Unleashing the Power of Full Stack Analytics: Gaining Comprehensive Insights for Business Success
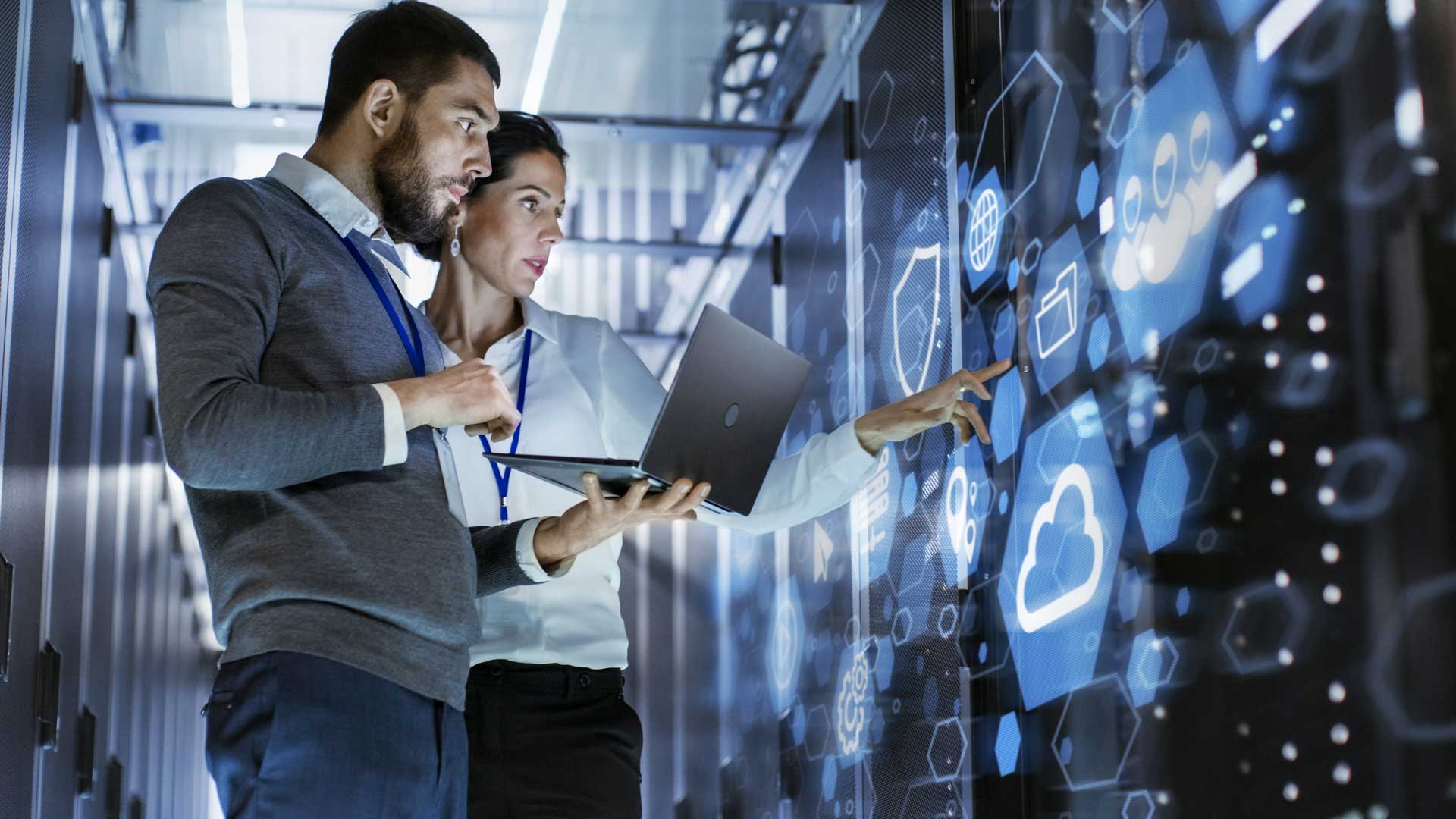
Full-stack analytics is a powerful tool that empowers businesses to gain comprehensive insights for achieving success. Companies can unlock valuable information that drives strategic decision-making by combining data from various sources, including customer interactions, website analytics, and sales data.
For instance, let’s consider e-commerce. Full-stack analytics enables them to analyze customer behavior throughout the customer journey. They can track website traffic, user engagement, and conversion rates to identify bottlenecks and optimize their online experience.
Furthermore, full-stack analytics can provide a holistic view of sales performance. Businesses can identify trends, forecast demand, and optimize their supply chain by integrating data from point-of-sale systems, inventory management, and customer relationship management tools. They can uncover insights such as which products are top sellers, regions have the highest demand, and which customers are most valuable. Furthermore, full-stack analytics can provide a holistic view of sales performance.
With full-stack analytics, businesses can make data-driven decisions that increase efficiency, improve customer satisfaction, and, ultimately, business success. By harnessing the information available, companies can stay ahead of the competition and adapt quickly to changing market dynamics. Embracing full-stack analytics is no longer an option; it’s necessary for businesses striving to thrive in today’s data-driven world.
