End-to-end analytics is a data analysis approach that provides a complete view of the data, allowing businesses to understand their operations and make data-driven decisions to optimize performance. This guide will give you a step-by-step overview of the end-to-end analytics process and explain how it can be used to gain holistic data insights.
- Identify Key Data Sources: The first step in the end-to-end analytics process is identifying the critical data sources relevant to the business process. This may include website traffic, social media engagement, sales data, and customer feedback.
- Integrate and Organize Data: The next step is to integrate and organize the data from the identified sources into a single source of truth. This involves ensuring that the data is accurate, consistent, and up-to-date.
- Analyze Data: Once the data is organized, the next step is to analyze it using various data analysis techniques, including data mining, predictive modeling, and machine learning. This involves uncovering patterns and trends in the data to gain insights into customer behavior, preferences, and needs.
- Use Insights to Optimize Performance: The insights gained from end-to-end analytics can be used to optimize every stage of the business process. For example, businesses can use the insights to personalize their marketing messages, improve their website navigation, optimize their product offerings, and identify areas for improvement in their operations.
- Monitor and Evaluate Performance: The final step in the end-to-end analytics process is to monitor and evaluate the performance of the optimized business process. This involves tracking key performance indicators (KPIs) and adjusting to ensure continued success.
End-to-end analytics is a powerful tool for businesses that want to make data-driven decisions and stay competitive in today’s market. By leveraging this approach, companies can comprehensively understand their data, optimize their operations, and drive growth and profitability.
What is End-to-End Analytics?
End-to-end analytics is a data analysis approach that involves analyzing every stage of a business process, from data capture to decision-making. It is a holistic approach that provides a complete view of the data, allowing businesses to understand their operations and make data-driven decisions to optimize performance.
The end-to-end analytics process typically involves identifying the critical data sources relevant to the business process, integrating and organizing the data into a single source of truth, analyzing the data using various data analysis techniques, and using the insights gained to optimize the business process.
The insights gained from end-to-end analytics can be used to personalize marketing messages, improve website navigation, optimize product offerings, and identify areas for improvement in business operations.
End-to-end analytics differs from traditional data analysis methods in that it takes a more comprehensive approach by analyzing every stage of the business process. It also involves working with structured and unstructured data sets and using various data analysis techniques, including data mining, predictive modeling, and machine learning.
End-to-end analytics is a powerful tool for businesses that want to make informed decisions and stay competitive in today’s market. By leveraging this approach, companies can better understand their data, optimize operations, and drive growth and profitability.
An introduction to end-to-end analytics and its role in providing comprehensive data insights
End-to-end analytics is an approach to data analysis that involves capturing, analyzing, and using data at every stage of a business process. End-to-end analytics aims to provide a complete view of the data, allowing businesses to understand their operations and make data-driven decisions to optimize performance.
End-to-end analytics involves identifying the critical data sources relevant to the business process, integrating and organizing the data into a single source of truth, and analyzing the data to gain insights. The insights gained from end-to-end analytics can be used to optimize every stage of the business process, from planning and execution to monitoring and evaluation.
One of the critical benefits of end-to-end analytics is that it provides a more holistic view of the data, allowing businesses to uncover patterns and trends that may not be apparent when looking at individual data sets.
By gaining a comprehensive understanding of their data, businesses can make more informed decisions, identify areas for improvement, and optimize their operations to drive growth and profitability.
End-to-end analytics is a powerful tool for businesses that want to make data-driven decisions and stay competitive in today’s market. By leveraging this approach, companies can gain a deeper understanding of their data, optimize their operations, and drive success in their industry.
