In today’s digital era, data-driven analytics has emerged as a powerful tool that revolutionizes decision-making in companies across industries. By harnessing the vast amount of data available, organizations can gain valuable insights that drive strategic and operational choices.
Statistics indicate the significant impact of data-driven decision-making. According to a recent survey, companies that actively utilize analytics are 1.5 times more likely to report higher decision-making maturity. Furthermore, organizations that base their decisions on data-driven insights experience a 5% increase in productivity and a 6% boost in profits.
One prominent example of data-driven analytics transforming decision-making is in the e-commerce sector. Companies like Amazon leverage customer data to personalize recommendations, resulting in a 29% increase in sales. By analyzing browsing behavior, purchase history, and demographic information, they deliver tailored product suggestions that enhance the shopping experience and drive customer satisfaction.
In addition, data-driven analytics enables businesses to optimize their supply chain management. Companies can streamline their operations, reduce costs, and minimize stockouts by examining historical data on demand patterns, inventory levels, and transportation routes. This improves efficiency, faster order fulfillment, and higher customer retention rates.
Data-driven analytics has become a game-changer in decision-making for companies. By leveraging the power of data, organizations can make informed choices, enhance productivity and profitability, and deliver exceptional customer experiences. Embracing this transformative approach enables businesses to stay ahead in today’s competitive landscape.
Explanation of data-driven analytics and its significance in modern business environments
Data-driven analytics refers to using data and advanced analytical techniques to gain insights and make informed decisions in business environments. It involves collecting, processing, and analyzing large volumes of structured and unstructured data to uncover patterns, trends, and correlations that can drive strategic and operational actions.
In modern business environments, data-driven analytics holds immense significance. Here are a few key reasons why:
- Enhanced Decision-Making: Data-driven analytics provides businesses with objective and evidence-based insights. It enables decision-makers to move beyond gut feelings and anecdotal evidence, making decisions based on accurate and quantifiable information. This minimizes risks, improves outcomes, and increases the likelihood of success.
- Competitive Advantage: In today’s highly competitive landscape, gaining a competitive edge is crucial. Data-driven analytics allows companies to uncover hidden opportunities and identify areas for improvement. By leveraging data insights, businesses can make proactive decisions, optimize processes, and innovate more effectively, outperforming their competitors.
- Improved Efficiency and Performance: Analyzing data helps identify bottlenecks, inefficiencies, and areas of underperformance within an organization. Businesses can optimize their operations, streamline workflows, and enhance overall efficiency by addressing these issues. This leads to cost savings, increased productivity, and improved business performance.
- Personalized Customer Experiences: Data-driven analytics enables businesses to understand their customers better. By analyzing customer data, such as demographics, preferences, purchase history, and behavior, companies can personalize their marketing messages, product recommendations, and customer experiences. This results in higher customer satisfaction, increased engagement, and improved customer retention rates.
- Predictive Insights: Leveraging historical data and predictive modeling techniques, data-driven analytics empowers businesses to forecast future trends, behaviors, and outcomes. This helps organizations make proactive decisions, anticipate market changes, identify emerging opportunities, and mitigate potential risks.
- Agile Decision-Making: Data-driven analytics facilitates real-time or near real-time analysis of data. This enables businesses to respond quickly to market dynamics, customer needs, and emerging trends. Agile decision-making based on up-to-date data ensures organizations remain adaptable, responsive, and better equipped to capitalize on changing circumstances.
Data-driven analytics is of paramount importance in modern business environments. It empowers organizations to make informed decisions, gain a competitive advantage, improve efficiency and performance, deliver personalized customer experiences, leverage predictive insights, and enable agile decision-making. Embracing data-driven approaches is vital for businesses looking to thrive and succeed in today’s data-rich world.
