Mastering Revenue Forecasting: Effective Models for Business Planning
Mastering revenue forecasting is crucial for effective business planning. Accurate revenue projections help organizations make informed decisions, allocate resources wisely, and set realistic goals. There are several effective models available for revenue forecasting that businesses can utilize.
One commonly used model is the historical analysis approach. Businesses can identify patterns, seasonal fluctuations, and growth rates by analyzing past revenue trends. This model relies on historical data to project future revenue based on historical performance. For example, a retail company can examine sales data from previous years to anticipate revenue during specific seasons or promotional periods.
Another powerful model is the market research approach. This involves conducting market analysis, studying industry trends, and understanding customer behavior. Businesses can estimate potential revenue opportunities by considering market size, competition, and consumer preferences. For instance, a technology company conducting market research may identify emerging trends and forecast revenue based on the demand for innovative products.
Furthermore, the predictive analytics model leverages advanced statistical techniques and machine learning algorithms. Businesses can generate accurate revenue forecasts by integrating various data sources, such as customer demographics, website traffic, and social media interactions. This model enables companies to adapt quickly to changing market dynamics and optimize their strategies accordingly.
Mastering revenue forecasting is essential for effective business planning. Companies can gain valuable insights and make informed decisions by utilizing models such as historical analysis, market research, and predictive analytics. Combining these models and ongoing evaluation and refinement will contribute to more accurate revenue projections and increased business success.
Importance of Revenue Forecasting in Business Planning
Revenue forecasting is vital in business planning, offering several key advantages for organizations. Here are the reasons why revenue forecasting is important:
- Decision Making: Accurate revenue forecasts provide essential information for making informed decisions. By clearly understanding future revenue potential, businesses can allocate resources effectively, plan marketing strategies, set realistic goals, and make informed investment decisions.
- Resource Allocation: Revenue forecasting helps businesses determine how to allocate their resources efficiently. It enables them to identify high and low revenue periods, allowing for optimized staffing levels, inventory management, and production planning. This helps prevent overstocking or underutilization of resources, minimizing costs and maximizing profitability.
- Financial Planning: Reliable revenue forecasts are crucial for effective financial planning. They enable businesses to anticipate cash flow, plan budgets, and set financial targets. This allows organizations to maintain healthy financial positions, secure funding if necessary, and identify potential gaps or areas that require attention.
- Performance Evaluation: Businesses can evaluate their performance by comparing actual and forecasted revenue. This analysis helps identify strengths, weaknesses, and areas for improvement. It also allows adjustments to strategies and tactics to achieve desired revenue targets.
- Investor Confidence: Accurate revenue forecasts enhance investor confidence in a business. When investors clearly understand the revenue potential, growth prospects, and financial stability, they are more likely to invest in or provide funding to the organization. This can help fuel expansion, innovation, and overall business development.
- Risk Management: Revenue forecasting enables businesses to assess potential risks and develop contingency plans. By understanding revenue patterns, businesses can identify potential threats, such as market fluctuations, changing customer preferences, or competitive challenges. This foresight allows proactive risk management and the ability to adapt strategies accordingly.
Revenue forecasting is essential for effective business planning. It enables informed decision-making, efficient resource allocation, financial planning, performance evaluation, investor confidence, and risk management. By leveraging accurate revenue forecasts, businesses can navigate uncertainties, capitalize on opportunities, and drive sustainable growth.
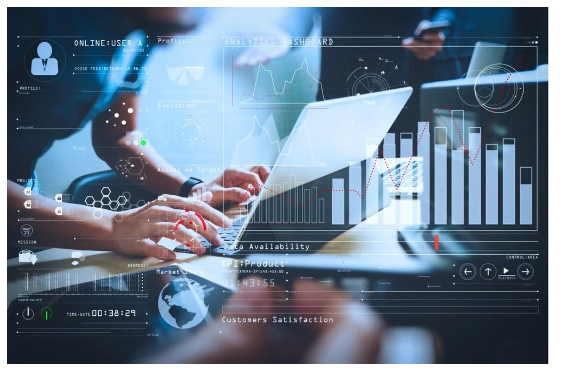
Understanding the role of revenue forecasting in strategic decision-making
Revenue forecasting plays a critical role in strategic decision-making for businesses. Here’s an overview of how revenue forecasting contributes to the process:
- Goal Setting: Revenue forecasts provide a foundation for setting strategic goals. By projecting future revenue levels, businesses can establish realistic targets and align their strategies to achieve them. This ensures that a clear understanding of the revenue potential drives strategic decisions.