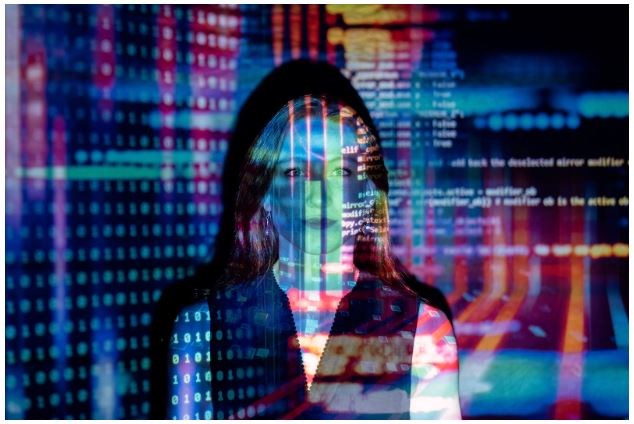
How end-to-end analytics differs from traditional data analysis methods?
End-to-end analytics differs from traditional data analysis methods in several ways. While conventional data analysis methods focus on analyzing individual data sets or processes, end-to-end analytics takes a more comprehensive approach by analyzing every stage of a business process.
Traditional data analysis methods often involve working with structured data sets and using statistical techniques to uncover patterns and trends. On the other hand, end-to-end analytics consists of working with both structured and unstructured data sets and using various data analysis techniques, including data mining, predictive modeling, and machine learning.
Another critical difference between end-to-end analytics and traditional data analysis methods is the focus on business outcomes. End-to-end analytics is designed to provide insights that can be used to optimize business processes and drive growth and profitability. In contrast, traditional data analysis methods often focus on understanding data for their own sake.
Finally, end-to-end analytics is more collaborative than traditional data analysis methods. It involves working with organizational stakeholders to identify critical data sources, develops analytical models, and implement data-driven solutions. Traditional data analysis methods, on the other hand, are often siloed within specific departments or teams.
End-to-end analytics provides a more holistic and business-focused approach to data analysis than traditional methods. By taking a comprehensive view of the data and working collaboratively across the organization, businesses can leverage end-to-end analytics to gain valuable insights and drive success in their industry.
The benefits of end-to-end analytics for business decision-making and strategy development
End-to-end analytics offers several benefits for businesses when it comes to making data-driven decisions and developing effective strategies. Here are some of the critical benefits of end-to-end analytics:
- A comprehensive view of data: End-to-end analytics provides a complete picture of the data, allowing businesses to understand their operations and make informed decisions based on insights from every stage of the business process.
- Improved decision-making: By gaining a deeper understanding of their data, businesses can make more informed decisions grounded in data and analytics rather than relying on intuition or assumptions.
- Increased efficiency: End-to-end analytics can help businesses identify areas for improvement in their operations, resulting in increased efficiency and cost savings.
- Competitive advantage: By leveraging end-to-end analytics to gain insights into customer behavior, preferences, and needs, businesses can stay ahead of the competition by providing better products and services that meet the evolving needs of their customers.
- Improved customer experience: End-to-end analytics can help businesses personalize their marketing messages, improve their website navigation, and optimize their product offerings to provide a better customer experience.
- Better risk management: By analyzing data from every stage of the business process, businesses can identify potential risks and take steps to mitigate them before they become significant issues.
End-to-end analytics can help businesses make more informed decisions, improve efficiency, gain a competitive advantage, and provide a better customer experience. By leveraging the insights hidden within their data, businesses can drive growth and success in their industry.
Critical Components of End-to-End Analytics
End-to-end analytics involves a comprehensive approach to data analysis that includes multiple components. Here are the critical elements of end-to-end analytics:
- Data Capture: The first step in end-to-end analytics is to capture data from various sources, including customer interactions, sales transactions, website visits, and social media engagement.
- Data Integration: The next step is to integrate the data from various sources into a single source of truth. This involves ensuring that the data is accurate, consistent, and up-to-date.
- Data Analysis: Once the data is integrated, the next step is to analyze it using various techniques, including data mining, predictive modeling, and machine learning. This helps uncover patterns and trends in the data, providing valuable insights into customer behavior and preferences.
- Visualization: Data visualization is an important component of end-to-end analytics that helps businesses make sense of the data. It involves presenting the data in visual formats such as graphs, charts, and dashboards, making it easier to understand and interpret.
- Reporting: The insights gained from end-to-end analytics must be communicated effectively across the organization. Reporting involves summarizing the findings clearly and concisely, highlighting the key insights and actionable recommendations.