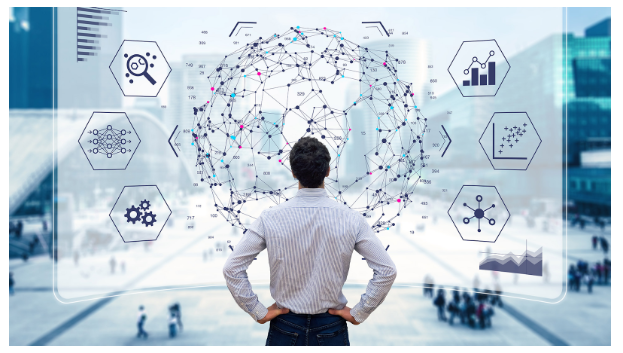
Importance of data-driven decision-making for companies' success and growth
Data-driven decision-making is essential for the success and growth of companies in today’s highly competitive business landscape. Here are some key reasons why data-driven decision-making is of utmost importance:
- Objective Insights: Data-driven decision-making relies on factual data and analysis rather than subjective opinions or assumptions. It provides objective insights into the current state of the business, market trends, customer behavior, and other relevant factors. By basing decisions on reliable data, companies can minimize biases and make more accurate choices.
- Identifying Opportunities: Data-driven decision-making helps companies identify hidden opportunities for growth. By analyzing data, businesses can uncover emerging market trends, customer preferences, and gaps in the market. This allows them to develop innovative products and services that meet customers’ evolving needs, leading to a competitive advantage.
- Mitigating Risks: Data-driven decision-making enables companies to assess and mitigate risks effectively. By analyzing historical data and market trends, businesses can anticipate potential risks and take proactive measures to minimize their impact. This could include identifying potential operational bottlenecks, predicting supply chain disruptions, or identifying financial risks.
- Enhanced Customer Experience: Data-driven decision-making enables companies to understand their customers better. Businesses can gain insights into their preferences, purchase behavior, and feedback by analyzing customer data. This information helps personalize marketing campaigns, improve product offerings, and deliver exceptional customer experiences, ultimately increasing customer satisfaction and loyalty.
- Operational Efficiency: Data-driven decision-making optimizes operational processes and resource allocation. By analyzing data on operational performance, companies can identify inefficiencies, bottlenecks, and areas of improvement. This allows for informed decisions on process optimization, resource allocation, and cost management, improving operational efficiency and cost savings.
- Continuous Improvement: Data-driven decision-making promotes a culture of continuous improvement within organizations. By monitoring and analyzing data, companies can measure the effectiveness of their strategies and initiatives. This data-driven feedback loop enables organizations to make data-backed adjustments, refine their approaches, and drive continuous growth and improvement.
- Competitive Advantage: In today’s data-driven world, companies that effectively leverage data to drive decision-making gain a competitive edge. Businesses can respond quickly to market changes, customer demands, and emerging trends by making informed decisions based on data insights. This agility and adaptability allow companies to stay ahead of the competition and seize growth opportunities.
Data-driven decision-making is vital for companies success and growth. It provides objective insights, identifies opportunities, mitigates risks, enhances the customer experience, optimizes operations, fosters continuous improvement, and provides a competitive advantage. By embracing data-driven approaches, companies can make informed decisions that drive their success in a rapidly evolving business landscape.
Benefits of Data-Driven Analytics
Data-driven analytics offers numerous benefits for businesses across industries. Here are some key advantages:
- Informed Decision-Making: Data-driven analytics provides businesses with accurate and relevant information to make informed decisions. Organizations gain valuable insights into customer behavior, market trends, operational efficiency, and more by analyzing data from various sources. This enables decision-makers to make choices based on evidence rather than assumptions, leading to better outcomes.
- Improved Efficiency and Productivity: Data-driven analytics helps identify inefficiency and inefficiencies within business processes. Organizations can pinpoint bottlenecks, streamline workflows, and optimize resource allocation by analyzing data. This leads to improved efficiency, reduced costs, and increased productivity, ultimately driving business growth.