- Resource Allocation: Effective revenue forecasting helps businesses allocate resources strategically. It provides insights into anticipated revenue streams, enabling organizations to allocate budgets, manpower, and other resources accordingly. This ensures that resources are optimally utilized to maximize revenue generation and support strategic initiatives.
- Market Assessment: Revenue forecasts require an in-depth analysis of market conditions, customer behavior, and industry trends. This process provides valuable insights into the market landscape, including potential opportunities and challenges. Businesses can leverage this information to make informed decisions regarding market entry, product development, pricing strategies, and target audience selection.
- Risk Management: Revenue forecasting helps identify potential risks and uncertainties that may impact revenue generation. Businesses can develop contingency plans and mitigate risks by assessing various scenarios and considering external factors, such as market volatility or regulatory changes. This allows for proactive decision-making that safeguards revenue and supports long-term strategic objectives.
- Investment Evaluation: Revenue forecasts are instrumental in evaluating potential investments and strategic initiatives. By estimating the revenue impact of different projects or expansion plans, businesses can assess their financial viability and prioritize investments that align with revenue growth objectives. This ensures that resources are allocated to initiatives with the most significant potential to generate revenue and drive overall business success.
- Performance Monitoring: Revenue forecasts serve as a benchmark for evaluating performance and measuring the success of strategic decisions. By comparing actual revenue against projected figures, businesses can identify performance gaps, adjust strategies, and take corrective actions. This continuous monitoring allows for adaptive decision-making and keeps the organization on track toward achieving its strategic objectives.
Revenue forecasting plays a vital role in strategic decision-making. It helps set goals, allocate resources effectively, assess the market landscape, manage risks, evaluate investments, and monitor performance. By incorporating revenue forecasts into decision-making, businesses can make more informed, data-driven strategic choices that drive sustainable growth and competitive advantage.
The impact of accurate revenue forecasting on financial planning and resource allocation
Accurate revenue forecasting significantly impacts financial planning and resource allocation within an organization. Here’s how it influences these crucial aspects:
- Financial Planning: Accurate revenue forecasts provide the foundation for effective financial planning. By projecting future revenue streams, businesses can estimate their expected cash inflows and plan their budgets accordingly. This enables organizations to allocate funds strategically, prioritize expenditures, and optimally allocate financial resources. It also helps identify potential gaps or shortfalls in revenue, allowing proactive measures to be taken to mitigate financial risks.
- Resource Allocation: Accurate revenue forecasting allows businesses to allocate their resources efficiently. With a clear understanding of future revenue expectations, organizations can align their staffing levels, production capacities, and inventory management accordingly. This ensures that resources are utilized optimally and prevents overstaffing or underutilization of assets. It also helps in avoiding unnecessary costs and minimizing waste.
- Investment Decisions: Accurate revenue forecasting is vital in making informed investment decisions. Organizations with reliable revenue projections can assess the financial viability of potential investments or expansion plans. This helps evaluate the expected return on investment and determine the feasibility of allocating resources to specific projects. It enables businesses to prioritize investments that align with revenue growth potential and contribute to the organization’s overall financial health.
- Risk Mitigation: Accurate revenue forecasting aids in identifying potential risks and uncertainties that may impact financial planning and resource allocation. Businesses can develop contingency plans and strategies to mitigate risks by considering various scenarios and analyzing market conditions. This ensures the organization is prepared for unexpected revenue fluctuations or market changes, reducing the potential negative impact on financial stability and resource allocation.