Understanding Full Stack Analytics
Full stack analytics is a comprehensive approach to data analysis that involves capturing, integrating, and analyzing data from multiple sources across an entire business ecosystem. It allows organizations better to understand their operations, customers, and market dynamics.
With full-stack analytics, businesses can collect data from various touchpoints, such as website interactions, mobile applications, social media platforms, customer relationship management systems, and more. Businesses can uncover valuable insights that drive informed decision-making by aggregating this data into a centralized analytics platform.
One of the critical benefits of full-stack analytics is the ability to track customer behavior across different channels and devices. For example, businesses can analyze customer interactions on their website, follow their engagement with email campaigns, and monitor their activity on social media platforms. By integrating and analyzing these diverse data sets, organizations can gain a holistic view of customer preferences, identify patterns, and personalize their marketing strategies accordingly.
Furthermore, full-stack analytics enables businesses to monitor and optimize their operational processes. Organizations can identify inefficiencies, streamline workflows, and improve overall performance by integrating data from various departments, such as finance, sales, and supply chain. For instance, they can analyze production data to identify bottlenecks, optimize inventory levels based on demand patterns, and forecast sales trends accurately.
Full-stack analytics gives businesses a comprehensive understanding of their operations, customers, and market dynamics. By leveraging data from multiple sources, organizations can make data-driven decisions, enhance customer experiences, and drive business growth.
What is Full Stack Analytics?
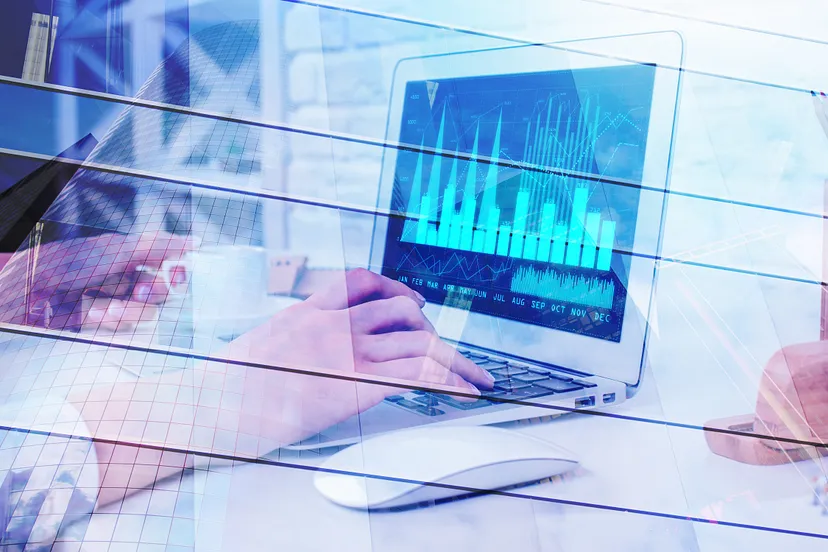
Full stack analytics refers to a comprehensive approach to data analysis that encompasses the entire data ecosystem of an organization. It involves collecting, integrating, and analyzing data from various sources, systems, and touchpoints across different departments and functions.
In traditional analytics approaches, businesses often focus on specific areas or silos of data, such as website analytics or sales data. However, full-stack analytics takes a broader perspective, considering data from multiple layers or “stacks” within an organization. These stacks may include customer data, operational data, financial data, marketing data, and more.
Full-stack analytics provides a more holistic view of business performance by integrating and analyzing data from different stacks. It allows organizations to uncover connections, correlations, and insights that may need to be apparent when analyzing data in isolation. This comprehensive approach helps identify trends, patterns, and opportunities that can drive strategic decision-making and optimize business operations.
Full-stack analytics often involves leveraging advanced analytics techniques, such as data mining, machine learning, and predictive modeling, to extract meaningful insights. It requires robust data infrastructure, including data integration tools, data warehouses, and analytics platforms, to handle the complexity of diverse data sources and enable seamless analysis.
The ultimate goal of full-stack analytics is to empower businesses with comprehensive insights to make informed decisions, improve operational efficiency, enhance customer experiences, and drive business growth. It allows organizations to maximize the value of their data by considering the entire data landscape, from the front-end customer interactions to the back-end operational processes.
The Benefits of Full Stack Analytics
Full Stack Analytics offers several benefits that can help organizations gain valuable insights, make data-driven decisions, and drive business success. Here are some key benefits of adopting Full Stack Analytics:
- Comprehensive Data Insights: Full Stack Analytics enables organizations to harness the power of diverse data sources, including structured, unstructured, and real-time data. Organizations can comprehensively view their operations, customers, and market trends by integrating and analyzing data from various systems, departments, and sources. This broader perspective allows for more accurate and insightful decision-making.
- Improved Data Integration: Full Stack Analytics facilitates data integration from multiple sources and systems. It helps break down data silos and ensures that relevant data is accessible and interconnected, providing a complete understanding of business processes and relationships. Improved data integration reduces duplication, improves data quality, and enables a more unified and consistent analysis.
