Streamlining Data-Driven Decision-Making: Business Analytics Standard Operating Procedures (SOP)
Streamlining data-driven decision-making is crucial for businesses in today’s competitive landscape. Implementing well-defined Standard Operating Procedures (SOPs) for business analytics can significantly enhance the effectiveness and efficiency of this process.
By following SOPs, organizations establish a consistent framework for data collection, analysis, and interpretation. This ensures that decision-makers have access to accurate and reliable information. For instance, a retail company can develop an SOP that outlines the specific metrics to track, such as sales revenue, customer acquisition costs, and conversion rates. These SOPs provide a standardized approach for data gathering across different departments and enable comparisons and trend analysis.
Furthermore, SOPs help prioritize data sources and define key performance indicators (KPIs) aligned with business objectives. For example, an e-commerce platform may identify website traffic, bounce rates, and average order value as critical KPIs. SOPs ensure that these metrics are consistently monitored, allowing businesses to identify trends, detect anomalies, and make data-driven decisions promptly.
Implementing SOPs also promotes accountability and transparency within an organization. By clearly defining roles and responsibilities for data collection, analysis, and reporting, SOPs reduce ambiguity and potential errors. This ensures that decision-makers have confidence in the presented data and fosters a culture of trust and collaboration.
In summary, having well-defined SOPs for business analytics is essential for streamlining data-driven decision-making. It provides a consistent framework, enables the identification of relevant metrics, and enhances accountability and transparency. By implementing SOPs, businesses can leverage data effectively and make informed decisions that drive growth and success.
Introduction to Business Analytics SOP
Standard Operating Procedures (SOPs) are vital in streamlining business analytics processes, ensuring consistency, and facilitating data-driven decision-making. By establishing guidelines and protocols, SOPs provide a structured approach to collecting, analyzing, and interpreting data within an organization.
A Business Analytics SOP aims to define the step-by-step procedures for various data handling and analysis activities. This includes data collection methods, data cleaning and preprocessing techniques, statistical analysis methodologies, and reporting guidelines. By documenting these procedures, organizations create a reference point for employees involved in analytics processes, ensuring uniformity and accuracy.
A well-designed SOP in business analytics promotes efficiency and minimizes errors. It provides clear instructions on data sources, extraction techniques, and validation procedures. For example, an SOP may specify using specific software tools or programming languages for data analysis, ensuring consistency across different analytical projects.
Moreover, an SOP helps standardize key performance indicators (KPIs) and metrics used for decision-making. It establishes the criteria for measuring success and aligns analytics efforts with organizational goals. By defining the KPIs and their calculation methodologies, an SOP ensures that everyone involved in the analytics process understands the tracked objectives and metrics.
Additionally, a Business Analytics SOP enhances data governance and security. It outlines data protection measures, data access controls, and data storage protocols to ensure compliance with privacy regulations and safeguard sensitive information.
A Business Analytics SOP is a comprehensive guide for conducting analytics activities within an organization. It promotes consistency, efficiency, and accuracy in data handling, analysis, and reporting. By adhering to the guidelines outlined in the SOP, organizations can make informed decisions based on reliable data, leading to improved business outcomes.
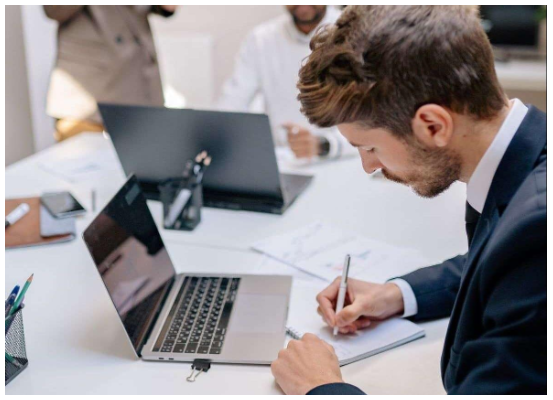
Explanation of Standard Operating Procedures (SOP) in the Context of business analytics
Standard Operating Procedures (SOPs) in business analytics are detailed guidelines and protocols that outline the specific processes and steps to be followed when conducting analytical tasks within an organization. SOPs provide a standardized data collection, analysis, interpretation, and reporting framework, ensuring consistency and reliability in the analytics workflow.