- Implementation: The final component of end-to-end analytics is the implementation of data-driven solutions. This involves using the insights gained from the analysis to optimize business processes, personalize marketing messages, improve website navigation, optimize product offerings, and identify areas for improvement in business operations.
End-to-end analytics involves multiple components that work together to provide a comprehensive approach to data analysis. By leveraging the insights hidden within their data, businesses can drive growth, optimize operations, and stay ahead of the competition.
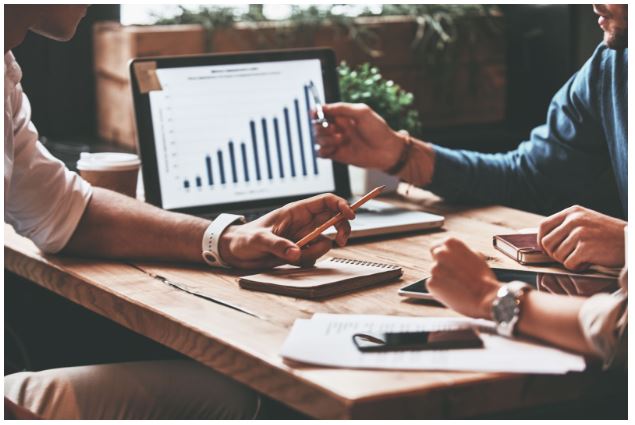
Defining the data lifecycle and key data touchpoints
The data lifecycle refers to the stages that data goes through, from its creation or captures to its disposal or archiving. The data lifecycle typically includes the following steps:
- Data Creation: This is the stage where data is first created or captured. Individuals may make it manually, automatically by machines or sensors, or generated through customer interactions.
- Data Storage: Once data is created, it must be stored securely and organized to ensure its availability for future use. Data can be kept on-premise or in the cloud, and various storage solutions are available to businesses, such as databases, data warehouses, and data lakes.
- Data Processing: This stage involves transforming the raw data into a usable format that can be analyzed. Data processing may include data cleaning, data integration, and data enrichment.
- Data Analysis: Once data is processed, it can be analyzed using various techniques such as statistical analysis, data mining, and machine learning. Data analysis aims to uncover patterns and trends in the data that can provide valuable insights for decision-making.
- Data Visualization: Data visualization involves presenting the insights gained from data analysis in a clear and understandable format, such as charts, graphs, and dashboards.
- Data Utilization: The final stage of the data lifecycle involves using the insights gained from data analysis to drive business decisions, improve operations, and optimize performance.
Key data touchpoints are critical points where data is captured or exchanged within a business process. Some examples of important data touchpoints include:
- Customer Interactions: This includes customer interactions such as sales calls, customer support tickets, and social media engagement.
- Sales Transactions: This includes data related to customer purchases, such as order details, payment information, and shipping information.
- Web Analytics: This includes data related to website traffic, such as page views, bounce rates, and click-through rates.
- Operational Data: This includes data related to business operations, such as inventory levels, production rates, and supply chain performance.
- Financial Data: This includes data related to financial performance, such as revenue, expenses, and profit margins.
Understanding the data lifecycle and key data touchpoints is essential for businesses that want to leverage data-driven insights to optimize their operations, drive growth, and stay ahead of the competition.
Identifying relevant data sources, such as customer interactions and operational data
Identifying relevant data sources is a critical step in implementing end-to-end analytics. Here are some of the key data sources that businesses should consider:
- Customer Interactions: Data related to customer interactions is a valuable source of information that can provide insights into customer behavior and preferences. This data may include customer demographics, purchase history, and feedback.
- Operational Data: Data related to business operations, such as inventory levels, production rates, and supply chain performance, can provide valuable insights into areas for improvement and optimization.
- Sales Transactions: Data related to sales transactions, such as order details, payment information, and shipping information, can provide insights into customer preferences and buying behavior.
- Web Analytics: Data related to website traffic, such as page views, bounce rates, and click-through rates, can provide insights into customer behavior and preferences, as well as the effectiveness of marketing campaigns.
