Unleashing the Power of Design: Enhancing Data Insights through Analytics UI
Unleashing the Power of Design: Enhancing Data Insights through Analytics UI
In today’s data-driven world, the importance of an intuitive and visually appealing Analytics User Interface (UI) cannot be overstated. By combining the power of design with analytics, businesses can unlock a treasure trove of valuable insights.
Statistics show that a well-designed analytics UI can significantly impact user engagement and decision-making. According to a recent study, websites, and applications with a user-friendly UI witnessed a 200% increase in user interaction compared to those with a poorly designed interface. This highlights the critical role design plays in capturing and retaining user attention.
One way to enhance data insights through analytics UI is by leveraging data visualization techniques. Users can quickly grasp patterns, trends, and correlations by presenting complex data in charts, graphs, and interactive dashboards. For instance, an e-commerce platform that incorporates visually appealing charts to display sales performance can enable business owners to identify top-selling products, customer preferences, and potential market opportunities at a glance.
Furthermore, an optimized analytics UI can empower users to explore data through interactive filters and drill-down capabilities. By allowing users to slice and dice data, they can uncover deeper insights and make data-driven decisions. For instance, a marketing team can refine its targeting strategies by analyzing customer behavior based on various demographic filters.
Businesses can enhance data insights and drive better decision-making by unleashing the power of design and optimizing the analytics UI. Incorporating data visualization techniques and interactive features can transform complex data into actionable insights, leading to improved user engagement and business growth.
Understanding the Importance of User Interface in Analytics
The user interface (UI) in analytics enables users to interact with data and derive valuable insights effectively. A well-designed UI can significantly impact an analytics platform’s usability, efficiency, and overall user experience.
- First and foremost, an intuitive UI simplifies the process of accessing and navigating through data. By presenting information clearly and in the organization, users can quickly locate the needed data, saving time and effort. This streamlined experience enhances productivity, allowing users to focus on analyzing data rather than grappling with complex interfaces.
- Moreover, a user-friendly UI promotes data comprehension. Users can easily interpret and understand complex datasets by incorporating visual elements such as charts, graphs, and data visualization techniques. For instance, a visually appealing dashboard displaying key performance indicators can provide instant insights into business metrics, enabling timely decision-making.
- Additionally, a well-designed UI empowers users to customize their analytics experience. By offering flexible options for data manipulation, filtering, and personalization, users can tailor the interface to meet their specific needs. This level of customization enhances user satisfaction and engagement, as individuals can focus on the data elements that matter most to them.
- Furthermore, an optimized UI promotes collaboration and knowledge sharing among users. By incorporating real-time data updates, annotations, and sharing capabilities, teams can collaborate seamlessly and leverage collective insights. This fosters a data-driven culture within organizations and encourages cross-functional collaboration.
The importance of user interface in analytics must be considered. A well-designed UI enhances usability, promotes data comprehension, enables customization, and fosters collaboration. By investing in a user-friendly analytics UI, businesses can empower their users to make data-driven decisions, derive meaningful insights, and ultimately drive success.
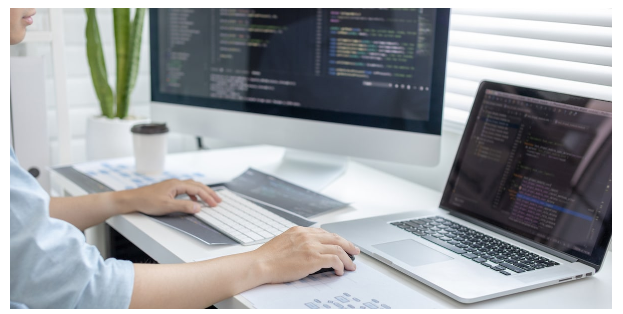
Role of user interface (UI) in Analytics and data insights
User Interface (UI) is crucial in analytics and data insights. It serves as the bridge between users and the complex world of data, enabling effective interaction, exploration, and extraction of valuable insights. A well-designed UI enhances usability, accessibility, and overall user experience, empowering individuals to make informed decisions based on data-driven insights.
