision-making. Testing the data and validating its quality before using it for analysis is crucial.
By doing so, we can identify and rectify errors in data collection, processing, or storage, which could impact the accuracy of the results.
Moreover, data analytics testing can also help identify data outliers, inconsistencies, and duplications that can distort the analysis results.
We can derive more accurate insights and make informed decisions that drive business growth and success by ensuring data quality.
What are the key components of data analytics testing?
The critical components of data analytics testing are as follows:
- Data Accuracy: Data accuracy is essential to ensure that the results of data analytics testing are reliable. Data accuracy can be tested through profiling, comparison, and quality checks.
- Data Completeness: Data completeness ensures that all the required data is available for analysis. This can be achieved by comparing the data with the source and verifying if all the data has been captured.
- Data Consistency: Data consistency ensures that the data is consistent across all data sets and data sources. It can be tested by comparing data across different sources and verifying if there are any discrepancies.
- Data Relevance: Data relevance ensures that the data used for analytics is relevant to the business needs. It can be tested by verifying the data against the business requirements and objectives.
- Data Timeliness: Data timeliness ensures that the data used for analytics is up-to-date and relevant. It can be tested by comparing the data with the source and verifying the latest updates.
- Data Security: Data security ensures that the data used for analytics is secure and protected. It can be tested by verifying the data access controls, data encryption, and data storage security.
By testing these key components, we can ensure that the data used for analytics is accurate, complete, consistent, relevant, timely, and secure. This can help in generating more accurate insights and making informed decisions.
Why is data analytics testing essential for data-driven decision-making?
Data analytics testing is critical for data-driven decision-making because it ensures that the insights and conclusions drawn from data analytics are accurate, reliable, and trustworthy.
Making decisions based on real or complete data can lead to better business outcomes, wasted resources, and missed opportunities.
Data analytics testing helps to mitigate the risks associated with using data for decision-making by verifying the quality and integrity of the data used in analytics.
By testing the data for accuracy, completeness, consistency, relevance, timeliness, and security, we can identify and correct errors in the data before it is used to make decisions.
Moreover, data analytics testing can help to identify outliers, patterns, and trends in the data that might be missed otherwise. It can also help to identify data correlations and relationships that can be used to make informed decisions.
Data analytics testing is crucial for data-driven decision-making as it ensures that the insights and conclusions drawn from data analytics are accurate, reliable, and trustworthy.
This can help businesses to make informed decisions that drive growth, improve efficiency, and increase profitability.
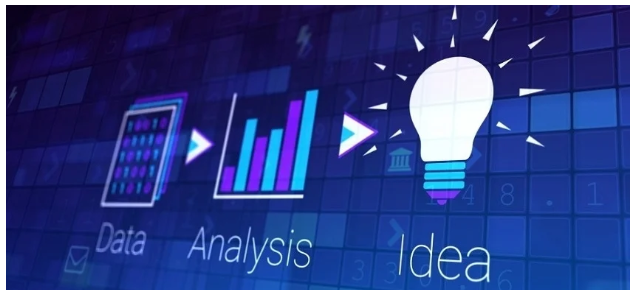
What are some common challenges of using data analytics testing?
There are several challenges associated with data analytics testing. Some of the common challenges are:
- Data Quality: Data quality can make data analytics testing challenging. Data quality issues such as incomplete, inconsistent, or inaccurate data can impact the accuracy of the insights generated from data analytics.
- Data Volume: As the volume of data increases, it can be challenging to test and validate the quality of data effectively. Large data sets can take a long time to process and analyze, making it difficult to detect issues or errors.
- Data Integration: Data analytics testing can be challenging when integrating data from different sources, such as databases, data warehouses, or data lakes. Integration challenges can result in consistency in the data, leading to accurate results.
- Data Privacy: Data privacy and security can be challenging while testing data analytics. Sensitive data such as personally identifiable information (PII) and financial data must be protected and secured from unauthorized access.
- Skilled Workforce: Data analytics testing requires specialized statistical analysis, data mining, and machine learning skills. Organizations may need help finding and retaining qualified personnel with the necessary expertise.
- Infrastructure and Tools: Adequate infrastructure and tools are required to conduct data analytics testing effectively. Organizations may need help providing the necessary infrastructure and tools for testing data analytics.
Data analytics testing faces several challenges, including data quality, volume, integration, privacy, skilled workforce, and infrastructure and tools.