- Performance Evaluation: Accurate revenue forecasting is a benchmark for evaluating performance and measuring the effectiveness of financial planning and resource allocation. By comparing actual revenue against forecasted figures, organizations can assess the accuracy of their projections and identify areas where improvements can be made. This allows for adjustments in financial planning strategies and resource allocation to optimize future outcomes and align them with organizational goals.
Accurate revenue forecasting is crucial for financial planning and resource allocation. It enables organizations to plan budgets, allocate resources efficiently, make informed investment decisions, mitigate risks, and evaluate performance effectively. By incorporating accurate revenue forecasts into these processes, businesses can enhance their financial stability, optimize resource utilization, and drive sustainable growth.
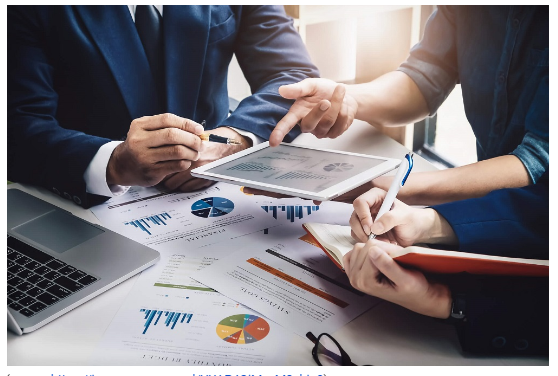
Aligning revenue forecasts with business goals and objectives
Aligning revenue forecasts with business goals and objectives is crucial for the success and growth of any organization. Businesses can make informed decisions and allocate resources effectively by ensuring that revenue projections align with the company’s strategic direction. For example, let’s consider a software company aiming to expand its market share by 20% in the next fiscal year.
The company can estimate the revenue potential of new customer segments or product offerings through data analysis and market research. The company can identify strategies to achieve the desired results by aligning its revenue forecasts with this growth objective. These may include increasing marketing budgets, launching targeted campaigns, or enhancing customer support.
By constantly monitoring and adjusting revenue forecasts based on actual performance, businesses can stay agile and responsive to market conditions, maximizing their chances of reaching their goals and driving sustainable growth.
Essential Revenue Forecasting Models
Regarding revenue forecasting, several essential models can help businesses make accurate projections. These models are based on different factors and can be tailored to suit specific industries and business scenarios. Here are three commonly used revenue forecasting models:
- Historical Growth Model: This model relies on past revenue data to project future growth. It analyzes historical trends, such as revenue growth rates over a specific period, and applies them to future periods. While simple to implement, this model assumes that historical patterns will continue, disregarding market fluctuations and other external factors.
- Market Share Model: Beneficial for competitive industries, the market share model focuses on a company’s share of the total market and projects revenue based on changes in market size and the company’s market share. This model provides insights into revenue growth or decline based on market conditions by considering market dynamics and the potential to gain or lose market share.
- Opportunity-based Model: This model identifies and quantifies potential revenue opportunities. It involves assessing the addressable market, target audience, customer acquisition rates, and pricing strategies to estimate revenue potential. This model provides a more comprehensive view of revenue forecasting by considering various factors that contribute to revenue generation, such as new product launches, geographic expansions, or strategic partnerships.
Remember, selecting the appropriate revenue forecasting model depends on your business’s needs and characteristics. It’s often beneficial to employ a combination of models to gain a more accurate and comprehensive understanding of revenue projections.
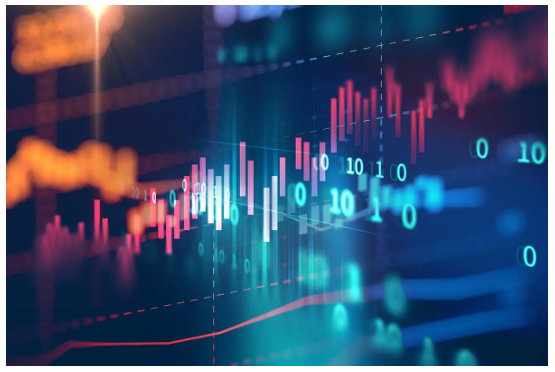
Time Series Analysis: Leveraging historical data and trends for revenue projections
Time series analysis is a powerful technique for leveraging historical data and trends to make revenue projections. By analyzing past revenue data over a specific period, you can identify patterns, seasonality, and other factors influencing revenue fluctuations. Here are some steps to effectively use time series analysis for revenue projections:
- Data Collection: Gather historical revenue data over a consistent time interval. Ensure that the data is accurate, complete, and covers a sufficiently long period to capture relevant trends.