- One key aspect of a robust analytics UI is its ability to present data in a clear and organized manner. Users can easily access and comprehend complex datasets through intuitive navigation, well-structured menus, and visually appealing data visualizations. By simplifying the presentation of data, the UI helps users grasp patterns, trends, and correlations, thereby facilitating the discovery of meaningful insights.
- Moreover, an optimized UI empowers users to interact with data flexibly and dynamically. Users can refine and explore data based on their specific needs and objectives by incorporating interactive features such as filters, drill-down capabilities, and customization options. This interactivity enables deeper analysis, promotes data exploration, and facilitates the discovery of actionable insights.
- Another crucial role of UI in analytics is fostering collaboration and knowledge sharing. A well-designed UI facilitates seamless collaboration by allowing users to annotate, share, and discuss insights with colleagues or team members. This collaborative environment promotes a culture of data-driven decision-making, enabling teams to leverage collective expertise and drive better outcomes.
- Furthermore, an effective UI keeps the user at the center of the analytics experience. It considers user preferences, workflow, and context, providing a personalized and tailored experience. By understanding the needs and goals of users, the UI can offer relevant recommendations, anticipate user requirements, and guide them toward valuable insights.
The user interface (UI) is vital in analytics and data insights. It serves as a gateway to navigate the complexities of data, enabling users to interact, analyze, and derive meaningful insights. A well-designed UI enhances usability, promotes interactivity, fosters collaboration, and prioritizes the user’s needs, ultimately empowering individuals and organizations to make informed decisions based on data-driven insights.
How can a well-designed UI enhance user experience and improve data comprehension?
A well-designed User Interface (UI) profoundly impacts user experience and data comprehension. By considering the following factors, a well-designed UI can enhance user experience and improve data comprehension:
- Visual Hierarchy: A well-designed UI establishes a clear visual hierarchy, prioritizing essential elements and guiding users’ attention. Visual cues such as color, size, and placement can highlight crucial data points, enabling users to focus on the most critical information and understand the data context more effectively.
- Consistency and Familiarity: Consistency in UI design elements, such as buttons, icons, and navigation, helps users build familiarity and intuitively understand how to interact with the interface. This consistency creates a seamless experience, reducing cognitive load and allowing users to focus on data comprehension rather than learning how to use the interface.
- Intuitive Navigation: A well-designed UI provides intuitive navigation that allows users to explore and navigate through data easily. By incorporating clear and well-organized menus, search functionalities, and logical information architecture, users can quickly find the needed data, leading to improved comprehension and faster decision-making.
- Data Visualization Techniques: Effective data visualization is critical to improving data comprehension. Well-designed UI leverages various visualization techniques, such as charts, graphs, and interactive dashboards, to present data in a visually appealing and easily understandable format. By representing complex data visually, users can grasp patterns, trends, and relationships more readily, enabling them to derive insights with greater ease.
- Customization Options: A well-designed UI offers customization options allowing users to tailor their analytics experience to their needs. Users can select relevant metrics, apply filters, and personalize the view of data, enabling them to focus on the most critical aspects of their analysis. This customization empowers users to delve deeper into the data, improving comprehension and more relevant insights.
- Responsive Design: With the increasing use of mobile devices, a well-designed UI ensures a responsive experience across different screen sizes and devices. By adapting the UI layout and functionality to different devices, users can access and comprehend data seamlessly, regardless of their platform.
Considering these aspects, a well-designed UI enhances user experience by reducing complexity, improving usability, and providing a visually engaging environment. This, in turn, leads to improved data comprehension as users can easily navigate, explore, and interpret data, enabling them to make informed decisions based on accurate insights.