One of the key purposes of SOPs in business analytics is to establish a structured approach to data handling. This includes specifying the sources from which data should be collected, the methods of data extraction, and the procedures for data cleaning, transformation, and preprocessing. SOPs ensure that data is collected uniformly, eliminating potential inconsistencies and errors that could impact the accuracy of analytical insights.
Furthermore, SOPs define the statistical analysis methodologies for the analytical process. This involves outlining the appropriate statistical techniques, algorithms, and models for data exploration, hypothesis testing, regression analysis, predictive modeling, or other analytical tasks. SOPs help ensure that data is analyzed consistently and objectively, enhancing the reliability of the findings and recommendations.
In addition to data handling and analysis, SOPs also address the reporting and visualization of analytical results. They specify the format, structure, and content of reports or dashboards, ensuring that the insights derived from analytics are effectively communicated to stakeholders. SOPs may define key performance indicators (KPIs) to be included in reports and provide guidelines for visual representations such as charts, graphs, and tables.
SOPs in business analytics also promote data governance and security. They establish guidelines for data access controls, data protection measures, and data storage protocols, ensuring compliance with privacy regulations and safeguarding sensitive information. SOPs help maintain data integrity, confidentiality, and the ethical use of data within the organization.
SOPs in the context of business analytics provide a systematic approach to data handling, analysis, and reporting. They ensure consistency, reliability, and compliance in the analytics workflow, promoting effective decision-making based on accurate and meaningful insights. By following SOPs, organizations can optimize their analytical processes and maximize the value derived from data.
Importance of establishing SOPs for data-driven decision-making
Organizations must establish Standard Operating Procedures (SOPs) for data-driven decision-making. Here are some key reasons why SOPs are crucial in this context:
- Consistency and Accuracy: SOPs ensure a consistent and standardized data collection, analysis, and interpretation approach. By defining specific procedures and guidelines, SOPs minimize variations and errors in decision-making. This consistency leads to more reliable and accurate insights, fostering confidence in the data-driven decisions made.
- Efficiency and Time Savings: SOPs streamline the workflow by providing clear instructions and best practices for handling data. They eliminate the need for reinventing the wheel with each new decision-making task. By following established SOPs, teams can work more efficiently, saving time and effort that would otherwise be spent on figuring out the process from scratch.
- Quality Assurance: SOPs help maintain the quality of data used in decision-making. They outline data validation techniques, cleaning procedures, and quality checks to ensure that only reliable and relevant data is utilized. This reduces the risk of erroneous conclusions or flawed decisions based on inaccurate or incomplete data.
- Scalability and Standardization: SOPs facilitate scalability by enabling organizations to replicate successful data-driven decision-making processes across various teams, departments, or locations. They serve as a reference point for training new employees and ensuring a consistent approach to analytics throughout the organization. Standardization through SOPs ensures that everyone is on the same page when handling data and making decisions.
- Compliance and Governance: SOPs are crucial in ensuring compliance with legal and ethical standards, such as data privacy regulations. They establish data protection, confidentiality, and proper data usage guidelines, ensuring that organizations operate within legal boundaries. SOPs also promote data governance by defining roles, responsibilities, and access controls, ensuring data is handled responsibly and securely.
- Continuous Improvement: SOPs provide a foundation for continuous improvement in data-driven decision-making. By documenting processes and outcomes, organizations can analyze the effectiveness of their methodologies and identify areas for optimization. SOPs can be periodically reviewed, updated, and refined to incorporate new techniques, technologies, or feedback, driving continuous enhancement of the decision-making process.
SOPs are essential for data-driven decision-making as they ensure consistency, accuracy, efficiency, quality, scalability, compliance, and continuous improvement. By establishing SOPs, organizations can enhance their decision-making capabilities and effectively leverage data power.