- Data Exploration: Visualize the data to gain insights into its patterns and trends. Plotting the revenue data on a time series chart can help identify seasonality, long-term trends, and outliers.
- Data Preprocessing: Clean the data by removing any outliers or errors that may distort the analysis. Handle missing data points by imputing or interpolating values if possible. Ensure that the time series data is evenly spaced and in a consistent format.
- Decomposition: Decompose the time series into its constituent components, typically trend, seasonality, and residual. This step helps separate the underlying patterns from random fluctuations. Various decomposition methods, such as additive or multiplicative decomposition, can be used depending on the characteristics of the data.
- Modeling: Select an appropriate forecasting model that suits the nature of your revenue data. Standard models include ARIMA (AutoRegressive Integrated Moving Average), exponential smoothing models (such as Holt-Winters), or machine learning algorithms like recurrent neural networks (RNNs) or gradient boosting machines (GBMs). Consider using statistical techniques such as cross-validation to assess the model’s accuracy.
- Model Fitting and Validation: The selected model fits the historical revenue data. Validate the model’s performance by comparing its forecasts with revenue values. Measure the accuracy using metrics like mean absolute error (MAE) or root mean squared error (RMSE).
- Forecasting: Once the model is validated, use it to make revenue projections for future periods. The model can generate forecasts considering seasonal and long-term effects based on the identified patterns and trends. However, it’s important to note that the accuracy of projections decreases as the forecast horizon extends further into the future.
- Sensitivity Analysis: Perform sensitivity analysis by adjusting key variables, such as sales volume, pricing, or market conditions, to understand their impact on revenue projections. This analysis helps assess the robustness of the forecasts and identify potential risks or opportunities.
- Monitoring and Updating: Continuously monitor the actual revenue performance against the projected values. Update the time series model periodically to incorporate new data and improve the accuracy of future revenue projections.
Remember that time series analysis provides a foundation for revenue projections. Still, it’s important to supplement it with domain expertise, market knowledge, and other relevant factors to make informed business decisions.
Regression Analysis: Establishing relationships between revenue and key variables
Regression analysis is a statistical technique used to establish relationships between a dependent variable (such as revenue) and one or more independent variables (also known as predictors or explanatory variables). By analyzing historical data and fitting a regression model, you can quantify the impact of these independent variables on revenue and make projections based on their values.
Here’s how you can use regression analysis to establish relationships between revenue and key variables:
- Identify Key Variables: Determine the key variables that significantly influence revenue. These variables can be advertising expenditure, pricing, marketing campaigns, market size, customer demographics, or other relevant factors affecting income.
- Data Collection: Gather historical data for revenue and the identified key variables. Ensure that the data is accurate, reliable, and covers sufficient time with suitable observations.
- Data Preprocessing: Clean the data by handling missing values, outliers, or errors. Transform the variables if needed, such as converting categorical variables into dummy variables or scaling continuous variables.
- Choose a Regression Model: Select an appropriate regression model based on the nature of the data and the research question. Common regression models include linear regression, multiple regression, polynomial regression, or advanced techniques like ridge regression or Lasso regression. The choice of the model may depend on the data’s assumptions and the relationships’ complexity.
- Model Specification: Specify the functional form of the regression equation based on the selected model. The equation should represent how the independent variables collectively influence the dependent variable (revenue). For example, a simple linear regression equation could be revenue = β0 + β1 * advertising expenditure.