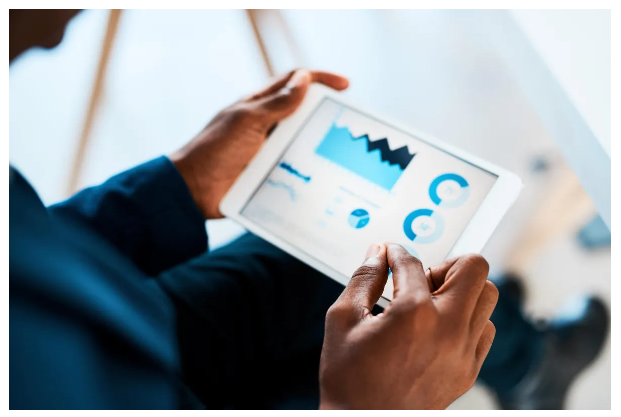
The impact of intuitive UI on user engagement and adoption of analytics tools
Analytics tools’ user interface (UI) drives user engagement and fosters adoption. When an analytics tool has an intuitive UI, it significantly impacts how users interact with the tool and influences their overall experience. Here are some key ways in which an intuitive UI enhances user engagement and drives adoption:
- Ease of Use: An intuitive UI simplifies the learning curve and makes it easier for users to navigate through the analytics tool. With straightforward and intuitive navigation, users can quickly find the needed features, reducing frustration and enhancing their overall experience. When users find an analytics tool easy to use, they are more likely to engage with it regularly and explore its capabilities.
- Quick and Accurate Insights: An intuitive UI enables users to access insights swiftly. Users can quickly comprehend the information and gain valuable insights by presenting data visually appealing and easily digestibly. Intuitive UI elements, such as interactive charts and visualizations, allow users to explore data effortlessly, leading to more accurate and timely decision-making.
- Seamless Data Exploration: A well-designed UI encourages users to explore data further and dive deeper into analytics. With intuitive filtering options, drill-down capabilities, and interactive features, users can navigate through different dimensions of data and uncover valuable insights. This promotes a sense of empowerment and encourages users to engage with the analytics tool more extensively.
- Personalization and Customization: An intuitive UI lets users personalize their analytics experience. Users can customize dashboards, reports, and visualizations to match their needs and preferences. By tailoring the tool to their requirements, users feel a greater sense of ownership and engagement, leading to increased adoption and usage.
- Mobile-Friendly Experience: In today’s mobile-centric world, an intuitive UI optimized for mobile devices is crucial. A responsive and mobile-friendly design ensures that users can access and engage with the analytics tool on the go. This flexibility and accessibility drive user engagement by enabling users to stay connected to their data and insights.
- User Feedback and Iterative Improvements: An intuitive UI encourages user feedback, as users feel more comfortable providing input on their experiences. Analytics tools that actively listen to user feedback and make iterative improvements to the UI based on user suggestions create a positive feedback loop. This fosters collaboration and ownership, driving user engagement and fostering long-term adoption.
An intuitive UI significantly impacts user engagement and adoption of analytics tools. By providing an easy-to-use, visually appealing, and personalized experience, an intuitive UI enhances user satisfaction, drives data exploration, and ultimately leads to increased adoption and usage of analytics tools.
Case studies showcasing successful UI designs in the analytics industry
- Case Study 1: Tableau
Tableau, a leading analytics and visualization platform, has achieved remarkable success with its user-friendly UI design. Its intuitive and visually appealing interface has significantly influenced its widespread adoption. Tableau’s UI allows users to connect to various data sources, drag and drop data fields, and easily create interactive visualizations.
The interface provides a seamless experience for data exploration, enabling users to analyze and derive insights from their data quickly. The intuitive nature of the UI empowers users to build complex reports and dashboards without the need for extensive coding or technical expertise.
The success of Tableau’s UI design is evident in its growing user base and positive user feedback. The platform’s intuitive interface has attracted diverse users, from analysts to business professionals, who appreciate the ease of use and the ability to create compelling visualizations that communicate insights effectively. - Case Study 2: Google Analytics
Google Analytics, a widely used web analytics platform, showcases a successful UI design catering to novice and advanced users. Its user-friendly interface has made it accessible to many users, enabling businesses to leverage data-driven insights for decision-making.
Google Analytics provides a simplified UI that allows users to track website traffic, user behavior, conversions, and more. The interface presents data through interactive charts, graphs, and reports, making it easy for users to understand and interpret key metrics. Users can navigate through different sections effortlessly, access advanced features, and customize their dashboards to focus on specific data points.