By addressing these challenges, organizations can improve the effectiveness and reliability of data analytics testing, resulting in more accurate insights and better decision-making.
Data Quality Management
Data quality management ensures the accuracy, completeness, consistency, relevance, timeliness, and security of data used for analysis. Effective data quality management involves identifying and correcting errors in data and preventing data quality issues from occurring in the first place.
Data quality management is essential because poor data quality can lead to incorrect insights, inaccurate reporting, and poor decision-making. It can also lead to wasted resources and missed opportunities.
The process of data quality management involves several steps, including:
- Data Profiling: Data profiling involves analyzing and understanding the characteristics of the data. This step can help identify data quality issues such as missing values, outliers, and inconsistencies.
- Data Cleansing: Data cleansing involves correcting errors and inconsistencies in the data. This can be achieved by removing duplicate records, repairing incorrect data, and filling in missing data.
- Data Standardization: Data standardization involves ensuring that the data is in a consistent format and follows a standardized naming convention. This step can help in improving data consistency and accuracy.
- Data Validation: Data validation involves verifying the accuracy and completeness of the data. This can be achieved by comparing the data with the source data and ensuring it meets the required quality standards.
- Data Monitoring: Data monitoring involves continuously monitoring data quality to ensure it remains accurate, complete, and consistent.
Effective data quality management is essential for ensuring accurate insights, reporting, and decision-making. It involves several steps, including data profiling, data cleansing, standardization, validation, and monitoring.
By implementing these steps, organizations can ensure the quality and integrity of their data, leading to improved business outcomes.
Data quality management frameworks
Data quality management frameworks provide a structured approach for managing data quality throughout the data lifecycle. These frameworks typically involve a set of principles, standards, and best practices that guide organizations in managing data quality effectively.
Some of the popular data quality management frameworks are as follows:
- Total Data Quality Management (TDQM): TDQM is a framework emphasizing the importance of data quality at every stage of the data lifecycle, from data acquisition to data archiving.
It involves several steps, including data profiling, cleansing, transformation, and validation. - Data Management Association (DAMA) Data Quality Framework: DAMA is a framework that provides a comprehensive approach to managing data quality. It involves six data quality dimensions: accuracy, completeness, consistency, validity, timeliness, and integrity.
- ISO 8000: ISO 8000 is a data quality management framework that provides a set of standards for managing data quality. It includes guidelines for data governance, profiling, cleansing, and validation.
- Six Sigma: Six Sigma is a data quality management framework that aims to improve data quality by reducing defects in the data. It involves several steps, including data collection, analysis, and process improvement.
- Data Quality Assessment Framework (DQAF): DQAF is a framework that provides a structured approach for assessing data quality. It involves several steps, including data profiling, data quality assessment, data quality improvement, and data quality monitoring.
Data quality throughout the data lifecycle. These frameworks typically involve a set of principles, standards, and best practices that guide organizations in managing data quality effectively.
By implementing these frameworks, organizations can ensure the quality and integrity of their data, leading to improved business outcomes.
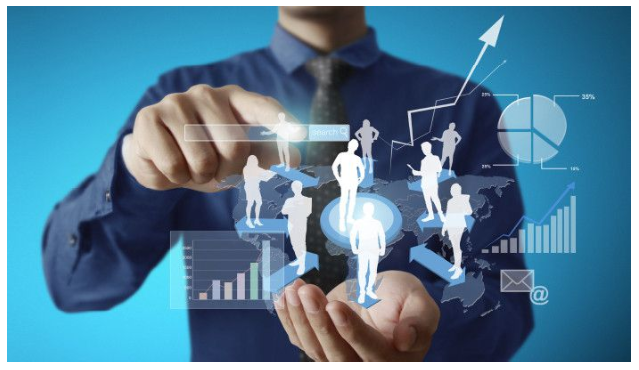
How to establish a data quality management program?
Establishing a data quality management program involves several steps. Some of the key steps are as follows:
- Identify Stakeholders: The first step in establishing a data quality management program is identifying the stakeholders responsible for managing data quality. This could include business leaders, data owners, data stewards, and IT professionals.
- Define Data Quality Standards: The next step is to define data quality standards that reflect the requirements and expectations of the stakeholders. This could include defining data quality metrics, data quality thresholds, and data quality rules.
- Conduct Data Quality Assessment: The next step is to conduct a data quality assessment to identify issues and gaps. This could involve working on data profiling, data validation, and data cleansing.