- Model Estimation: Use the collected data to estimate the regression coefficients (β values) through model estimation or model fitting. This involves finding the best-fitting parameters that minimize the differences between the actual revenue and predicted values generated by the regression equation.
- Model Evaluation: Assess the regression model’s goodness of fit and statistical significance. This includes examining metrics like R-squared (coefficient of determination), adjusted R-squared, p-values, and F-statistics. These measures help determine how well the independent variables explain the variation in revenue and whether the model is statistically valid.
- Interpretation: Interpret the estimated regression coefficients to understand the direction and magnitude of the relationships between the independent variables and revenue. Positive coefficients indicate a positive relationship, while negative coefficients indicate a negative relationship. The coefficient’s importance represents the income change associated with a one-unit change in the corresponding independent variable, holding other variables constant.
- Revenue Projections: Once the regression model is validated and deemed appropriate, use it to make revenue projections based on the values of the independent variables. You can estimate the corresponding revenue levels by plugging in different values for the key variables. However, exercise caution when making projections outside the range of the observed data, as extrapolation can be less reliable.
- Model Validation and Updating: Continuously validate the regression model’s performance by comparing the predicted revenue values with the actual revenue data. Update the model periodically as new data becomes available to improve its accuracy and relevance.
Regression analysis allows you to quantify the relationships between revenue and key variables, providing insights into how changes in those variables can impact revenue. However, it’s crucial to consider the limitations of regression analysis, such as potential confounding factors, multicollinearity, and the need for ongoing monitoring and adjustment as market conditions change.
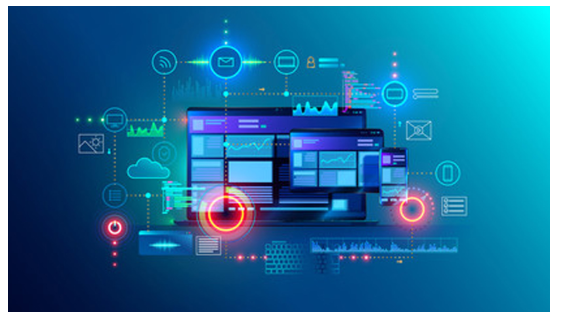
Market-based Models: Incorporating market dynamics and customer behavior in forecasting
Market-based models aim to incorporate market dynamics and customer behavior into revenue forecasting by considering external factors influencing revenue generation. These models go beyond traditional regression and time series analyses by considering market conditions, competitive landscape, customer preferences, and other relevant factors. Here are some approaches to incorporating market dynamics and customer behavior in forecasting:
- Market Segmentation: Divide the market into segments based on customer characteristics, such as demographics, purchasing behavior, or psychographics. Analyze each piece separately to understand their unique needs, preferences, and purchasing patterns. This segmentation allows for targeted forecasting and tailored strategies for each customer segment.
- Customer Lifetime Value (CLV): Calculate the CLV for different customer segments by estimating a customer’s potential revenue over their entire relationship with the company. CLV considers acquisition costs, retention rates, average purchase frequency, and customer churn. Incorporate CLV into forecasting models to understand the revenue impact of customer acquisition, retention, and loyalty strategies.
- Market Share Analysis: Evaluate your company’s market share and relationship with overall market size and growth. Consider factors like competitive positioning, pricing strategies, and market trends. Market share analysis helps forecast how market dynamics and competitive landscape changes can impact your company’s revenue.
- Customer Surveys and Research: Conduct surveys and gather customer feedback to understand their preferences, satisfaction levels, and purchase intentions. Incorporate this data into forecasting models to estimate the revenue impact of changes in customer sentiment, product features, or marketing campaigns.
- Demand Forecasting: Utilize demand forecasting techniques considering market factors like seasonality, macroeconomic indicators, and industry trends. For example, econometric models can be used to estimate the sensitivity of demand to changes in pricing, advertising expenditure, or market conditions.
- Competitive Analysis: Monitor competitors’ activities, market positioning, and pricing strategies. Incorporate this information into forecasting models to assess the potential impact of competitive actions on your company’s revenue. Analyze the market dynamics, such as market growth rates, entry of new competitors, or technological advancements, to understand their influence on revenue projections.