- Enhanced Agility and Flexibility: Full Stack Analytics enables organizations to be more agile in their data analysis processes. This agility supports rapid decision-making and enables organizations to adapt to changing market conditions and customer needs. It allows for ad-hoc queries, iterative exploration, and faster data processing, empowering data scientists and analysts to uncover insights quickly.
- Advanced Analytics Capabilities: Full Stack Analytics leverages advanced analytics techniques such as predictive analytics, machine learning, and natural language processing. These capabilities enable organizations to go beyond descriptive analytics and gain predictive and prescriptive insights. By leveraging these techniques, organizations can identify patterns, make accurate predictions, optimize processes, and drive proactive decision-making.
- Enhanced Customer Understanding: Full Stack Analytics enables organizations to understand their customers better. This understanding facilitates personalized marketing, targeted recommendations, and improved customer experiences. Organizations can build comprehensive customer profiles by analyzing diverse customer data sources, including transactional data, social media interactions, website behavior, and customer support interactions.
- Real-Time Analytics: Full Stack Analytics supports real-time data processing and analysis, allowing organizations to make decisions based on up-to-date information. Organizations can monitor operational performance, detect anomalies, and respond swiftly to emerging trends or issues. Real-time analytics is particularly valuable in finance, e-commerce, and supply chain management, where timely insights and actions are critical.
- Data-Driven Decision-Making: Full Stack Analytics empowers organizations to make data-driven decisions at all levels. Data-driven decision-making minimizes risks, improves operational efficiency, and increases the likelihood of achieving strategic objectives. By providing comprehensive and accurate insights, organizations can move away from gut feelings or assumptions and base their decisions on solid evidence.
- Improved Operational Efficiency: Full Stack Analytics identifies bottlenecks, inefficiencies, and areas of improvement within business processes. Organizations can uncover opportunities to streamline processes, reduce costs, and optimize resource allocation by analyzing data from various operational systems. This optimization enhances operational efficiency and improves overall performance.
- Competitive Advantage: Full Stack Analytics provides organizations with a competitive edge. Organizations can differentiate themselves in the market by leveraging comprehensive data insights, advanced analytics techniques, and real-time decision-making. They can uncover market trends, identify emerging opportunities, and respond swiftly to customer needs, improving customer satisfaction, increasing market share, and sustaining growth.
- Scalability and Future Readiness: Full Stack Analytics is designed to handle large volumes of data, making it scalable for future growth. It allows organizations to adapt to evolving data sources, technologies, and analytical needs. By embracing Full Stack Analytics, organizations can future-proof their analytics capabilities and ensure they are well-equipped to handle the data challenges of tomorrow.
Full Stack Analytics allows organizations to extract maximum value from their data, gain deeper insights, and drive better business outcomes. It facilitates data-driven decision-making, improves operational efficiency, and helps organizations stay competitive.
Leveraging Full Stack Analytics for Actionable Insights
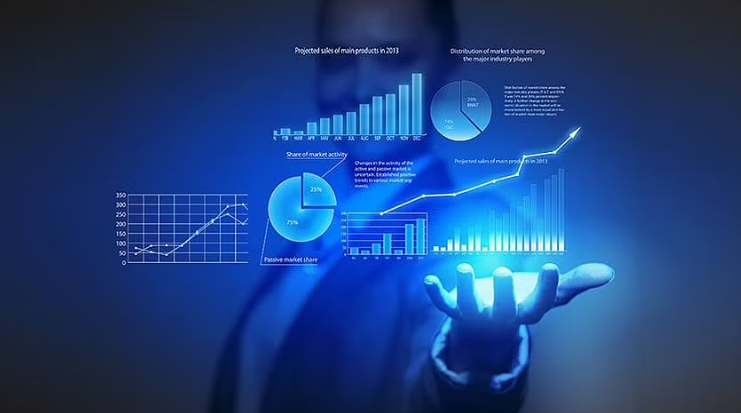
Leveraging Full Stack Analytics can provide organizations with actionable insights that drive meaningful business outcomes. Here are some critical considerations for utilizing Full Stack Analytics to derive actionable insights:
- Define Clear Objectives: Start by clearly defining the objectives and questions you want to address with Full Stack Analytics. What specific business challenges or opportunities do you aim to tackle? By having well-defined goals, you can focus your analytics efforts and ensure the insights generated are relevant and actionable.
- Identify Relevant Data Sources: Determine the relevant data sources to your objectives. This may include structured data from databases, unstructured data from social media or customer feedback, or real-time data from sensors or IoT devices. Assess the quality and availability of these data sources to ensure that you have reliable inputs for your analysis.
- Establish Data Integration and Quality: Ensure that the data from various sources is integrated effectively. Implement data integration techniques, such as data pipelines or ETL (Extract, Transform, Load) processes, to combine disparate data in a unified and consistent format. Also, prioritize data quality initiatives to address issues such as missing values, inconsistencies, or outliers that can impact the accuracy of your insights.
- Leverage Advanced Analytics Techniques: Apply advanced analytics techniques to extract insights from the integrated data. Data mining, machine learning, predictive modeling, or natural language processing can help uncover patterns, correlations, trends, or anomalies that provide actionable insights. Choose the most appropriate techniques based on your objectives and the nature of your data.