- Develop Data Quality Improvement Plan: Based on the data quality assessment results, develop a data quality improvement plan that outlines the actions needed to improve data quality. This could involve data cleaning, data enrichment, and data transformation.
- Implement Data Quality Improvement Plan: Implement the data quality improvement plan, which could involve developing data quality dashboards, data quality reports, and data quality metrics.
- Monitor Data Quality: The final step is continuously monitoring data quality. This could involve setting up data quality monitoring processes, data quality audits, and data quality checks.
Establishing a data quality management program involves identifying stakeholders, defining data quality standards, conducting a data quality assessment, developing a data quality improvement plan, implementing the project, and monitoring data quality.
By following these steps, organizations can ensure the quality and integrity of their data, leading to improved business outcomes.
Techniques for monitoring and measuring data quality
There are several techniques for monitoring and measuring data quality. Some of the common methods are as follows:
- Data Profiling: Data profiling is a technique used to measure data quality by analyzing the characteristics of the data. Data profiling helps identify data quality issues such as missing values, outliers, and inconsistencies.
- Data Sampling: Data sampling is a technique used to measure data quality by selecting a subset of data from a more extensive data set. Data sampling helps identify data quality issues in the subset, which can be extrapolated to the more comprehensive data collection.
- Data Cleansing: Data cleansing is a technique used to measure data quality by correcting errors and inconsistencies in the data. Data cleansing involves removing duplicate records, correcting incorrect data, and filling in missing data.
- Data Validation: Data validation is a technique used to measure data quality by verifying the accuracy and completeness of the data. Data validation involves comparing the data with the source data and ensuring it meets the required quality standards.
- Data Auditing: Data auditing is a technique used to measure data quality by examining data management processes and procedures. Data auditing helps identify data quality issues related to data governance, access controls, and security.
- Data Quality Metrics: Data quality metrics are measures used to quantify data quality. Data quality metrics include completeness, accuracy, consistency, and timeliness.
Monitoring and measuring data quality techniques include data profiling, data sampling, data cleansing, data validation, data auditing, and data quality metrics. By using these techniques, organizations can ensure the quality and integrity of their data, leading to improved business outcomes.
Best practices for maintaining data quality
Maintaining data quality is critical for ensuring accurate insights and informed decision-making. Here are some best practices for maintaining data quality:
- Define Data Quality Standards: Establish clear and specific data quality standards that reflect the requirements and expectations of the stakeholders. This could include defining data quality metrics, data quality thresholds, and data quality rules.
- Develop Data Governance Policies: Implement policies defining roles and responsibilities for managing data quality. This could include appointing data stewards, establishing data quality management processes, and representing data ownership.
- Conduct Data Quality Assessments: Conduct regular data quality assessments to identify data quality issues and gaps. This could involve data profiling, data validation, and data cleansing.
- Implement a Data Quality Improvement Plan: Based on the data quality assessment results, develop and implement a data quality improvement plan that outlines the actions needed to improve data quality. This could involve data cleaning, data enrichment, and data transformation.
- Provide Data Quality Training: Provide training to stakeholders on data quality best practices, data quality tools, and techniques for maintaining data quality. This could include data profiling tools, quality metrics, and validation tools.
- Establish Data Quality Monitoring Processes: Establish data quality monitoring processes to maintain data quality over time. This could involve setting up data quality checks, data quality dashboards, and data quality reports.
- Continuously Improve Data Quality: Improve data quality by conducting ongoing data quality assessments, implementing data quality improvement plans, and monitoring data quality constantly.
Maintaining data quality requires a structured approach that involves defining data quality standards, developing data governance policies, conducting data quality assessments, implementing data quality improvement plans, providing data quality training, establishing data quality monitoring processes, and continuously improving data quality.
By following these best practices, organizations can ensure the quality and integrity of their data, leading to improved business outcomes.
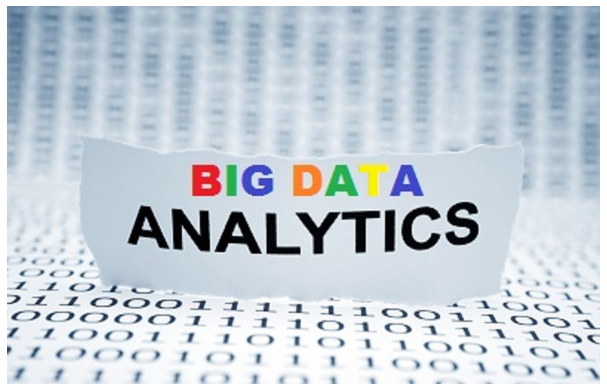
Data Analytics Testing Techniques
Data analytics testing techniques validate the accuracy, completeness, and quality of data used for analysis. Here are some standard data analytics testing techniques:
- Data Profiling: Data profiling involves analyzing the characteristics of the data to identify data quality issues such as missing values, data outliers, and data inconsistencies.