- Social Media Engagement: Data related to social media engagement, such as likes, shares, and comments, can provide insights into customer sentiment and preferences.
- Financial Data: Data related to financial performance, such as revenue, expenses, and profit margins, can provide insights into the overall health of the business and areas for improvement.
Identifying relevant data sources is critical for businesses leveraging end-to-end analytics to gain valuable insights and optimize their operations. By working with stakeholders from across the organization, companies can identify the essential data sources relevant to their business processes and leverage the insights hidden within their data to drive success in their industry.
Best practices for integrating and analyzing different types of data
Integrating and analyzing different data types can be challenging, as it often involves working with data structured differently or stored in other formats. Here are some best practices for integrating and analyzing different types of data:
- Identify Key Data Elements: The first step in integrating different data types is identifying the key elements relevant to the business process. This involves working with stakeholders from across the organization to identify the critical data points that need to be captured and analyzed.
- Establish Data Standards: Once the critical data elements have been identified, it’s important to establish data standards to ensure that the data is consistent and accurate. This may involve defining data dictionaries, validation rules, and quality standards.
- Use Data Integration Tools: There are various data integration tools available that can help businesses integrate data from different sources, such as ETL (Extract, Transform, Load) tools and data integration platforms. These tools can automate the data integration process and help ensure the data is accurate and consistent.
- Normalize Data: Different types of data may be stored in different formats or structures. Normalizing the data involves converting it into a standard form or network, making it easier to integrate and analyze.
- Use Advanced Analytics Techniques: Analyzing different data types often involves using advanced analytics techniques such as data mining, predictive modeling, and machine learning. These techniques can help uncover patterns and trends in the data that may not be apparent using traditional analysis methods.
- Data Visualization: Data visualization is essential to analyzing different data types. It involves presenting the insights from data analysis in a clear and understandable format, such as charts, graphs, and dashboards.
Integrating and analyzing different data types requires a strategic approach that involves identifying critical data elements, establishing data standards, using data integration tools, normalizing data, using advanced analytics techniques, and presenting insights using data visualization.
By following these best practices, businesses can gain valuable insights from their data and optimize their operations to drive growth and success.
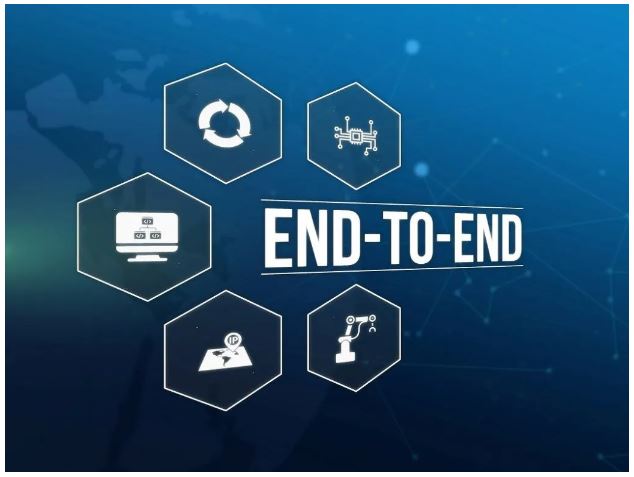
Implementing End-to-End Analytics
Implementing end-to-end analytics involves a strategic approach that involves several key steps. Here are the steps involved in implementing end-to-end analytics:
- Identify Business Objectives: The first step in implementing end-to-end analytics is to identify the business objectives that the organization wants to achieve. This involves working with stakeholders from across the organization to identify key performance indicators (KPIs) and metrics that will be used to measure success.
- Identify Relevant Data Sources: Once the business objectives have been identified, the next step is identifying the relevant data sources used to measure performance. This may include data related to customer interactions, operational data, sales transactions, web analytics, and financial data.
- Develop Data Strategy: Once the relevant data sources have been identified, the next step is to develop a strategy outlining how the data will be captured, stored, integrated, and analyzed. This involves identifying the tools and technologies used to manage the data and the processes and workflows used to integrate and analyze the data.