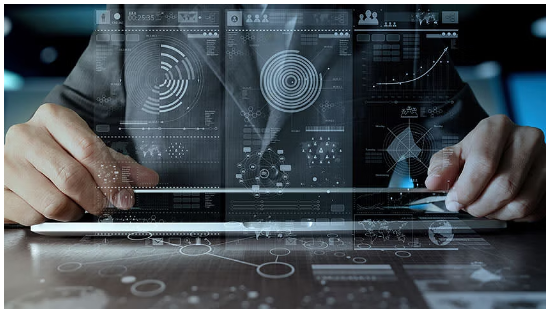
Benefits of implementing standardized processes in business analytics
Implementing standardized processes in business analytics offers several benefits for organizations. Here are some key advantages:
- Consistency and Replicability: Standardized processes ensure consistency in how data is collected, analyzed, and interpreted across different projects, teams, and departments. This consistency allows for better comparability of results and promotes reliable decision-making. Standardized processes also enable replicating successful analytics approaches, making it easier to scale analytical efforts throughout the organization.
- Efficiency and Time Savings: Standardized processes streamline the analytics workflow by providing predefined steps and guidelines. This reduces the time and effort spent on figuring out the process from scratch for each new project. With standardized processes, teams can work more efficiently, focusing on analyzing data and deriving insights rather than reinventing the process repeatedly.
- Improved Accuracy and Quality: Standardized processes help maintain the accuracy and quality of analytical outputs. Organizations ensure that best practices are followed consistently by specifying data collection methods, data cleaning techniques, and analytical methodologies. This reduces errors and biases in data analysis, leading to more reliable and trustworthy insights.
- Enhanced Collaboration and Communication: Standardized processes facilitate collaboration among team members and stakeholders involved in the analytics process. When everyone follows the same set of processes and procedures, it becomes easier to communicate and share knowledge. Standardization promotes a common understanding of analytical methodologies, simplifying discussing and exchanging insights and findings.
- Scalability and Agility: Standardized processes enable organizations to scale their analytics efforts effectively. As the volume and complexity of data grow, standardized methods provide a framework that can be applied consistently across various datasets and business scenarios. Standardization also enhances agility by allowing teams to adapt quickly to new projects or changing requirements while still adhering to the established processes.
- Compliance and Risk Management: Implementing standardized processes helps organizations adhere to regulatory requirements and manage data handling and analysis risks. By incorporating privacy and security measures into standardized processes, organizations can ensure compliance with relevant regulations and safeguard sensitive information effectively.
- Continuous Improvement: Standardized processes provide a foundation for continuous improvement in business analytics. By documenting and analyzing the results and outcomes of standardized processes, organizations can identify areas for optimization, refine methodologies, and incorporate new techniques or technologies. This iterative approach promotes the ongoing enhancement of analytical capabilities and outcomes.
Implementing standardized processes in business analytics brings consistency, efficiency, accuracy, collaboration, scalability, compliance, and continuous improvement. These benefits enable organizations to derive more value from their data, make informed decisions, and gain a competitive edge in today’s data-driven business environment.
Defining and Documenting Analytics Processes
Defining and documenting analytics processes is crucial in establishing a structured and efficient approach to data analysis. Here’s a guide on how to define and document analytics processes effectively:
- Identify the Scope: Determine the scope of your analytics processes. This involves understanding the specific objectives, stakeholders, and data sources involved. Consider the analytics tasks, such as descriptive, diagnostic, predictive, or prescriptive analytics.
- Map the Workflow: Visualize and map out the workflow of your analytics processes. Identify the steps involved, from data collection to analysis to reporting. Consider the dependencies between different stages and the roles and responsibilities of team members at each step.
- Define Data Collection Procedures: Clearly define the procedures for data collection, including the sources, methods, and frequency of data collection. Specify the data variables to be captured, ensuring they align with the analytics objectives. Document any data preprocessing steps, such as cleaning, transformation, and integration.
- Outline Analytical Techniques: Specify the analytical techniques and methodologies to be applied. This may include statistical analysis, data mining, machine learning algorithms, or visualization techniques. Provide guidelines on selecting the appropriate techniques based on the nature of the data and the analytics goals.