- Data Sampling: Data sampling involves selecting a subset of data from a more extensive data set for testing. Data sampling helps identify data quality issues in the subset, which can be extrapolated to the more comprehensive data set.
- Data Validation: Data validation involves verifying the accuracy and completeness of the data by comparing it with the source data and ensuring that it meets the required quality standards.
- Data Reconciliation: Data reconciliation involves comparing data across different systems or sources to ensure consistency and accuracy.
- Data Cleansing: Data cleansing involves correcting errors and inconsistencies in the data. Data cleansing involves removing duplicate records, correcting incorrect data, and filling in missing data.
- Data Integration Testing: Data integration testing involves testing the accuracy and completeness of data after it has been integrated from multiple sources.
- Metadata Testing: Metadata testing involves testing the accuracy and completeness of the data dictionary and other metadata related to the data.
- Statistical Testing: Statistical testing involves testing the data for statistical significance to ensure that the data is valid for analysis.
Data analytics testing techniques include data profiling, data sampling, validation, reconciliation, cleansing, integration, metadata testing, and statistical testing.
By using these techniques, organizations can ensure the quality and integrity of their data, leading to accurate insights and informed decision-making.
What are data analytics testing techniques?
Data analytics testing techniques validate the accuracy, completeness, and quality of data used for analysis. Here are some standard data analytics testing techniques:
- Data Profiling: Data profiling involves analyzing the characteristics of the data to identify data quality issues such as missing values, data outliers, and data inconsistencies.
- Data Sampling: Data sampling involves selecting a subset of data from a more extensive data set for testing. Data sampling helps identify data quality issues in the subset, which can be extrapolated to the more comprehensive data set.
- Data Validation: Data validation involves verifying the accuracy and completeness of the data by comparing it with the source data and ensuring that it meets the required quality standards.
- Data Reconciliation: Data reconciliation involves comparing data across different systems or sources to ensure consistency and accuracy.
- Data Cleansing: Data cleansing involves correcting errors and inconsistencies in the data. Data cleansing involves removing duplicate records, correcting incorrect data, and filling in missing data.
- Data Integration Testing: Data integration testing involves testing the accuracy and completeness of data after it has been integrated from multiple sources.
- Metadata Testing: Metadata testing involves testing the accuracy and completeness of the data dictionary and other metadata related to the data.
- Statistical Testing: Statistical testing involves testing the data for statistical significance to ensure that the data is valid for analysis.
Data analytics testing techniques include data profiling, data sampling, validation, reconciliation, cleansing, integration, metadata testing, and statistical testing.
By using these techniques, organizations can ensure the quality and integrity of their data, leading to accurate insights and informed decision-making.
What are popular data analytics testing techniques?
Popular data analytics testing techniques include:
- Data profiling: This technique involves analyzing data characteristics to identify data quality issues such as missing values, outliers, and inconsistencies.
- Data sampling: This technique involves selecting a representative subset of data from a more extensive data set for testing purposes. Data sampling helps identify data quality issues in the subset, which can be extrapolated to the more comprehensive data set.
- Data validation: This technique involves verifying the accuracy and completeness of the data by comparing it with the source data and ensuring that it meets the required quality standards.
- Data reconciliation: This technique involves comparing data across different systems or sources to ensure consistency and accuracy.
- Data cleansing: This technique involves correcting errors and inconsistencies in the data. Data cleansing involves removing duplicate records, correcting incorrect data, and filling in missing data.
- Data integration testing: This technique involves testing the accuracy and completeness of data after it has been integrated from multiple sources.
- Metadata testing: This technique involves testing the accuracy and completeness of the data dictionary and other metadata related to the data.
- Statistical testing: This technique involves testing the data for statistical significance to ensure that the data is valid for analysis.
- Exploratory data analysis (EDA): This technique involves visualizing and summarizing the data to identify trends, patterns, and outliers in the data.