The success of Google Analytics’ UI design can be seen in its widespread adoption among website owners, marketers, and businesses of all sizes. Its intuitive interface has made web analytics more accessible, empowering users to make data-informed decisions and optimize their online presence. - Case Study 3: Power BI
Microsoft Power BI is a popular business intelligence and data visualization tool that has gained significant traction due to its powerful yet user-friendly UI design. The platform’s intuitive interface has contributed to its success in enabling users to transform data into meaningful insights.
Power BI offers a drag-and-drop interface that allows users to connect to multiple data sources, create interactive visualizations, and build comprehensive dashboards and reports. The interface provides a seamless experience for data exploration, enabling users to navigate and analyze data quickly. Its extensive pre-built visualizations and customization options enhance the user experience.
The success of Power BI’s UI design can be seen in its growing user base, which includes individuals and organizations across various industries. Its intuitive and visually appealing interface has made data analysis and reporting more accessible, empowering users to make data-driven decisions and share insights within their organizations.
Tableau, Google Analytics, and Power BI case studies highlight successful UI designs in the analytics industry. These platforms have achieved widespread adoption by prioritizing intuitive interfaces that empower users to explore and derive insights from their data effectively. The user-friendly interfaces and powerful analytics capabilities have significantly impacted their success and user satisfaction.
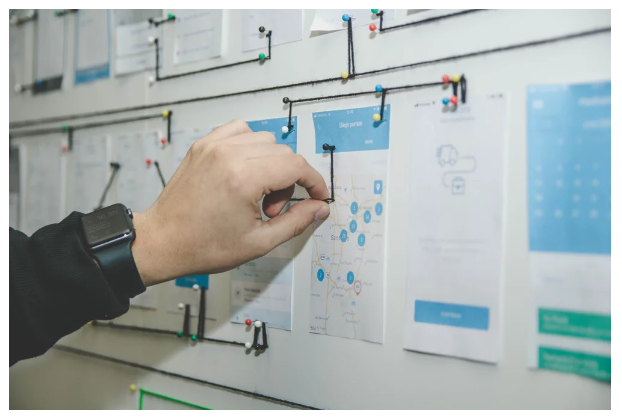
The connection between UI design and decision-making processes
The relationship between UI design and decision-making processes is crucial, as the design of the user interface directly influences how users interact with data and make decisions based on insights. Here are key aspects of this connection:
- Data Accessibility: A well-designed UI ensures that users can easily access the relevant data they need for decision-making. By providing intuitive navigation, search functionalities, and transparent data organization, the UI enables users to locate and retrieve data efficiently. Easy data accessibility reduces the time and effort required to find information, allowing users to make faster decisions.
- Data Visualization and Comprehension: UI design plays a vital role in presenting data in a visually appealing and easily understandable manner. Through effective data visualization techniques such as charts, graphs, and interactive dashboards, the UI helps users comprehend complex data sets, patterns, and relationships. Clear and intuitive visual representations aid in making informed decisions based on data insights.
- Interactivity and Exploration: An intuitive UI encourages users to interact with data, explore different dimensions, and derive more profound insights. By providing interactive features such as filtering, drill-down capabilities, and data exploration tools, the UI enables users to analyze data from various angles and perspectives. This interactivity facilitates a more comprehensive understanding of the data, supporting better decision-making.
- Contextual Information: UI design can provide contextual information at the decision-making point. This includes displaying relevant metrics, KPIs, trends, and contextual data that assist users in making informed choices. By presenting contextual information within the decision-making workflow, the UI guides users toward relevant insights and helps them evaluate options effectively.
- Collaboration and Sharing: UI design can facilitate decision-making and sharing of insights. The UI allows users to collaborate, discuss, and share insights with colleagues or team members by incorporating annotations, comments, and sharing capabilities. This fosters a collaborative decision-making process and ensures that relevant stakeholders share and consider insights.