- Visualize and Communicate Insights Effectively: Use data visualization techniques to present the insights clearly and intuitively. Visualization tools and dashboards can help stakeholders understand the essential findings and trends. Ensure that the visuals are tailored to the needs of different stakeholders, making it easier for them to interpret the insights and take action based on the information presented.
- Incorporate Context and Business Knowledge: Consider the contextual factors and existing business knowledge when interpreting the insights. The insights generated by Full Stack Analytics should be interpreted in the context of your industry, market dynamics, and specific business operations. This contextual understanding, combined with the insights, allows for more informed decision-making.
- Encourage Collaboration and Cross-Functional Insights: Foster collaboration between different teams and departments to derive comprehensive insights. Encourage cross-functional discussions and brainstorming sessions to gain diverse perspectives and uncover insights that may not be apparent when working in silos. Full Stack Analytics combines data from various sources and functions, enabling a holistic view of the organization.
- Test and Validate Insights: Validate the insights generated through Full Stack Analytics by testing them against real-world scenarios or conducting A/B testing. This validation process helps ensure the accuracy and reliability of the insights before making strategic decisions or implementing changes based on the findings.
- Iterate and Refine: Full Stack Analytics is an iterative process. Continuously monitor the performance and impact of the actions taken based on insights. Measure the outcomes against the objectives and refine your analytics. This iterative approach enables organizations to learn, improve, and adapt their analytical strategies.
- Foster a Data-Driven Culture: To truly leverage Full Stack Analytics for actionable insights, foster a data-driven culture within the organization. A data-driven culture ensures that actionable insights are consistently sought, valued, and acted upon throughout the organization. Encourage stakeholders at all levels to embrace data-driven decision-making, promote data literacy, and provide training and resources to enable employees to leverage analytics tools effectively.
By following these steps and leveraging Full Stack Analytics effectively, organizations can gain actionable insights that drive strategic decision-making, operational improvements, and sustainable business success.
Data Collection and Integration
Data collection and integration are essential processes in data management and analysis. They involve gathering and merging data from various sources to create a unified and comprehensive dataset for further analysis. Here’s a brief overview of data collection and integration:
Data Collection:
Data collection refers to the process of gathering data from diverse sources. This can include various methods such as surveys, interviews, observations, experiments, or collecting data from existing databases or systems. The collected data can be in different formats, such as structured data (organized and well-defined), semi-structured data (partially organized), or unstructured data (lacks a specific organization).
Critical considerations in data collection include:
- Purpose and Scope: Clearly define the purpose and scope of data collection, including the specific variables or attributes to be collected and the target population or data sources.
- Data Sources: Identify the relevant data sources and determine how to access and retrieve data from them. This may involve interacting with databases, APIs, web scraping, or manual data entry.
- Data Quality: Ensure data quality by implementing measures to minimize errors, inconsistencies, or biases in the collected data. This can involve data validation, data cleaning, and data verification techniques.
Data Integration:
Data integration involves combining and merging data from multiple sources into a unified dataset. The goal is to create a consistent and comprehensive data view, enabling practical analysis and decision-making. Data integration can be complex, especially when dealing with disparate data sources and diverse data formats.
Key considerations in data integration include:
- Data Mapping: Identify the common attributes or variables across different datasets and establish mappings between them. This step helps align data elements and ensure accurate integration.
- Data Transformation: Convert and harmonize data formats, structures, and representations to facilitate seamless integration. This may involve standardizing units of measurement, resolving naming inconsistencies, or aggregating data at different levels of granularity.
- Data Cleaning and Deduplication: Detect and resolve inconsistencies, redundancies, or duplications in the integrated dataset. Data cleaning techniques, such as outlier detection, error correction, or record linkage, can be applied to improve data quality.
- Data Governance: Implement data governance practices to ensure data integrity, security, and compliance during integration. This includes establishing data access controls, data privacy measures, and adherence to relevant regulations or policies.
Data collection and integration are iterative processes, and it’s crucial to continually validate, refine, and update the integrated dataset as new data becomes available. The quality and accuracy of the collected and integrated data directly impact the reliability and validity of subsequent data analysis and decision-making processes.
Advanced Analytics Techniques
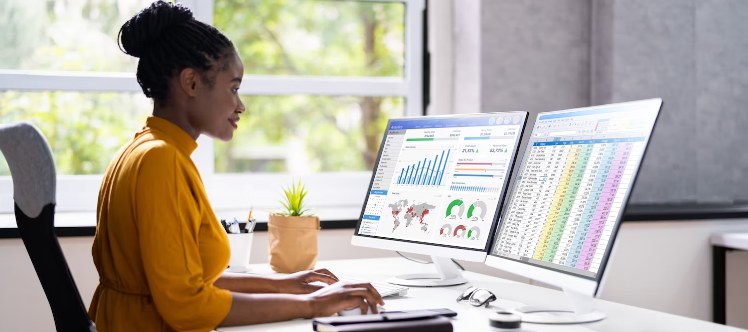
Advanced analytics techniques encompass a range of sophisticated methods and approaches used to analyze complex and large-scale data sets, uncover patterns, make predictions, and generate insights. Here are some critical advanced analytics techniques:
- Machine Learning (ML): Machine learning algorithms enable computers to learn patterns and relationships in data without being explicitly programmed. ML is widely used for tasks like predictive modeling, anomaly detection, recommendation systems, and natural language processing. ML techniques include supervised learning (classification, regression), unsupervised learning (clustering, dimensionality reduction), and reinforcement learning.