Popular data analytics testing techniques include data profiling, data sampling, validation, reconciliation, data cleansing, integration testing, metadata testing, statistical testing, and exploratory data analysis (EDA).
By using these techniques, organizations can ensure the quality and integrity of their data, leading to accurate insights and informed decision-making.
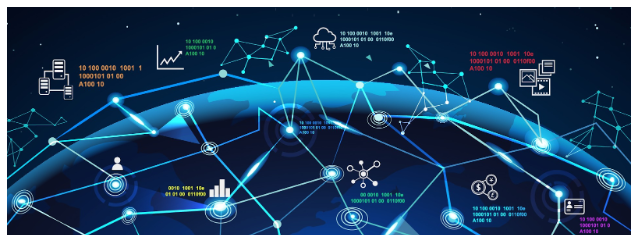
How do I implement data analytics testing techniques?
Implementing data analytics testing techniques involves several steps. Here is a general framework for implementing data analytics testing techniques:
- Define the Scope: The first step is to define the scope of the testing effort. This could include identifying the data sets to be tested, the testing objectives, and the testing schedule.
- Select the Techniques: The next step is to select the appropriate data analytics testing techniques that best suit the testing objectives and the data sets.
This could involve selecting from practices such as data profiling, data sampling, data validation, data reconciliation, data cleansing, data integration testing, metadata testing, statistical testing, and exploratory data analysis (EDA). - Prepare the Test Data: The next step is to prepare the test data for testing. This could involve extracting the required data from the source systems, cleaning the data, and transforming the data as necessary.
- Perform the Tests: The next step is to perform the selected data analytics testing techniques on the test data. This could involve using tools or manual processes to perform the testing.
- Analyze the Test Results: The next step is to analyze the test results to identify data quality issues and gaps. This could involve visualizing the results and identifying data trends, patterns, and outliers.
- Report the Findings: The next step is to report the findings of the testing effort. This could involve creating reports, dashboards, or presentations that summarize the test results and provide recommendations for improvement.
- Implement Improvements: The final step is implementing improvements based on the test findings. This could involve correcting data quality issues, improving data management processes, or enhancing data analytics testing techniques.
Implementing data analytics testing techniques involves defining the scope, selecting the appropriate methods, preparing the test data, performing the tests, analyzing the test results, reporting the findings, and implementing improvements.
By following this framework, organizations can ensure the quality and integrity of their data, leading to accurate insights and informed decision-making.
What is an example of data analytics testing techniques in action?
Here is an example of data analytics testing techniques in action:
Suppose a retail company wants to analyze customer purchase behavior to identify trends and patterns in their buying habits. The company has an extensive database of customer transaction data that must be tested for accuracy and completeness before it can be analyzed.
The company uses data analytics testing techniques to validate the data before analyzing it. The following are the steps taken to implement the testing:
Define the Scope: The testing effort aims to validate the accuracy and completeness of the customer transaction data.
Select the Techniques: The company selects the following data analytics testing techniques:
- Data Profiling: To identify missing values, outliers, and inconsistencies.
- Data Sampling: To select a representative subset of data for testing purposes.
- Data Validation: To verify the accuracy and completeness of the data by comparing it with the source data.
Prepare the Test Data: The company extracts the required data from the source systems, cleans the data, and transforms the data as necessary.
Perform the Tests: The company performs the selected data analytics testing techniques on the test data using data analytics tools.
Analyze the Test Results: The company analyzes the test results to identify data quality issues and gaps. They remember missing values, data outliers, and data inconsistencies in the data.
Report the Findings: The company creates a report summarizing the test results and provides recommendations for improvement. They recommend correcting the missing values, data outliers, and data inconsistencies before proceeding with the analysis.
Implement Improvements: The company implements improvements based on the test findings. They correct the data quality issues, retest the data, and proceed with the analysis.
The retail company used data analytics testing techniques to validate the customer transaction data before analyzing it. By using these techniques, the company ensured the quality and integrity of the data, leading to accurate insights and informed decision-making.
Final Thoughts
Data quality management and data analytics testing are essential for organizations to ensure their data’s accuracy, completeness, and reliability.
By following best practices for data quality management and implementing data analytics testing techniques, organizations can improve the quality and integrity of their data, leading to accurate insights and informed decision-making.
It is essential to continuously monitor and improve data quality, as data is a critical asset for organizations in today’s data-driven world. Data quality management should be a continuous process that involves regular assessments, improvements, and monitoring of data quality metrics.