- User-Friendly Interfaces: A well-designed UI minimizes cognitive load and reduces the learning curve for users. The UI reduces friction in the decision-making process by providing a user-friendly interface that aligns with user expectations. Users can focus on the task rather than struggling to navigate or understand the interface, leading to more effective and efficient decision-making.
UI design significantly influences decision-making processes by enhancing data accessibility, visualization, interactivity, contextual information, and collaboration and providing user-friendly interfaces. A well-designed UI empowers users to make informed decisions based on data insights, leading to better outcomes and driving the success of organizations.
Key Principles for Designing Effective Analytics UI
When designing an effective Analytics User Interface (UI), several key principles can guide the process and ensure a successful outcome. Here are some essential principles to consider:
- User-Centered Design: Place the user at the center of the design process. Understand the users’ goals, tasks, and needs to create a UI that aligns with their expectations and workflows. Conduct user research, gather feedback, and iterate the design based on user insights to ensure a user-centered approach.
- Simplicity and Clarity: Keep the UI design simple and intuitive. Eliminate unnecessary complexity and distractions. Use clear and concise labels, icons, and instructions to guide users through the interface. Avoid clutter and provide a visual hierarchy to prioritize essential elements and make information easily scannable.
- Consistency: Maintain consistency throughout the UI design to create a seamless user experience. Use consistent layouts, color schemes, typography, and interaction patterns across screens and modules. Thickness reduces cognitive load, enhances familiarity, and improves usability.
- Data Visualization: Leverage effective data visualization techniques to present complex data clearly and understandably. Utilize charts, graphs, and visual elements to convey patterns, trends, and relationships. Ensure that visualizations are interactive, allowing users to explore and interact with data for deeper insights.
- Flexibility and Customization: Provide users with flexibility and customization options to tailor the UI to their needs and preferences. Allow users to customize layouts, views, and display options. Offer flexible data filtering, sorting, and grouping capabilities to empower users to analyze data in ways that suit their requirements.
- Responsive Design: Design the UI responsive and adaptable to different devices and screen sizes. Ensure the interface works well on desktops, laptops, tablets, and mobile devices. Consider the varying screen resolutions, touch interfaces, and other device-specific considerations to provide a consistent platform experience.
- Feedback and Error Handling: Offer clear and immediate feedback to users when they interact with the UI. Provide visual cues, notifications, and status updates to inform users about system responses and actions. Handle errors gracefully by providing meaningful error messages and suggestions for resolution.
- Accessibility: Design the UI with accessibility in mind, ensuring that individuals with different abilities and assistive technologies can use it. Follow accessibility guidelines to make the UI perceivable, operable, understandable, and robust for all users.
- Performance Optimization: Optimize the UI’s performance to ensure fast loading times, smooth interactions, and minimal latency. Avoid unnecessary delays, reduce loading times for data visualizations, and optimize data retrieval and processing to provide a seamless and responsive experience.
- Iterative Improvement: Continuously gather user feedback, conduct usability testing, and iterate on the UI design. Embrace an iterative approach to refine and enhance the UI based on user insights and evolving requirements. Regularly assess the UI’s effectiveness and make improvements to address any usability issues or gaps.
By adhering to these key principles, you can design an effective Analytics UI that promotes user engagement, facilitates data comprehension, and empowers users to make informed decisions based on data insights.
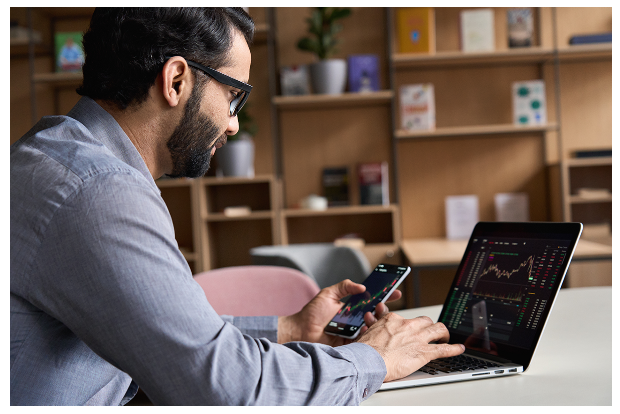
Understanding user needs and goals to inform UI design decisions
Understanding user needs and goals is critical for informing UI design decisions. By gaining insights into users’ requirements, preferences, and objectives, designers can create a user interface that meets their expectations and enhances their overall experience. Here are some key steps to understand user needs and goals:
- User Research: Conduct user research to gather data about the target audience. This can involve techniques such as surveys, interviews, and usability testing. Explore their background, roles, and responsibilities related to analytics. Identify their pain points, challenges, and specific tasks they need to accomplish using the UI.