- Predictive Analytics: Predictive analytics uses historical data, statistical modeling, and ML algorithms to predict future events or outcomes. It involves identifying patterns and trends in data to forecast customer behavior, demand, sales, or other relevant business metrics. Predictive analytics techniques include regression analysis, time series forecasting, decision trees, and ensemble models like random forests or gradient boosting.
- Text Mining and Natural Language Processing (NLP): Text mining and NLP techniques extract insights from unstructured text data such as customer reviews, social media posts, emails, or documents. NLP involves sentiment analysis, topic modeling, named entity recognition, and text classification. These techniques enable organizations to gain insights from textual data and understand customer opinions, feedback, and trends.
- Cluster Analysis: Cluster analysis is an unsupervised learning technique that groups similar data points based on their characteristics or behavior. It helps identify natural segments or clusters within a dataset, allowing organizations to understand customer segments, market segments, or patterns in data. Standard cluster analysis techniques include k-means, hierarchical, and density-based clustering.
- Neural Networks: Neural networks are a class of ML models inspired by the structure and function of the human brain. Neural networks are used for complex pattern recognition tasks, such as image and speech recognition. They consist of interconnected nodes (neurons) arranged in layers. Deep learning, a subset of neural networks, involves training models with multiple hidden layers to learn hierarchical representations of data.
- Recommendation Systems: Recommendation systems suggest personalized recommendations to users based on their preferences, behaviors, or past interactions. Collaborative filtering, content-based filtering, and hybrid approaches are commonly used in recommendation systems. These techniques are employed in various domains, such as e-commerce, media, and entertainment, to enhance customer experiences and drive sales.
- Time Series Analysis: Time series analysis analyzes data collected over time to understand patterns, trends, and dependencies. It involves techniques like trend analysis, seasonal decomposition, autoregressive integrated moving average (ARIMA) models, and state space models. Time series analysis is valuable for forecasting, demand planning, stock market analysis, and monitoring system performance.
- Social Network Analysis (SNA): Social network analysis examines the relationships and interactions between individuals, organizations, or entities. It helps identify influencers, communities, and patterns of information flow within networks. SNA techniques, such as network centrality measures, community detection, and link prediction, are widely used in social media analysis, marketing, fraud detection, and network optimization.
- Simulation and Optimization: Simulation and optimization techniques involve creating models that mimic real-world systems or processes. These models are then used to simulate different scenarios and optimize decision-making. Monte Carlo simulation, discrete event simulation, linear programming, and genetic algorithms are examples of techniques used for simulation and optimization. They are commonly applied to supply chain optimization, resource allocation, and risk analysis.
- Spatial Analytics: Spatial analytics analyzes geographic or spatial data to gain insights and make informed decisions. It involves techniques like spatial clustering, regression, geospatial interpolation, and network analysis.
Optimizing Business Performance with Full Stack Analytics
Optimizing business performance with Full Stack Analytics involves leveraging comprehensive data insights and advanced analytics techniques to drive improvements across various aspects of the organization. Here are some key strategies to optimize business performance using Full Stack Analytics:
- Define Key Performance Indicators (KPIs): Identify and define the key metrics and performance indicators that align with your business objectives. Clear and measurable KPIs provide a benchmark for performance assessment and help prioritize optimization efforts. These KPIs could be related to revenue, customer satisfaction, operational efficiency, or other critical focus areas.
- Data-Driven Decision-Making: Utilize Full Stack Analytics to support data-driven decision-making across all levels of the organization. Base decisions on insights derived from data analysis rather than relying solely on intuition or anecdotal evidence. This approach ensures that findings are objective, backed by evidence, and likely to lead to positive outcomes.
- Operational Efficiency: Identify areas within your operations where Full Stack Analytics can help improve efficiency. Analyze processes, workflows, and resource allocation to uncover bottlenecks, waste, or inefficiencies. By leveraging insights from Full Stack Analytics, you can streamline operations, reduce costs, and optimize resource utilization.
- Customer Segmentation and Personalization: Use Full Stack Analytics to understand your customer base better. Segment customers based on their characteristics, behaviors, or preferences and develop personalized marketing strategies or product offerings for each segment. By tailoring experiences to individual customer needs, you can enhance customer satisfaction, retention, and loyalty.
- Supply Chain Optimization: Apply Full Stack Analytics to optimize your supply chain operations. Analyze inventory levels, demand patterns, transportation routes, or supplier performance data to identify improvement opportunities. Insights from Full Stack Analytics can help streamline supply chain processes, reduce costs, minimize stockouts, and enhance overall efficiency.