- Document Analysis Steps: Define the steps involved in the analysis process. This may include exploratory data analysis, hypothesis testing, model development, validation, and evaluation. Document the specific procedures, parameters, and algorithms used at each stage.
- Establish Reporting Guidelines: Define the format, structure, and content of analytical reports or dashboards. Specify the key metrics, visualizations, and insights to be included. Consider the target audience and their information needs. Document any data visualization, storytelling, or interpretation guidelines to ensure effective communication of the findings.
- Incorporate Quality Assurance Measures: Include quality assurance procedures in the documentation. This may involve data validation checks, cross-validation techniques, or benchmarking against external sources. Document any steps taken to ensure data accuracy, reliability, and integrity.
- Define Documentation Standards: Establish clear standards for documenting the analytics processes. Ensure consistency in terminology, format, and style across the documentation. Consider using flowcharts, diagrams, and supporting documentation to enhance understanding.
- Review and Update: Regularly review and update the documentation as needed. Analytics processes evolve, so it’s essential to incorporate new techniques, technologies, or feedback. Solicit input from stakeholders and subject matter experts to ensure the documentation remains up-to-date and relevant.
- Communicate and Train: Communicate the documented analytics processes to relevant stakeholders. Provide training and resources to team members involved in the analytics process. Foster a culture of adherence to the documented processes to ensure consistency and alignment.
By following these steps, you can effectively define and document analytics processes, promoting consistency, efficiency, and accuracy in your data analysis efforts.
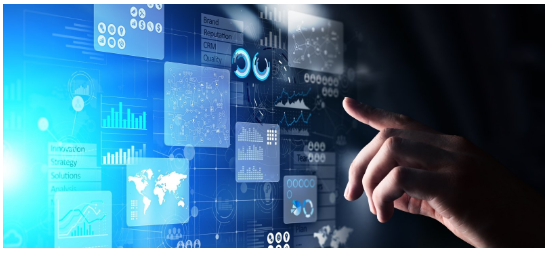
Identifying key business analytics processes that require SOPs
Several key processes benefit from having well-defined Standard Operating Procedures (SOPs) in business analytics. Here are some essential analytics processes that often require SOPs:
- Data Collection: SOPs for data collection outline the procedures, methods, and tools used to gather relevant data. This includes specifying data sources, extraction techniques, and validation protocols. SOPs ensure consistency in data collection practices, enabling reliable and accurate analysis.
- Data Cleaning and Preprocessing: SOPs for data cleaning and preprocessing establish guidelines for handling data quality issues, missing values, outliers, and inconsistencies. They define data cleaning, transformation, and normalization steps to ensure the data is suitable for analysis.
- Data Analysis and Modeling: SOPs for data analysis and modeling outline the analytical techniques, algorithms, and models used to derive data insights. They define the steps and parameters for performing statistical analysis, data mining, machine learning, or other modeling techniques. SOPs ensure consistency in applying these methodologies across different projects or analyses.
- Reporting and Visualization: SOPs for reporting and visualization guide the creation of reports, dashboards, and visual representations of analytical insights. They specify the format, structure, and content of reports, including key performance indicators (KPIs), metrics, and visualizations to be included. SOPs ensure clear and effective communication of analytical findings.
- Quality Assurance and Validation: SOPs for quality assurance and validation establish procedures for verifying analytical results’ accuracy, reliability, and integrity. They include checks for data accuracy, model validation techniques, and cross-validation procedures. SOPs ensure that the analytical outcomes meet predefined quality standards.
- Decision-Making and Actionable Insights: SOPs for decision-making and actionable insights provide guidelines for translating analytical findings into actionable recommendations. They outline the process for identifying insights, evaluating their impact, and supporting decision-making. SOPs ensure that the analytics outputs are effectively utilized for driving business decisions.
- Data Governance and Security: SOPs for data governance and security address the protection, access control, and privacy of data used in analytics. They define data storage, backup, and retention procedures and guidelines for data access and usage. SOPs ensure compliance with data protection regulations and mitigate data security risks.