- Define User Personas: Create user personas based on the research findings. Personas represent typical users and provide a deeper understanding of their characteristics, goals, motivations, and behaviors. Use these personas as a reference throughout the design process to ensure the UI meets their needs.
- Identify Use Cases: Identify the common use cases and scenarios in which users interact with the analytics UI. Consider the specific tasks they must perform, such as data exploration, report generation, or performance tracking. This helps to prioritize features and functionalities that support these use cases.
- Empathy Mapping: Build empathy by creating empathy maps that visualize users’ emotions, thoughts, actions, and pain points during their interactions with the analytics UI. This exercise helps designers better understand users’ mindsets and enables them to design a UI that addresses their concerns effectively.
- User Feedback and Iteration: Continuously gather user feedback throughout the design process. Share prototypes or mockups with users and observe how they interact with the UI. Collect their insights, suggestions, and pain points. Use this feedback to refine and iterate the UI design to better align with user needs and goals.
- Task Analysis: Conduct a task analysis to break down complex tasks into smaller, manageable steps. Understand the sequence of actions users need to take to accomplish their goals. This analysis helps identify opportunities for simplifying the UI and optimizing the user flow to enhance efficiency and effectiveness.
- Contextual Inquiry: Observe users in their natural environment, such as their workplace, to understand how they currently handle analytics tasks. This provides valuable insights into their workflow, tools they currently use, and pain points they encounter. Use these insights to inform UI design decisions that improve their workflow and address their specific needs.
- Collaboration and Stakeholder Input: Involve stakeholders, such as analysts, data scientists, or managers, in the design process. Seek their input and understand their requirements to ensure the UI also caters to their needs. Collaboration with stakeholders helps align the UI design with organizational goals and ensures buy-in from key decision-makers.
By thoroughly understanding user needs and goals, designers can make informed UI design decisions that prioritize usability, address pain points, and empower users to accomplish their tasks effectively. This user-centered approach leads to a UI that resonates with users, enhances their experience, and drives adoption and satisfaction.
Simplifying complex data structures and straightforwardly presenting the information.
Simplifying complex data structures and straightforwardly presenting information is essential for effective data communication. When dealing with complex data, it’s important to make it accessible and easily understandable for your target audience. Here are some strategies to simplify complex data structures and present information straightforwardly:
- Organize and structure the data: Start by organizing the data logically and coherently. Break down complex data into smaller, manageable chunks. Use headings, subheadings, and sections to create a clear structure that guides users through the information.
- Focus on the key message: Identify the key news or main insights you want to convey with the data. Keep your presentation focused on those key points and eliminate unnecessary or extraneous information. By distilling the data to its most essential elements, you can avoid overwhelming your audience with irrelevant details.
- Use clear and concise language: Communicate your message using clear and concise language. Avoid technical jargon or complex terminology that may confuse your audience. Use simple and everyday language that is easily understood by your target audience.
- Visualize the data: Visual representations, such as charts, graphs, and diagrams, can simplify complex data structures and make information more digestible. Choose appropriate visualizations that best represent your data and highlight the key insights. Use labels, legends, and annotations to provide additional context and clarity.
- Utilize storytelling techniques: Frame the data within a narrative or story to make it more relatable and engaging. Connect the data points with real-world examples or scenarios your audience can easily understand. This helps to create a context that enhances comprehension and retention of the information.
- Provide context and explanations: Contextualize the data by providing answers and additional context. Help your audience understand the significance and implications of the data by providing relevant background information or explaining any underlying factors or trends.