- Predictive Maintenance: Leverage Full Stack Analytics to implement predictive maintenance strategies. Analyze historical maintenance data, sensor data, or equipment performance metrics to predict when maintenance is required or failures are likely to occur. Proactively identifying issues and scheduling maintenance can minimize downtime, reduce repair costs, and improve asset performance.
- Pricing and Revenue Optimization: Utilize Full Stack Analytics to optimize pricing strategies and maximize revenue. Analyze customer behavior, market trends, and competitor data to determine optimal pricing levels, discounts, or promotions. Understanding price elasticity and demand patterns allow you to set prices that maximize profitability and drive revenue growth.
- Risk Management: Apply Full Stack Analytics to identify and mitigate risks within your business operations. Analyze historical data, market trends, or external factors to predict and assess potential threats. By identifying areas of vulnerability, you can develop risk mitigation strategies, implement proactive measures, and improve overall business resilience.
- Marketing and Campaign Optimization: Leverage Full Stack Analytics to optimize marketing campaigns and initiatives. Analyze customer data, campaign performance metrics, and customer engagement patterns to identify the most effective marketing channels, messaging, or targeting strategies. By leveraging insights from Full Stack Analytics, you can optimize marketing spend, improve campaign ROI, and enhance customer acquisition.
- Continuous Improvement: Full Stack Analytics enables continuous improvement by providing real-time or near real-time insights. Use the insights gained to drive ongoing optimizations, iterate on strategies, and make data-driven adjustments to achieve continuous improvement. Establish a feedback loop and regularly monitor performance against KPIs.
By applying Full Stack Analytics to these strategies, organizations can unlock valuable insights, make informed decisions, and optimize business performance across various functional areas, improving profitability, customer satisfaction, and competitive advantage.
Operational Efficiency and Process Improvement
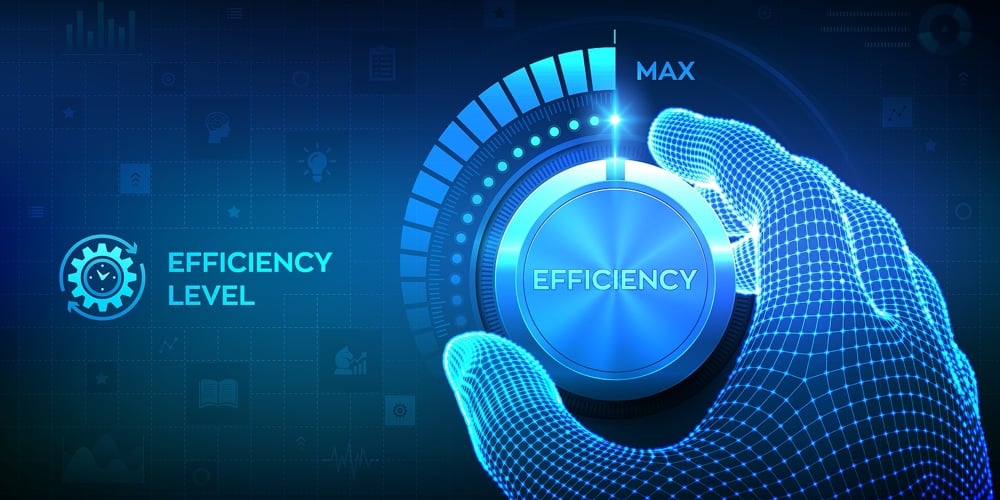
Operational efficiency and process improvement are crucial aspects of any organization’s success. They involve identifying inefficiency or bottlenecks within processes and implementing strategies to streamline operations, reduce waste, and enhance productivity. Here are some key points to consider when focusing on operational efficiency and process improvement:
- Process Analysis: Start by conducting a thorough analysis of your existing processes. Identify each step, input, output, and sequence of activities involved. This analysis will help you understand where inefficiencies or delays are occurring.
- Set Clear Goals: Define specific objectives and goals for process improvement. Clear goals provide direction and help measure progress. These goals include reducing production time, minimizing errors, increasing customer satisfaction, and improving resource allocation.
- Standardization and Documentation: Documenting processes and creating standard operating procedures (SOPs) is essential for consistency and training. SOPs outline step-by-step instructions, responsibilities, and quality standards, ensuring tasks are uniformly and efficiently.
- Identify Bottlenecks: Identify bottlenecks or areas where processes slow down or break down. Various factors, such as resource constraints, inefficient workflows, or outdated technology, can cause these bottlenecks. By identifying and addressing these bottlenecks, you can improve overall process efficiency.
- Automation and Technology: Explore opportunities for automation and the use of technology to streamline processes. Automation can eliminate repetitive manual tasks, reduce errors, and improve speed and accuracy. Consider adopting software solutions, robotic process automation (RPA), or artificial intelligence (AI) tools where applicable.
- Continuous Improvement: Implement a culture of continuous improvement within your organization. Encourage employees to contribute ideas and suggestions for process enhancement. Regularly review processes, gather feedback, and adjust to drive ongoing efficiency gains.
- Data-Driven Decision Making: Leverage data and analytics to make informed decisions. Data can provide valuable insights into areas that need attention and inform optimization efforts. Use Key Performance Indicators (KPIs) to measure process performance, identify trends, and track improvement over time.