- Enhanced Customer Understanding: Data-driven analytics allows businesses to better understand their customers. Companies can identify patterns, preferences, and trends by analyzing customer data, including demographics, purchase history, and online behavior. This insight helps tailor marketing strategies, personalize customer experiences, and deliver relevant products or services, increasing customer satisfaction and loyalty.
- Competitive Advantage: Leveraging data-driven analytics provides a competitive edge in the marketplace. Businesses can identify opportunities, optimize pricing strategies, and differentiate their offerings by analyzing industry trends, competitor performance, and customer insights. This allows companies to stay ahead of the competition and adapt to changing market conditions effectively.
- Risk Mitigation: Data-driven analytics helps identify and mitigate potential risks. Organizations can detect anomalies, predict future outcomes, and assess potential risks by analyzing historical and real-time data. This enables proactive risk management and the implementation of strategies to minimize or avoid potential negative impacts.
- Innovation and New Revenue Streams: Data-driven analytics can uncover untapped opportunities for innovation and new revenue streams. By analyzing market data, consumer trends, and emerging technologies, organizations can identify gaps in the market and develop innovative products or services to meet customer demands. This promotes business growth and opens up new avenues for revenue generation.
- Continuous Improvement: Data-driven analytics fosters a culture of continuous improvement within organizations. By regularly analyzing data and performance metrics, businesses can identify areas for improvement, monitor the effectiveness of strategies, and make data-backed adjustments. This iterative process of improvement ensures ongoing growth and competitiveness.
Data-driven analytics offers numerous benefits, including informed decision-making, improved efficiency and productivity, enhanced customer understanding, a competitive advantage, risk mitigation, innovation, and continuous improvement. By harnessing the power of data, businesses can drive growth, profitability, and success in today’s data-driven world.
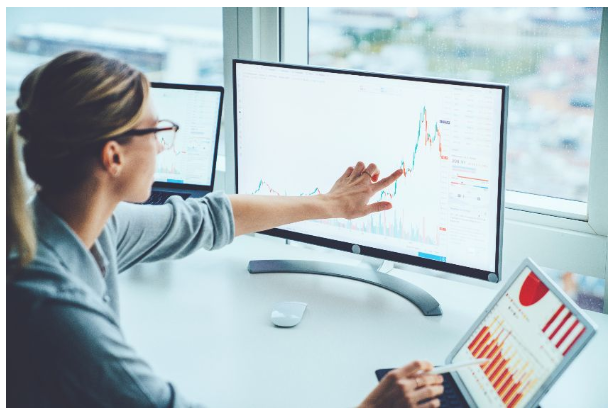
Overview of the advantages companies gain from implementing data-driven analytics
Implementing data-driven analytics provides companies with a range of advantages that contribute to their success. Here’s an overview of the key benefits:
- Actionable Insights: Data-driven analytics empowers companies to extract actionable insights from large, complex datasets. By leveraging advanced analytical techniques, businesses can identify patterns, trends, and correlations in their data. These insights enable informed decision-making, improving strategies, operational efficiency, and better outcomes.
- Improved Decision-Making: Data-driven analytics helps organizations make smarter decisions based on factual evidence rather than intuition or guesswork. Businesses comprehensively understand their operations, market dynamics, and customer behavior by analyzing historical and real-time data. This enables decision-makers to make data-backed choices that drive business growth and mitigate risks.
- Enhanced Operational Efficiency: Implementing data-driven analytics allows companies to optimize their operations and enhance efficiency. Organizations can identify bottlenecks, streamline workflows, and allocate resources effectively by analyzing data related to processes, resource allocation, and performance metrics. This leads to cost savings, increased productivity, and improved operational effectiveness.
- Personalized Customer Experiences: Data-driven analytics enables companies to deliver personalized customer experiences. Organizations can tailor their marketing efforts, product recommendations, and customer service interactions by analyzing customer data, including preferences, purchase history, and demographics. This personalization enhances customer satisfaction, engagement, and loyalty.