It’s important to note that the specific analytics processes requiring SOPs may vary based on the organization’s industry, objectives, and analytical maturity. SOPs should be tailored to address the unique needs and requirements of the business and its analytics initiatives.
Creating clear and detailed guidelines for each process
Creating clear and detailed guidelines for each business analytics process is essential for ensuring consistency and accuracy in data analysis. Here’s a breakdown of how to establish guidelines for some key analytics processes:
Data Collection Guidelines:
- Specify the data sources, such as databases, APIs, or external sources, along with the relevant access procedures.
- Define the methods and tools for data extraction, including SQL queries, web scraping, or data integration techniques.
- Document data validation procedures, including completeness, accuracy, and consistency checks.
- Outline any data privacy or ethical considerations, ensuring compliance with relevant regulations.
Data Cleaning and Preprocessing Guidelines:
- Document the steps for handling missing values, outliers, and inconsistent data.
- Define procedures for data cleaning, such as removing duplicates, standardizing formats, or imputing missing values.
- Specify data transformation techniques, such as scaling, log transformations, or normalization. d. Establish guidelines for handling data quality issues, including data profiling and outlier detection methodologies.
Data Analysis and Modeling Guidelines:
- Specify the analytical techniques and algorithms suitable for the given objectives.
- Document the steps for exploratory data analysis, descriptive statistics, and visualization techniques.
- Define the process for selecting and validating predictive models, such as cross-validation or train-test splits.
- Outline any assumptions or requirements for specific analytical methods to ensure consistent application.
Reporting and Visualization Guidelines:
- Define the structure and format of analytical reports or dashboards.
- Specify the key metrics and performance indicators to be included in the reports.
- Provide guidelines for data visualization, ensuring clear and effective communication of insights. d. Outline any standards for report documentation, including version control or naming conventions.
Quality Assurance and Validation Guidelines:
- Establish procedures for assessing the accuracy and reliability of data and analytical results.
- Define metrics or benchmarks for evaluating the quality of models or predictions.
- Document validation techniques like cross-validation, hypothesis testing, or benchmarking against external sources.
- Specify the frequency or criteria for reviewing and validating the analytical processes to ensure ongoing quality assurance.
Decision-Making and Actionable Insights Guidelines:
- Guide how to translate analytical findings into actionable recommendations.
- Document the process for evaluating the impact and feasibility of recommendations.
- Establish criteria or decision rules for prioritizing and implementing insights.
- Specify any supporting documentation or contextual information required for decision-making.
Data Governance and Security Guidelines:
- Define data access controls, including user roles, permissions, and authentication protocols.
- Establish guidelines for data storage, backup, retention, and disposal.
- Document procedures for protecting sensitive data, including encryption or anonymization techniques.
- Specify compliance measures for data privacy regulations and security best practices.
By creating clear and detailed guidelines for each process, organizations can ensure consistency, accuracy, and efficiency in their analytics efforts while promoting a standardized approach to data analysis.
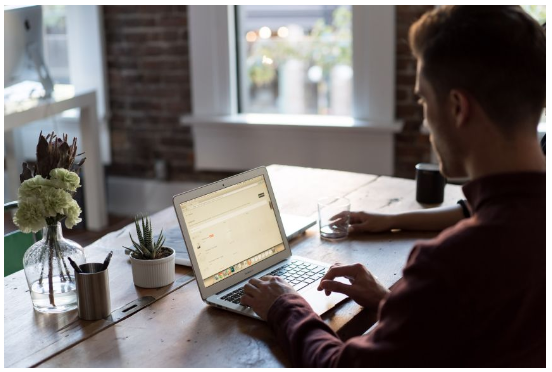
Documenting step-by-step procedures and workflows
When documenting step-by-step procedures and workflows for business analytics processes, it’s important to provide clear and concise instructions that enable others to replicate the process effectively. Here’s a guide on how to document these procedures:
- Start with an Overview: Begin by providing an overview of the process or workflow. Describe its purpose, objectives, and the desired outcomes.
- Break Down the Steps: Divide the process into sequential steps. Number or label each step to make it easy to follow. Use headings or subheadings to group related steps together.