- Employee Engagement and Training: Engage employees in process improvement initiatives. Involve them in identifying challenges and finding solutions. Offer training and development programs to enhance their skills and knowledge, empowering them to contribute to operational efficiency improvements.
- Lean and Six Sigma Methodologies: Lean and Six Sigma methodologies provide structured approaches for process improvement. Lean focuses on eliminating waste and non-value-added activities, while Six Sigma aims to reduce process variation and defects. Consider incorporating these methodologies into your improvement efforts.
- Collaboration and Communication: Foster a culture of collaboration and open communication across teams and departments. Encourage cross-functional collaboration to identify interdependencies and areas for improvement that span multiple processes. Effective communication ensures everyone understands process improvement initiatives’ goals, roles, and responsibilities.
Remember, operational efficiency and process improvement are ongoing endeavors. Regularly monitor and reassess processes to adapt to changing circumstances and maintain a competitive edge in your industry.
Customer Experience and Personalization
Customer experience and personalization are critical to building strong customer relationships and driving business growth. By delivering exceptional experiences and tailoring interactions to individual customer preferences, organizations can differentiate themselves in the market and foster customer loyalty. Here are some key points to consider when prioritizing customer experience and personalization:
- Customer Journey Mapping: Gain a deep understanding of your customer’s journey by mapping out their interactions and touchpoints with your organization. Identify pain points, moments of delight, and opportunities for personalization throughout the customer lifecycle.
- Customer Segmentation: Divide your customer base into segments based on common characteristics, needs, or behaviors. This segmentation allows you to tailor your messaging, offerings, and experiences to specific customer groups, enhancing relevance and personalization.
- Personalized Communication: Leverage customer data and insights to deliver customized communication across various channels. Address customers by name, offer relevant recommendations based on their preferences, and provide targeted offers or promotions that align with their interests.
- Omni-Channel Experience: Ensure a seamless experience across multiple channels, such as in-store, online, mobile, and social media. Customers expect consistent and personalized interactions regardless of their chosen track, so invest in integrating and aligning these channels to provide a unified experience.
- Proactive Customer Service: Anticipate customer needs and address them proactively. Use data analytics to identify potential issues or opportunities and reach customers before encountering problems. Proactive customer service demonstrates care, builds trust, and enhances the overall experience.
- Personalized Product Recommendations: Utilize customer data and past purchase behavior to offer personalized product recommendations. Implement recommendation engines or algorithms that suggest relevant products or services based on customers’ preferences, browsing history, or purchase patterns.
- Feedback and Listening: Actively seek customer feedback through surveys, social media monitoring, or customer support interactions. Listen to their concerns, suggestions, and preferences, and continuously use this feedback to improve your products, services, and experiences.
- Empower Frontline Employees: Equip frontline employees with the tools, training, and autonomy to deliver personalized experiences. Encourage them to build customer relationships, understand their needs, and go the extra mile to create memorable interactions.
- Seamless Self-Service Options: Provide self-service options that are intuitive and user-friendly. Empower customers to find answers, make changes, or resolve issues through well-designed websites, knowledge bases, chatbots, or interactive voice response (IVR) systems.
- Measure and Optimize: Continuously measure and analyze customer experience metrics, such as Net Promoter Score (NPS), customer satisfaction (CSAT), or customer effort score (CES). Use these insights to identify areas for improvement and prioritize initiatives that will have the most significant impact on customer satisfaction and loyalty.
Remember, customer experience and personalization should be an ongoing focus as customer expectations and preferences evolve. Regularly review and adapt your strategies to meet changing customer needs and deliver exceptional experiences that foster long-term customer loyalty.
FAQs:
What is the difference between Full Stack Analytics and traditional analytics approaches?