Finally, staying up-to-date with the latest trends and technologies in data quality management and data analytics testing is essential to remain competitive.
With the increasing importance of data in today’s business environment, organizations that invest in data quality management and data analytics testing will be better equipped to succeed in the long term.
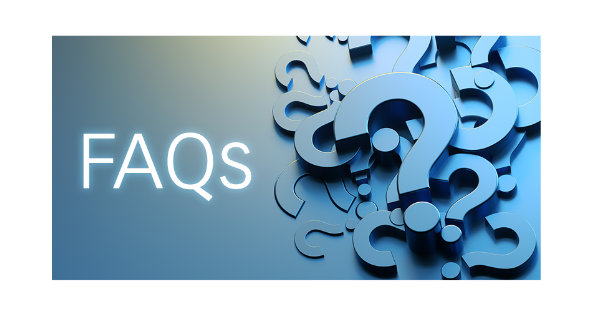
FAQ's
Q: How do I use data analytics testing for data-driven decision-making?
Data analytics testing is a critical component of data-driven decision-making. Here are some ways in which data analytics testing can be used for data-driven decision-making:
- Validate Data Quality: Data analytics testing techniques such as data profiling, data sampling, and data validation can be used to validate the data quality used for analysis. This ensures that data-driven decisions are based on accurate and reliable data.
- Identify Data Quality Issues: Data analytics testing can identify data quality issues such as missing values, outliers, and inconsistencies. By identifying these issues, organizations can take corrective actions to improve the quality of their data and ensure that their data-driven decisions are based on accurate and reliable data.
- Improve Data Management Processes: Data analytics testing can help identify gaps in data management processes that may impact data quality. By identifying these gaps, organizations can take corrective actions to improve their data management processes and ensure that their data-driven decisions are based on accurate and reliable data.
- Monitor Data Quality: Data analytics testing can be used to monitor the quality of data continuously. This ensures that data-driven decisions are based on up-to-date and accurate data.
- Evaluate the Impact of Data-Driven Decisions: Data analytics testing can be used to evaluate the impact of data-driven decisions. This involves analyzing the quality of the data used to make the decision and measuring the outcomes of the decision. This helps organizations to improve their data-driven decision-making processes continuously.
Data analytics testing is critical in ensuring the quality and integrity of data used for data-driven decision-making.
By validating data quality, identifying data quality issues, improving data management processes, monitoring data quality, and evaluating the impact of data-driven decisions, organizations can make better, data-driven decisions based on accurate and reliable data.
Q: What are some best practices for data analytics testing for decision-making?
Here are some best practices for using data analytics testing for decision-making:
- Define Clear Objectives: Clearly define the objectives of the data analytics testing effort, including the specific data sets to be tested and the testing methods to be used.
- Use Appropriate Data Analytics Testing Techniques: Select the appropriate data analytics testing techniques that best suit the testing objectives and the data sets.
This could involve selecting from practices such as data profiling, data sampling, data validation, data reconciliation, data cleansing, data integration testing, metadata testing, statistical testing, and exploratory data analysis (EDA). - Ensure Data Quality: Validate the accuracy and completeness of the data using data analytics testing techniques. Ensure the data is clean, consistent, and reliable before decision-making.
- Establish Data Governance Policies: Policies defining roles and responsibilities for managing data quality. This could include appointing data stewards, establishing data quality management processes, and representing data ownership.
- Monitor Data Quality: Monitor the data quality continuously using data analytics testing techniques to ensure that data-driven decisions are based on up-to-date and accurate data.
- Leverage Visualizations: Use data visualization tools to help identify trends, patterns, and outliers in the data. Visualizing the data can help make it more accessible and easier to understand for decision-makers.
- Continuously Improve: Improve data analytics testing processes and techniques to ensure data-driven decisions are based on the highest quality data available.
Best practices for using data analytics testing for decision-making include defining clear objectives, using appropriate data analytics testing techniques, ensuring data quality, establishing data governance policies, monitoring data quality, leveraging visualizations, and continuously improving.
By following these best practices, organizations can make better, data-driven decisions that are based on accurate and reliable data.
Q: How can I integrate data analytics testing with other marketing tools?
Integrating data analytics testing with other marketing tools can help organizations to gain deeper insights into their marketing performance and make better, data-driven decisions. Here are some ways to integrate data analytics testing with other marketing tools:
- Integrating Data Analytics Testing with Customer Relationship Management (CRM) Tools: Organizations can incorporate data analytics testing with their CRM tools to understand customer behavior and preferences better.