- Competitive Advantage: Companies that leverage data-driven analytics gain a competitive edge in the market. Businesses can identify opportunities, optimize pricing, and differentiate their offerings by utilizing data to understand market trends, customer preferences, and competitor strategies. This enables them to stay ahead of the competition and adapt quickly to changing market conditions.
- Effective Risk Management: Data-driven analytics helps companies identify and mitigate risks effectively. Organizations can anticipate potential risks by analyzing data for anomalies, trends, and predictive indicators and take proactive measures to mitigate them. This minimizes financial, operational, and reputational risks, ensuring business continuity and stability.
- Innovation and Business Growth: Data-driven analytics fuels innovation and drives business growth. By exploring data and identifying insights, companies can uncover new opportunities, develop innovative products or services, and enter new markets. Data-driven insights also help organizations identify areas for improvement and innovation within existing processes and products, fostering continuous growth.
- Measurable Results and ROI: Implementing data-driven analytics allows companies to accurately measure and track their progress. By setting key performance indicators (KPIs) and analyzing data, businesses can measure the impact of their strategies and initiatives. This enables them to make data-backed decisions, optimize their investments, and demonstrate a return on investment (ROI) for their data-driven initiatives.
Companies gain significant advantages from implementing data-driven analytics, including actionable insights, improved decision-making, enhanced operational efficiency, personalized customer experiences, competitive advantage, effective risk management, innovation, and measurable results. By embracing data-driven approaches, organizations can optimize their operations, drive growth, and succeed in today’s data-rich business environment.
Enhanced decision-making accuracy, efficiency, and effectiveness
Implementing data-driven analytics enhances decision-making accuracy, efficiency, and effectiveness in several ways:
- Accurate Insights: Data-driven analytics provides objective and accurate insights by analyzing large volumes of data. This ensures decision-makers have a comprehensive and reliable understanding of the business landscape, market trends, customer behavior, and operational performance. The accuracy of data-driven insights minimizes the risk of making decisions based on incomplete or biased information.
- Real-time Analysis: Data-driven analytics enables real-time or near-real-time analysis of data. This allows decision-makers to access up-to-date information, monitor key metrics, and respond quickly to changing circumstances. Real-time insights enable agile decision-making, ensuring businesses can make timely adjustments, seize opportunities, and mitigate risks effectively.
- Data Visualization: Data-driven analytics often employs data visualization techniques, such as charts, graphs, and dashboards, to present complex data in a visually appealing and easy-to-understand manner. Visual representations facilitate quicker comprehension of information, enabling decision-makers to grasp patterns, trends, and relationships at a glance. This enhances decision-making efficiency by reducing the time required to interpret data.
- Predictive Analytics: Data-driven analytics leverages predictive modeling and forecasting techniques to anticipate future outcomes based on historical data patterns. By using predictive analytics, decision-makers can make proactive choices, optimize resource allocation, and anticipate market trends. This proactive approach minimizes reactive decision-making and allows businesses to stay ahead of the curve.
- Scenario Analysis: Data-driven analytics enables decision-makers to conduct scenario analysis and simulations. By manipulating variables and exploring hypothetical scenarios, businesses can assess the potential impact of different decisions before implementation. This helps evaluate various options, compare outcomes, and select the most favorable action, increasing decision-making effectiveness.
- Data-driven Decision Support Systems: Implementing data-driven decision support systems gives decision-makers access to relevant, timely information. These systems integrate data from various sources, apply advanced analytics techniques, and deliver actionable insights directly to decision-makers. Decision support systems enhance decision-making efficiency and effectiveness by automating data analysis and delivering insights in a user-friendly format.
- Performance Measurement and Evaluation: Data-driven analytics allows businesses to measure and evaluate the performance of their decisions. By setting clear metrics and tracking key performance indicators (KPIs), organizations can assess the effectiveness of their decisions over time. This feedback loop helps decision-makers refine their strategies, learn from past experiences, and continuously improve decision-making outcomes.