- Provide Detailed Instructions: For each step, provide clear and detailed instructions on what needs to be done. Use text, visuals (such as screenshots or diagrams), and examples to enhance understanding. Be specific about the tools, software, or programming languages required.
- Include Key Considerations or Tips: Highlight any crucial considerations or tips that can help improve the efficiency or effectiveness of the process. For example, mention common challenges or pitfalls to avoid, best practices, or shortcuts that can save time.
- Address Decision Points: If there are decision points within the process where different options or paths can be chosen, clearly outline the criteria or factors to consider for making those decisions. Guide how to proceed based on specific circumstances or requirements.
- Document Inputs and Outputs: Clearly state the inputs required for each step and the expected outputs or deliverables. This helps ensure that the process is executed correctly and that the results are consistent.
- Include References and Resources: Provide references to any relevant documentation, data sources, or external resources that can support the execution of the process. This could include links to specific websites, research papers, or internal documentation.
- Review and Validate: Before finalizing the documentation, review the step-by-step procedures and workflows to ensure accuracy, clarity, and completeness. Consider seeking feedback or validation from subject matter experts or other stakeholders involved.
- Format and Structure: Logically organize the documentation. Use headings, subheadings, bullet points, or numbering to make it easy to navigate and comprehend. Consider using a consistent template or format to maintain uniformity across different documentation.
- Keep it Updated: As processes evolve or new techniques and technologies emerge, make sure to update the documentation accordingly. Periodically review and revise the procedures to ensure they remain relevant and aligned with current practices.
Following these guidelines, you can effectively document step-by-step procedures and workflows for business analytics processes. Clear and detailed documentation enables efficient knowledge transfer, promotes consistency, and facilitates the effective execution of analytics tasks.
Ensuring consistency and repeatability in analytics activities
Ensuring consistency and repeatability in analytics activities is essential for reliable and accurate results. Here are some key practices to maintain consistency and repeatability:
- Standardize Processes: Establish standardized processes and workflows for analytics activities. Clearly define the steps, methodologies, and tools to be used. This ensures that everyone follows the same procedures, minimizing variations and inconsistencies.
- Document Standard Operating Procedures (SOPs): Document detailed SOPs for each analytics activity, including data collection, preprocessing, analysis, and reporting. Clearly outline the specific guidelines, parameters, and best practices to be followed. SOPs serve as a reference for team members, ensuring a consistent approach to analytics.
- Define Metrics and Key Performance Indicators (KPIs): Clearly define the metrics and KPIs to be tracked and measured during analytics activities. Establish consistent definitions and calculation methodologies for these metrics. This ensures that the same measurements are used consistently across different projects and analyses.
- Implement Data Quality Assurance Measures: Develop data quality assurance protocols to ensure consistency and accuracy in data used for analysis. This includes data validation, cleansing, and standardization procedures. Maintaining high-quality data minimizes discrepancies and errors that could impact analytical outcomes.
- Utilize Version Control: Implement version control mechanisms for analytical models, scripts, and code. This enables tracking changes and ensures that the same version is used consistently across different analyses. Version control also facilitates collaboration and reproducibility.
- Maintain Data Governance and Security: Implement robust data governance and security measures to ensure data access, privacy, and protection consistency. Define data access controls, permissions, and protocols. Adhere to data privacy regulations and establish secure data storage and handling practices.
- Conduct Peer Reviews and Validation: Foster a culture of peer reviews and validation to ensure consistency and quality in analytics activities. Have team members review each other’s work, provide feedback, and identify potential issues. This helps catch errors, promotes learning, and maintains consistency.
- Establish Documentation and Knowledge Management: Document and organize analytical processes, methodologies, and findings in a centralized knowledge management system. This facilitates knowledge sharing, enables easy access to previous work, and promotes consistency in decision-making.
- Provide Training and Continuous Learning: Offer training programs to enhance the skills and knowledge of team members involved in analytics activities. This ensures that everyone understands the established processes and methodologies, promoting consistent execution. Encourage continuous learning and staying updated with the latest trends and techniques in analytics.