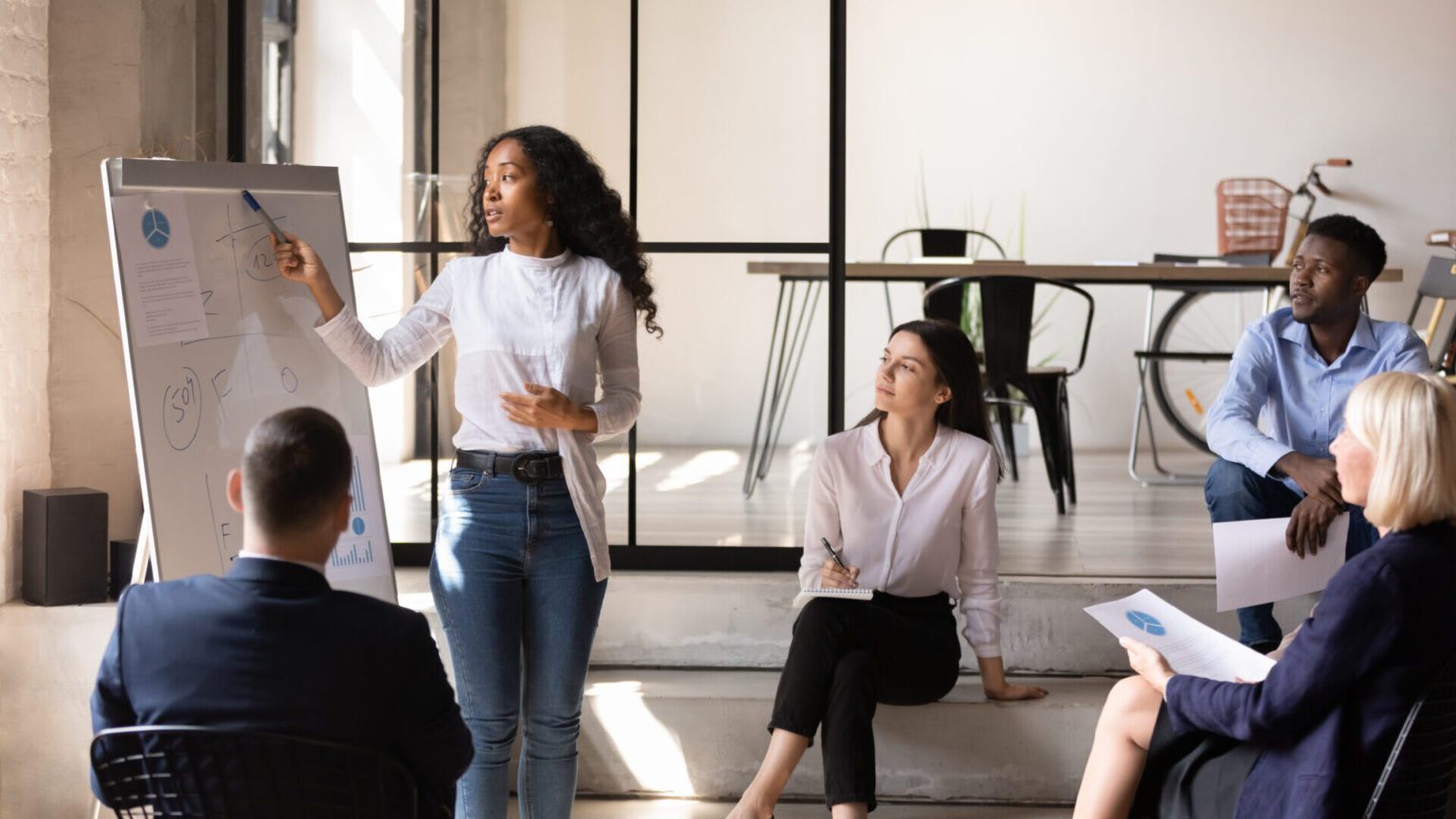
Full Stack Analytics and traditional analytics approaches differ in scope, methodology, and level of integration across different data sources and technology platforms. Here are some critical distinctions between the two:
- Scope and Integration: Full Stack Analytics encompasses a comprehensive view of an organization’s data, including data from various sources such as structured databases, unstructured data, external data feeds, and real-time streaming data. It integrates data from different systems, departments and heads into a unified analytics environment. Traditional analytics approaches, on the other hand, often focus on specific data sets or limited sources of data within a particular department or function.
- Data Collection and Processing: Full Stack Analytics emphasizes collecting and processing large volumes of diverse data types. It often involves technologies like data lakes, big data platforms, or cloud-based data warehouses to handle the storage and processing of massive data sets. Traditional analytics approaches rely on smaller, structured data sets stored in relational databases or data warehouses.
- Technology Stack: Full Stack Analytics leverages various technologies and tools to manage the entire analytics workflow, including data collection, storage, processing, visualization, and reporting. Traditional analytics approaches often focus on tools or platforms tailored to a specific function or department. This may include technologies like Hadoop, Spark, NoSQL databases, data integration platforms, advanced analytics tools, and interactive data visualization tools.
- Agility and Flexibility: Full Stack Analytics enables organizations to be more agile and flexible in exploring and analyzing data. It allows data scientists and analysts to access and integrate diverse data sources, perform ad-hoc analyses, and iterate quickly to discover insights. Traditional analytics approaches are often more rigid and structured, with predefined reports or dashboards designed for specific business needs.
- Advanced Analytics Capabilities: Full Stack Analytics often incorporates advanced techniques like predictive analytics, machine learning, and natural language processing. These techniques enable organizations to derive meaningful insights from complex and unstructured data. Traditional analytics approaches typically focus on descriptive analytics, summarizing historical data to provide insights into past performance.
- Business Impact and Decision-Making: Full Stack Analytics aims to provide organizations with a holistic view of their data, enabling data-driven decision-making at all levels. It emphasizes leveraging data to drive strategic initiatives, optimize operations, and improve customer experiences. Traditional analytics approaches often focus on department-specific metrics and reports, primarily used for operational monitoring and decision-making within a specific function.
Full Stack Analytics can be seen as an evolution or extension of traditional analytics approaches. While conventional analytics approaches are still valuable in specific contexts, Full Stack Analytics provides a more comprehensive and integrated approach to handling diverse data sets, enabling organizations to unlock deeper insights and make more informed decisions across the entire business landscape.