- Use data summaries and highlights: Summarize the key findings or insights concisely and visually appealingly. Use bullet points, callouts, or infographics to highlight critical information. This lets your audience quickly grasp the main takeaways without getting lost in the details.
- Iterate and gather feedback: Continuously iterate on your data presentation and gather feedback from your audience. Pay attention to how well they understand the information and where they need clarification. Incorporate their input to simplify further and improve the clarity of your data presentation.
Remember, the goal is to make complex data accessible to your audience without oversimplifying or losing important details. Strive for a balance between simplicity and accuracy, ensuring that your audience can quickly grasp the main points while still capturing the essence of the data.
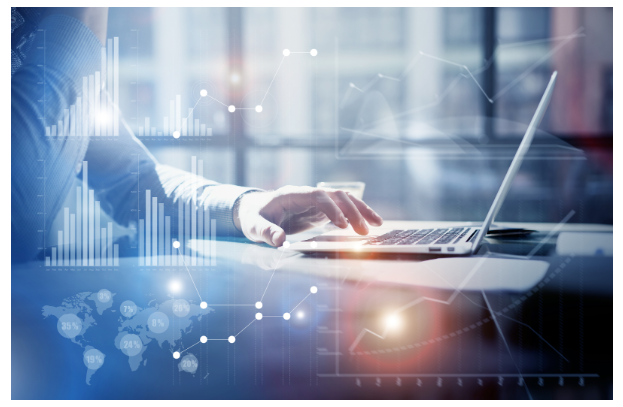
Utilizing visual hierarchy and data grouping techniques for improved data exploration
Visual hierarchy and data grouping techniques are crucial for improving data exploration and analysis. Here are some specific strategies you can implement to enhance your data exploration process:
- Order and emphasize information: Establish a clear visual hierarchy by ordering and emphasizing information based on its importance or relevance. Use larger font sizes, bold or italic styles, and contrasting colors to highlight key data points or headings. This helps users quickly identify and focus on the most critical aspects of the data.
- Organize data into groups: Group related data together to improve understanding and make it easier to identify patterns or trends. Visual cues such as borders, shading, or whitespace distinguish different groups or categories. For example, if you have sales data, you can group products by type or region to facilitate comparisons and analysis.
- Provide clear labels and titles: Ensure that tags and titles are clear, concise, and descriptive. Use meaningful names for categories, axes, and data points to provide users with a clear understanding of what the data represents. Avoid using ambiguous or generic labels that can confuse users.
- Use color effectively: Leverage color to convey information and aid in data exploration. Choose a consistent color palette that is visually appealing and intuitive. Use color to represent different categories or variables, and ensure the color choices are accessible for all users. Be mindful of color blindness and consider using patterns or textures in addition to colors.
- Apply visual cues for relationships: Use cues like lines, arrows, or connectors to illustrate relationships or connections between data points. This helps users understand the dependencies or correlations within the data. For example, you can use lines to connect data points in a network diagram to show relationships between nodes.
- Utilize interactive elements: Incorporate interactive elements to enhance data exploration. Interactive features like filters, sliders, or zooming capabilities enable users to focus on specific subsets of data, adjust parameters, and explore the data from different perspectives. Interactive elements empower users to engage with the data and uncover insights based on their interests or needs.
- Employ appropriate visualizations: Select the most appropriate visualizations to represent your data effectively. Different charts, graphs, or diagrams can reveal different aspects of the data. Choose visualizations that align with the data characteristics and the insights you want to convey. For instance, use bar charts for comparisons, line charts for trends, or scatter plots for correlations.
- Consider responsive and intuitive design: Ensure your data exploration interface is responsive and works well across various devices and screen sizes. Design the interface as intuitive and user-friendly, with clear navigation and controls. Allow users to explore and manipulate the data without feeling overwhelmed or confused.
By implementing these visual hierarchy and data grouping techniques, you can enhance the effectiveness of your data exploration process and enable users to derive meaningful insights from the data. Remember to adapt these techniques to your specific data and user requirements for the best results.