- Regularly Monitor and Evaluate: Continuously monitor and evaluate the performance and outcomes of analytics activities. Use feedback and metrics to identify areas for improvement and refinement. Regularly review and update SOPs to incorporate lessons learned and optimize processes.
By following these practices, organizations can maintain consistency and repeatability in their analytics activities. Consistent processes and methodologies lead to reliable and comparable results, enabling informed decision-making based on accurate and trustworthy insights.
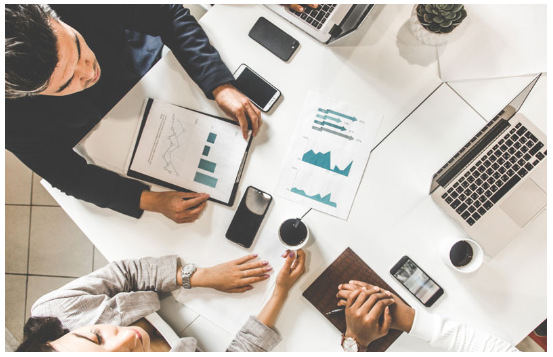
Implementing Quality Control and Assurance Measures
Implementing quality control and assurance measures is crucial for maintaining analytics activities’ accuracy, reliability, and integrity. Here are key steps to implement quality control and assurance measures in business analytics:
- Data Quality Assessment: Conduct a thorough data quality assessment before analyzing. Define criteria for data completeness, accuracy, consistency, and relevance. Implement data profiling techniques to identify potential issues or anomalies in the data. Address any data quality issues through cleansing, standardization, or enrichment processes.
- Validation and Cross-Verification: Validate the accuracy and reliability of analytical models, algorithms, and calculations. Cross-verify results using different techniques or data subsets to ensure consistency. Employ cross-validation, holdout testing, or benchmarking against known standards or external sources.
- Documentation and Version Control: Maintain comprehensive documentation of analytical processes, including data sources, preprocessing steps, modeling techniques, and decision-making criteria. Implement version control mechanisms to track changes and ensure that analytical models, code, and scripts are well-documented, properly versioned, and auditable.
- Peer Reviews and Validation: Foster a culture of peer reviews and validation. Encourage team members to review each other’s work, provide feedback, and identify potential errors or biases. This promotes learning, and knowledge sharing and improves the overall quality of analytics outputs.
- Sensitivity Analysis and Robustness Checks: Conduct sensitivity analysis to assess the impact of varying assumptions, parameters, or data inputs on the analytical results. Perform robustness checks to ensure the stability and reliability of the models or algorithms under different scenarios or datasets.
- Regular Audits and Quality Checks: Implement regular audits and quality checks of the analytics processes and outputs. This includes reviewing data sources, methodologies, calculations, and reporting. Identify and rectify any deviations or discrepancies from established standards or procedures.
- Compliance with Regulations and Ethics: Ensure compliance with relevant regulations, industry standards, and ethical guidelines in data handling, privacy, and security. Adhere to data protection laws and maintain the confidentiality and integrity of sensitive information throughout the analytics process.
- Continuous Learning and Improvement: Foster a culture of continuous learning and improvement. Stay updated with the latest advancements, techniques, and best practices in analytics. Encourage professional development and provide training opportunities to enhance skills and knowledge within the team.
- Stakeholder Feedback and Validation: Seek feedback from stakeholders involved in decision-making based on analytics outputs. Validate the analytics results’ relevance, accuracy, and usefulness with domain experts, managers, or end-users. Incorporate their feedback and suggestions to refine and improve the analytics processes and outputs.
- Ongoing Monitoring and Evaluation: Continuously monitor and evaluate the performance and impact of analytics activities. Track key metrics and indicators to assess the effectiveness and value of the analytics outputs. Use feedback loops to ensure ongoing improvement and optimize the implemented quality control and assurance measures.
By implementing these quality control and assurance measures, organizations can ensure their analytics activities’ accuracy, reliability, and integrity. This fosters trust in the data-driven decision-making process and facilitates better business outcomes based on sound analytical insights.