Data-driven analytics enhances decision-making accuracy by providing reliable and objective insights. It improves decision-making efficiency through real-time analysis, data visualization, and scenario analysis. Furthermore, data-driven analytics boosts decision-making effectiveness by leveraging predictive analytics and facilitating performance measurement and evaluation. By harnessing the power of data, businesses can make more accurate, efficient, and effective decisions that drive success and growth.
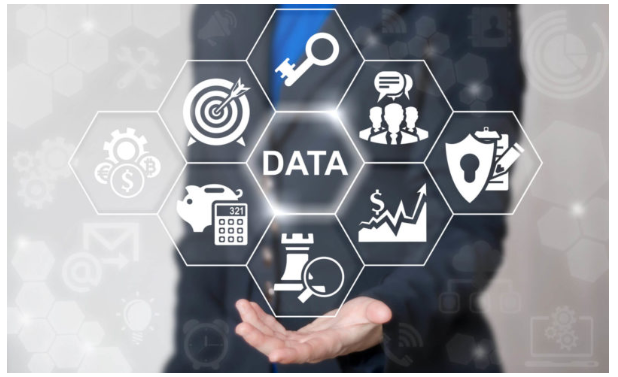
Key Components of a Data-Driven Analytics Framework
A data-driven analytics framework comprises several key components that enable effective data analysis and decision-making. Here are the essential components of a data-driven analytics framework:
- Data Collection: This component involves identifying and collecting relevant data from various sources, including internal databases, external APIs, customer interactions, social media, and more. It includes defining data requirements, establishing data governance practices, and implementing data collection methods to ensure high-quality and reliable data availability.
- Data Integration and Storage: Once collected, data must be integrated from different sources and stored in a centralized and accessible manner. This involves data transformation, cleansing, and normalization to ensure data consistency and compatibility. Data warehouses or data lakes are commonly used to store and manage large volumes of structured and unstructured data.
- Data Processing and Analysis: This component focuses on processing and analyzing the collected data to derive meaningful insights. It involves data exploration, cleaning, aggregation, statistical analysis, machine learning, and other analytical techniques. Advanced analytics tools and algorithms are applied to uncover patterns, correlations, trends, and anomalies in the data.
- Data Visualization: Data visualization is crucial for presenting complex data in a visually intuitive and understandable format. This component involves creating visual representations such as charts, graphs, dashboards, and reports to communicate insights effectively. Data visualization tools enable decision-makers to quickly grasp information, identify patterns, and make informed decisions.
- Predictive Analytics and Modeling: Predictive analytics leverages historical data to forecast future trends, behavior, and outcomes. This component uses statistical modeling, machine learning algorithms, and predictive analytics techniques to develop predictive models. These models enable organizations to make data-driven predictions and simulate scenarios for better decision-making.
- Decision Support Systems: Decision support systems (DSS) provide a platform for integrating data analytics into decision-making. DSS tools offer interactive interfaces, data visualization capabilities, and access to relevant insights and reports. These systems enable decision-makers to access real-time information, perform ad-hoc analysis, and make informed decisions based on data-driven insights.
- Performance Measurement and Evaluation: This component involves defining key performance indicators (KPIs) and establishing metrics to measure the effectiveness of decisions and analytics initiatives. Performance measurement and evaluation help assess the impact of data-driven analytics on business outcomes, identify areas for improvement, and refine decision-making processes.
- Continuous Improvement and Iteration: A data-driven analytics framework should promote a culture of continuous improvement. This involves regularly reviewing and refining the framework, updating data collection methods, enhancing analysis techniques, and incorporating feedback from decision-makers. Continuous improvement ensures that the framework evolves with changing business needs and technological advancements.
By incorporating these key components, organizations can establish a robust data-driven analytics framework that enables effective data analysis, insights generation, and informed decision-making across various business functions.