- Scenario Analysis: Conduct a scenario analysis to assess the impact of different market conditions and potential events on revenue. Develop multiple scenarios with varying assumptions, such as changes in customer behavior, economic downturns, regulatory changes, or new market entrants. By simulating these scenarios, you can estimate the range of potential revenue outcomes and make more robust projections.
- Machine Learning and Predictive Analytics: Leverage advanced analytics techniques, such as machine learning algorithms, to analyze large volumes of data and uncover hidden patterns and insights. Predictive models can be developed to forecast revenue based on historical data, market variables, customer behavior, and other relevant features.
- Continuous Monitoring and Feedback Loop: Continuously monitor and evaluate the accuracy of market-based forecasting models against actual revenue performance. Incorporate feedback from the market and update the models regularly to adapt to changing market dynamics, customer behavior, and emerging trends.
Market-based models provide a more holistic approach to revenue forecasting by considering the complexities of the market environment and customer behavior. They enable businesses to make more informed decisions and develop strategies that align with market trends and customer preferences.
Best Practices for Effective Revenue Forecasting
Effective revenue forecasting is crucial for businesses to make informed decisions, allocate resources, and set realistic goals. Here are some best practices to follow for effective revenue forecasting:
- Data Quality and Accuracy: Ensure that the data used for forecasting is accurate, reliable, and of high quality. Remove any outliers, correct errors, and handle missing data appropriately. Validate the data against multiple sources and perform data cleansing and preprocessing as needed.
- Historical Data Analysis: Analyze historical revenue data to identify patterns, trends, and seasonality. Understand the past factors that have influenced revenue, such as marketing campaigns, pricing changes, market conditions, or customer behavior. This analysis provides insights for developing forecasting models and making informed projections.
- Multiple Forecasting Methods: Utilize a combination of forecasting methods to improve accuracy and reliability. Consider using time series analysis, regression analysis, market-based models, or machine learning algorithms. Combining different methods can provide a more comprehensive view of revenue projections and help capture various factors influencing revenue.
- Collaborative Approach: Involve multiple stakeholders and departments in the forecasting process. Seek input from sales, marketing, finance, and operations teams to understand the business landscape better. Collaboration ensures that diverse perspectives and domain expertise are considered, leading to more accurate forecasts.
- Scenario Analysis: Conduct scenario analysis by developing multiple revenue scenarios based on different assumptions and market conditions. Consider best-case, worst-case, and moderate scenarios to understand possible outcomes. This analysis helps assess risks, opportunities, and the potential impact of various factors on revenue.
- Regular Monitoring and Adjustment: Continuously monitor the actual revenue performance against the forecasted values. Regularly update the forecasts based on new data, market changes, and emerging trends. By tracking performance and making adjustments, you can improve the accuracy of future predictions and identify potential deviations early on.
- Sensitivity Analysis: Perform sensitivity analysis to understand the impact of key variables on revenue. Identify the variables with the highest sensitivity and assess their potential risks and opportunities. This analysis helps quantify the effects of changes in factors like pricing, market demand, or customer behavior on revenue projections.
- Communication and Documentation: Communicate the revenue forecasts’ assumptions, methodologies, and limitations. Document the forecasting process and share the findings and insights with relevant stakeholders. Effective communication and documentation foster transparency, accountability, and better decision-making.
- Ongoing Learning and Improvement: Continuously learn from past forecasting experiences and strive for continuous improvement. Evaluate the accuracy of forecasts, assess forecast errors, and identify areas for enhancement. Incorporate feedback, refine forecasting models, and adapt to changing business dynamics to improve future revenue forecasting.
- Review and Revisit Forecasts: Regularly review and revisit revenue forecasts to ensure their relevance and alignment with business objectives. Update the estimates based on changes in market conditions, business strategies, or external factors that impact revenue. Regularly revisiting predictions keeps them current and valid for decision-making.