By combining data from different sources, organizations can gain a comprehensive view of their customers and create targeted marketing campaigns. - Integrating Data Analytics Testing with Web Analytics Tools: Organizations can incorporate data analytics testing with their tools to gain insights into website performance and user behavior.
Organizations can optimize website data for better user engagement and conversion rates by analyzing website data. - Integrating Data Analytics Testing with Social Media Tools: Organizations can incorporate data analytics testing with their social media tools to gain insights into social media performance and user behavior.
Organizations can create targeted social media campaigns that engage users and drive conversions by analyzing social media data. - Integrating Data Analytics Testing with Email Marketing Tools: Organizations can incorporate data analytics testing with their email marketing tools to gain insights into email performance and user behavior.
Organizations can optimize email marketing campaigns by analyzing email data for better engagement and conversion rates. - Integrating Data Analytics Testing with Marketing Automation Tools: Organizations can incorporate data analytics testing with their marketing automation tools to gain insights into marketing campaign performance and user behavior.
Organizations can optimize marketing campaign data for better engagement and conversion rates by analyzing marketing campaign data.
Integrating data analytics testing with other marketing tools can help organizations gain deeper insights into their marketing performance and make better, data-driven decisions.
Organizations can create targeted marketing campaigns that engage users and drive conversions by analyzing data from different sources.
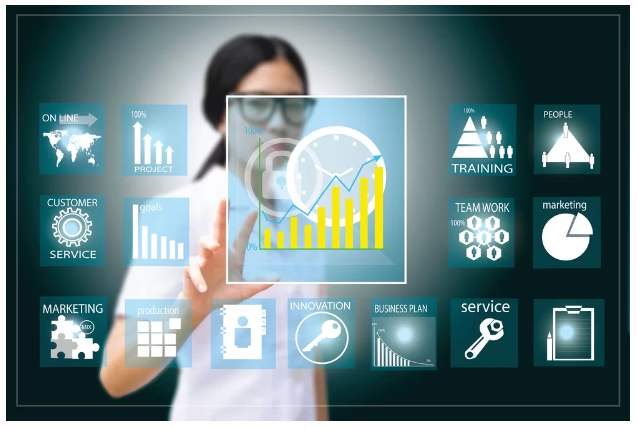
Q: What is an example of using data analytics testing for a specific business need?
Here is an example of using data analytics testing for a specific business need:
Suppose a retail company wants to increase sales by creating targeted marketing campaigns that engage customers and drive conversions. The company has an extensive database of customer transaction data that needs to be analyzed to identify trends and patterns in customer behavior.
The company decides to use data analytics testing techniques to validate the accuracy and completeness of the customer transaction data before analyzing it. The following are the steps taken to implement the testing:
- Define the Scope: The testing effort aims to validate the accuracy and completeness of the customer transaction data.
- Select the Techniques: The company selects the following data analytics testing techniques:
a. Data Profiling: To identify missing values, outliers, and inconsistencies.
b. Data Sampling: To select a representative subset of data for testing purposes.
c. Data Validation: To verify the accuracy and completeness of the data by comparing it with the source data. - Prepare the Test Data: The company extracts the required data from the source systems, cleans the data, and transforms the data as necessary.
- Perform the Tests: The company performs the selected data analytics testing techniques on the test data using data analytics tools.
- Analyze the Test Results: The company analyzes the test results to identify data quality issues and gaps. They remember missing values, data outliers, and data inconsistencies in the data.
- Report the Findings: The company creates a report summarizing the test results and provides recommendations for improvement. They recommend correcting the missing values, data outliers, and data inconsistencies before proceeding with the analysis.
Implement Improvements: The company implements improvements based on the test findings. They correct the data quality issues, retest the data, and proceed with the analysis.
After validating the data quality, the company uses data analytics techniques such as exploratory data analysis and statistical modeling to identify trends and patterns in customer behavior. They use this information to create targeted marketing campaigns that engage customers and drive conversions.
For example, the company may identify that customers who buy a particular product type are likelier to purchase a different product. Based on this insight, they create targeted marketing campaigns that recommend complementary products to customers who have purchased the initial product.
Using data analytics testing techniques to validate their data’s accuracy and completeness, the company could identify trends and patterns in customer behavior and create targeted marketing campaigns that drove sales and increased customer engagement.