Overcoming Challenges in Implementing Data-Driven Analytics
Implementing data-driven analytics can be accompanied by several challenges. However, organizations can overcome these challenges through proactive measures and strategic approaches. Here are some common challenges and potential solutions for successfully implementing data-driven analytics:
- Data Quality and Accessibility: Ensuring data quality and accessibility is crucial for effective analytics. Organizations should invest in data governance practices, establish quality standards, and implement data cleansing processes to address inconsistencies and inaccuracies. Creating a centralized data repository and improving data integration capabilities can also enhance data accessibility and reduce silos.
- Data Security and Privacy: With increased data usage comes the responsibility of protecting sensitive information. Organizations should prioritize data security and privacy by implementing robust security measures, adhering to regulatory requirements (e.g., GDPR, CCPA), and employing encryption techniques. Data access controls, user permissions, and regular security audits are essential for maintaining data confidentiality and integrity.
- Skill Gaps and Talent Acquisition: Building a skilled analytics team can be challenging due to the need for more data scientists and analysts. Organizations can address this by investing in training programs to upskill existing employees, partnering with external experts or consultants, or leveraging automated analytics tools that require minimal coding skills. Collaborating with academic institutions and promoting data literacy within the organization can also help bridge skill gaps.
- Organizational Culture and Change Management: Implementing a data-driven culture requires organizational buy-in and change management. Leaders should communicate the value of data-driven decision-making, foster a culture that embraces data, and incentivize employees to utilize data insights. Training, support, and resources for employees to adopt data-driven practices will facilitate a smoother transition.
- Technology Infrastructure and Scalability: Implementing data-driven analytics requires a robust technology infrastructure that can handle large volumes of data and scale as needed. Investing in appropriate hardware, software, and cloud-based solutions can ensure a reliable and scalable infrastructure. Regular assessments of technology needs and upgrades will help meet evolving analytical requirements.
- Aligning Analytics with Business Goals: Data-driven analytics should align with the organization’s strategic objectives. Defining clear business goals and identifying key performance indicators (KPIs) that align with those goals is crucial. This ensures that analytics efforts are focused on providing actionable insights that directly contribute to business success.
- Change Resistance and Adoption: Resistance to change can hinder the implementation of data-driven analytics. Effective change management strategies, such as education and communication programs, stakeholder involvement, and showcasing early successes, can address resistance and encourage the widespread adoption of data-driven practices.
- Iterative Approach and Continuous Improvement: Implementing data-driven analytics is an iterative process. It is important to start with specific use cases and gradually expand the scope based on lessons learned. Continuous monitoring, evaluation, and refinement of the analytics framework allow organizations to adapt to evolving needs, emerging technologies, and changing business requirements.
By addressing these challenges and adopting a holistic approach, organizations can successfully implement data-driven analytics and leverage its full potential to drive informed decision-making, gain competitive advantages, and foster business growth.
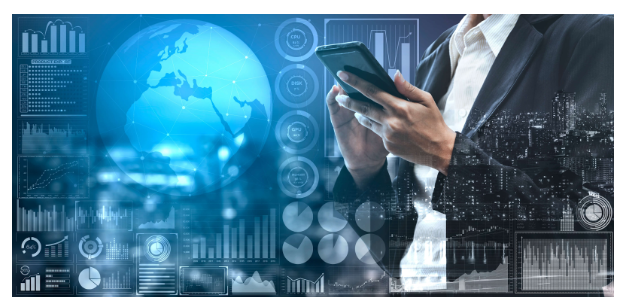
Successful Case Studies
Certainly! Here are a few successful case studies highlighting the implementation of data-driven analytics:
- Netflix: Netflix, the global streaming giant, extensively uses data-driven analytics to personalize user experiences and recommend content. By analyzing user viewing habits, ratings, and preferences, Netflix leverages machine learning algorithms to provide tailored recommendations, increasing user engagement and retention. Their recommendation engine is estimated to save the company $1 billion annually by reducing churn.