Remember that revenue forecasting is not an exact science and has inherent uncertainties. By following these best practices, you can improve the accuracy and reliability of revenue forecasts, enabling better planning and decision-making for your business.
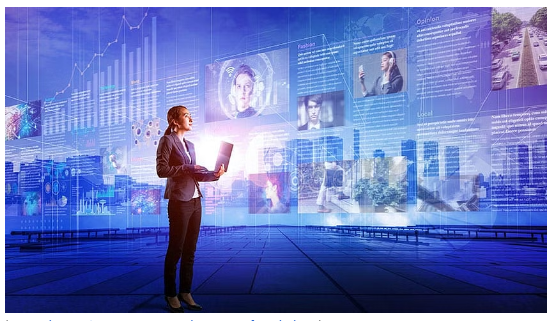
Data-driven Approach: Collecting and analyzing relevant data for accurate forecasts
A data-driven approach is essential for accurate revenue forecasting. By collecting and analyzing relevant data, businesses can uncover insights, identify trends, and make informed decisions. Here are some key steps to take for a data-driven approach to revenue forecasting:
- Define Forecasting Goals: Clearly define the goals and objectives of your revenue forecasting. Determine the questions you want to answer, such as predicting future revenue, understanding the impact of specific variables, or identifying growth opportunities. This clarity will guide your data collection and analysis efforts.
- Identify Relevant Data Sources: The data sources contain the necessary revenue forecasting information. These sources may include historical sales data, customer data, market research reports, industry data, website analytics, social media metrics, or any other data that provides insights into revenue drivers.
- Data Collection: Collect the identified data from various sources. Ensure the data is comprehensive, accurate, and relevant to your forecasting goals. Automate data collection processes whenever possible to ensure consistency and minimize errors.
- Data Cleaning and Preprocessing: Clean the collected data by removing duplicates, correcting errors, and handling missing values. Preprocess the data by transforming variables, aggregating data at appropriate levels (daily, monthly, etc.), and ensuring consistency across different datasets. This step prepares the data for analysis.
- Data Integration: Integrate different datasets that are relevant to revenue forecasting. For example, combine sales data with customer data to analyze the purchasing behavior of different customer segments. The integration enables a more comprehensive analysis and a better understanding of revenue drivers.
- Exploratory Data Analysis: Conduct exploratory data analysis to gain insights into the relationships and patterns within the data. Use visualizations, summary statistics, and statistical techniques to identify trends, outliers, correlations, and other significant patterns that can inform your revenue forecasts.
- Feature Selection: Select the most relevant features (independent variables) from the collected data that significantly impact revenue. Use techniques like correlation analysis, feature importance, or domain expertise to identify the most influential variables in driving revenue.
- Model Development: Develop predictive models based on the selected features and historical revenue data. Utilize appropriate modeling techniques such as regression analysis, time series analysis, machine learning algorithms, or a combination of methods. Train the models using historical data and evaluate their performance using appropriate metrics.
- Model Validation: Validate the performance of the developed models using validation techniques like cross-validation, holdout validation, or out-of-sample testing. Assess the accuracy and robustness of the models and compare their predictions against actual revenue data to ensure they are reliable and accurate.
- Continuous Monitoring and Iterative Improvement: Continuously monitor the performance of the forecasting models and compare the predicted revenue with the actual payment. Identify any discrepancies or deviations and update the models accordingly. Iterate and refine your models to improve accuracy and adapt to changing market conditions.
- Incorporate Feedback and Expert Knowledge: Incorporate feedback from stakeholders, domain experts, and sales teams to enhance the accuracy of the forecasts. They may provide insights, market knowledge, or business intelligence that can be integrated into the forecasting models.
- Stay Agile and Iterative: Revenue forecasting is an ongoing process. As new data becomes available, incorporate it into the forecasting models and update the projections. Be prepared to iterate and refine your models as you gain more insights and improve your understanding of revenue drivers.
A data-driven approach to revenue forecasting ensures that decisions are based on evidence and insights derived from relevant data. It enhances the accuracy and reliability of revenue projections and enables businesses to make more informed and strategic decisions.