- Amazon: Amazon, the world’s largest online retailer, is renowned for its data-driven approach. Their data analytics capabilities optimize inventory management, pricing strategies, and customer experiences. By analyzing customer behavior, Amazon is able to deliver highly targeted and personalized product recommendations, leading to increased sales and customer satisfaction.
- Walmart: a global retail giant, Walmart relies on data-driven analytics to optimize its supply chain and inventory management. By analyzing historical sales data, product demand patterns, and customer behavior, Walmart has implemented sophisticated forecasting models to predict demand accurately. This enables them to optimize inventory levels, reduce stockouts, and streamline their supply chain operations, resulting in significant cost savings.
- Starbucks: Starbucks utilizes data-driven analytics to enhance customer experiences and drive operational efficiency. Through its loyalty program and mobile app, Starbucks collects valuable customer data, including purchase history and preferences. They leverage this data to personalize promotions, optimize store layouts, and streamline operations. The data-driven approach has helped Starbucks increase customer loyalty and drive revenue growth.
- Spotify: Spotify, the popular music streaming platform, leverages data-driven analytics to curate personalized playlists and recommend songs to its users. By analyzing user listening habits, Spotify’s algorithms generate personalized playlists based on individual preferences, historical data, and music trends. This data-driven approach has been instrumental in driving user engagement and retention.
These case studies highlight how organizations across different industries have successfully implemented data-driven analytics to gain a competitive advantage, optimize operations, and deliver personalized experiences to their customers. By leveraging data and analytics effectively, these companies have achieved remarkable success and continue to innovate in their respective industries.
Best Practices for Embracing Data-Driven Analytics
To effectively embrace data-driven analytics, organizations should follow certain best practices. Here are some key practices to consider:
- Define Clear Objectives: Clearly define the business objectives you aim to achieve through data-driven analytics. Whether it’s improving customer satisfaction, optimizing operations, or driving revenue growth, having well-defined goals provides a clear direction for your analytics initiatives.
- Identify Relevant Data: Identify the data types relevant to your business objectives. This includes internal data (such as customer, sales, and operational data) and external data (such as market trends, social media data, and competitor data). Determine the sources, quality, and accessibility of the data required to support your analytics efforts.
- Ensure Data Quality: Establish processes to ensure data quality and integrity. This includes data cleansing, normalization, and validation to address inconsistencies and inaccuracies. Implement data governance practices to maintain data accuracy, reliability, and regulation compliance.
- Invest in Data Infrastructure: Invest in the right infrastructure to support your data-driven analytics initiatives. This may involve data storage solutions, cloud-based platforms, and data integration tools. Ensure scalability and flexibility to accommodate growing data volumes and evolving analytics needs.
- Develop Analytical Capabilities: Build a skilled analytics team or upskill existing employees to analyze and interpret data effectively. This includes data scientists, analysts, and engineers with the necessary technical and analytical skills. Provide training programs, access to analytics tools, and opportunities for continuous learning to enhance analytical capabilities.
- Leverage Advanced Analytics Techniques: Utilize advanced analytics techniques, such as machine learning, predictive modeling, and data visualization, to uncover valuable insights. These techniques help in identifying patterns, correlations, and trends that may not be evident through traditional analysis methods.
- Foster a Data-Driven Culture: Cultivate a culture that values data-driven decision-making. Encourage employees to embrace analytics and make data-backed decisions. This involves promoting data literacy, providing access to analytics tools and dashboards, and recognizing and rewarding data-driven initiatives.
- Prioritize Data Security and Privacy: Safeguard data by implementing robust security measures and adhering to privacy regulations. Establish data access controls, encryption protocols, and data governance policies to protect sensitive information and maintain customer trust.
- Continuously Evaluate and Improve: Regularly evaluate the impact and effectiveness of your data-driven analytics initiatives. Monitor key performance indicators (KPIs), track outcomes, and assess the value generated. Use these insights to refine your analytics strategies, address gaps, and drive continuous improvement.
- Foster Collaboration: Encourage collaboration and cross-functional teams to leverage the collective expertise of different departments. Foster a collaborative environment where business stakeholders and analytics teams work together to identify opportunities, define requirements, and implement data-driven solutions.
By following these best practices, organizations can effectively embrace data-driven analytics and leverage its transformative potential to drive informed decision-making, optimize operations, and achieve business success.
FAQ's
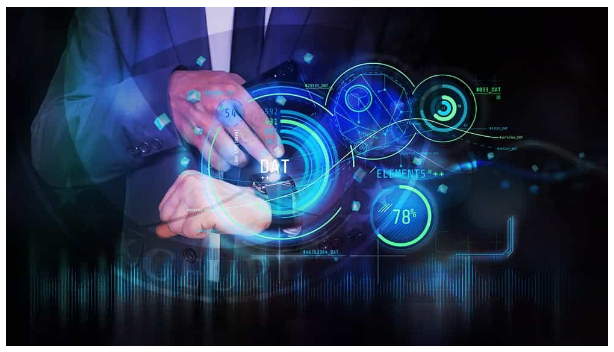
How does data-driven analytics impact decision-making processes in companies?
Data-driven analytics has a significant impact on decision-making processes in companies. Here’s how it influences decision-making:
- Fact-based Decision-Making: Data-driven analytics provides objective and evidence-based insights. Decision-makers can rely on data rather than subjective opinions or assumptions. This promotes fact-based decision-making, reducing the risk of biases and increasing the accuracy of decisions.
- Informed Decision-Making: Data-driven analytics equips decision-makers with valuable information and insights. By analyzing historical and real-time data, organizations comprehensively understand various aspects of their business, including market trends, customer behavior, operational performance, and financial metrics. This informed decision-making ensures choices are based on a solid understanding of the current state of the business.
- Quantitative Insights: Data-driven analytics provides quantitative insights that help decision-makers assess and compare different options. Analyzing data, organizations can quantify the potential outcomes, risks, and benefits associated with each decision. This enables decision-makers to evaluate more precisely and select the most favorable course of action.
- Risk Assessment and Mitigation: Data-driven analytics enables organizations to identify and assess risks more effectively. By analyzing historical data and using predictive modeling techniques, decision-makers can identify potential risks, simulate scenarios, and evaluate the likelihood and impact of different outcomes. This empowers them to develop risk mitigation strategies and make decisions that minimize potential negative consequences.
- Performance Monitoring and Evaluation: Data-driven analytics facilitates continuous monitoring and evaluation of decision outcomes. By setting key performance indicators (KPIs) and tracking relevant metrics, decision-makers can measure the impact and effectiveness of their decisions. This feedback loop helps identify areas for improvement, refine strategies, and make data-backed adjustments to drive better outcomes.
- Agility and Adaptability: Data-driven analytics enhances the agility and adaptability of decision-making processes. By leveraging real-time or near-real-time data analysis, decision-makers can respond quickly to changing market dynamics, customer needs, and emerging trends. This agility allows organizations to make timely adjustments, seize opportunities, and stay ahead of the competition.
- Evidence-based Justification: Data-driven analytics provides decision-makers with evidence-based justification for their choices. By leveraging data insights, decision-makers can present a compelling case supported by quantitative evidence. This strengthens decision-making confidence and facilitates effective communication with stakeholders.
- Continuous Improvement: Data-driven analytics promotes a culture of constant improvement in decision-making. Organizations can collect feedback, analyze outcomes, and refine their decision-making processes based on data insights. This iterative approach fosters learning, innovation, and better decision-making outcomes.
In summary, data-driven analytics significantly impacts decision-making processes in companies by promoting fact-based and informed decisions, facilitating risk assessment and mitigation, enabling performance monitoring and evaluation, enhancing agility and adaptability, providing evidence-based justification, and driving continuous improvement. By leveraging data insights, decision-makers can make more informed, effective, and strategic choices contributing to business success.