- Build Analytics Infrastructure: Once the data strategy has been developed, the next step is to build the analytics infrastructure. This involves implementing the tools and technologies that will be used to manage and analyze the data, such as data integration platforms, data warehouses, and analytics tools.
- Analyze Data: Once the analytics infrastructure has been built, the next step is to analyze the data using various techniques such as data mining, predictive modeling, and machine learning. The insights gained from the analysis can be used to optimize business processes, improve operations, and drive growth and profitability.
- Communicate Results: The final step in implementing end-to-end analytics is communicating the analysis results to organizational stakeholders. This involves presenting the insights from the study in a clear and understandable format, such as charts, graphs, and dashboards.
Implementing end-to-end analytics requires a strategic approach that involves identifying business objectives, identifying relevant data sources, developing a data strategy, building an analytics infrastructure, analyzing data, and communicating results. By following these steps, businesses can leverage the insights hidden within their data to drive growth and success in their industry.
Developing an end-to-end analytics framework based on business goals and objectives
Developing an end-to-end analytics framework involves creating a strategic approach to data analysis aligned with the organization’s business goals and objectives. Here are the critical steps involved in developing an end-to-end analytics framework:
- Define Business Goals and Objectives: The first step in developing an end-to-end analytics framework is to define the business goals and objectives. This involves working with stakeholders from across the organization to identify the key performance indicators (KPIs) and metrics that will be used to measure success.
- Identify Relevant Data Sources: Once the business goals and objectives have been identified, the next step is to identify the relevant data sources that will be used to measure performance. This may include data related to customer interactions, operational data, sales transactions, web analytics, and financial data.
- Develop a Data Strategy: The next step is to develop a strategy outlining how the data will be captured, stored, integrated, and analyzed. This involves identifying the tools and technologies used to manage the data and the processes and workflows used to integrate and analyze the data.
- Build Analytics Infrastructure: Once the data strategy has been developed, the next step is to build the analytics infrastructure. This involves implementing the tools and technologies that will be used to manage and analyze the data, such as data integration platforms, data warehouses, and analytics tools.
- Analyze Data: The next step is to analyze the data using data mining, predictive modeling, and machine learning techniques. The insights gained from the analysis can be used to optimize business processes, improve operations, and drive growth and profitability.
- Communicate Results: The final step in developing an end-to-end analytics framework is communicating the analysis results to organizational stakeholders. This involves presenting the insights from the research in a clear and understandable format, such as charts, graphs, and dashboards.
Developing an end-to-end analytics framework involves aligning data analysis with the business goals and objectives of the organization. By following these steps, businesses can leverage the insights hidden within their data to drive growth and success in their industry.
Leveraging automation tools and workflows to streamline data collection and analysis
Leveraging automation tools and workflows is an effective way to streamline data collection and analysis. Here are some of the benefits of using automation tools and workflows for data collection and analysis:
- Efficiency: Automation tools and workflows can help businesses collect and analyze data more efficiently by eliminating the need for manual data entry and analysis.
- Accuracy: Automation tools and workflows can help ensure data accuracy by reducing the risk of errors during manual data entry.
- Scalability: Automation tools and workflows can be scaled to handle large volumes of data, making it easier to process and analyze large datasets.
- Consistency: Automation tools and workflows can ensure that data collection and analysis are consistent across different departments and business units.
- Time-saving: Automation tools and workflows can help businesses save time by automating repetitive tasks such as data entry and report generation.
Some examples of automation tools and workflows that can be used for data collection and analysis include:
- Data Extraction and Transformation Tools: These tools can help businesses extract data from various sources and transform it into a usable format for analysis.
- Data Cleaning and Validation Tools: These tools can help businesses clean and validate data to ensure accuracy and consistency.
- Machine Learning and Artificial Intelligence Tools: These tools can automate the data analysis process and uncover patterns and trends in the data.
- Reporting and Dashboard Tools: These tools can be used to automate the generation of reports and dashboards, making it easier to communicate insights to stakeholders across the organization.
Leveraging automation tools and workflows can help businesses streamline data collection and analysis, save time and resources, and uncover valuable insights that can drive growth and success in their industry.
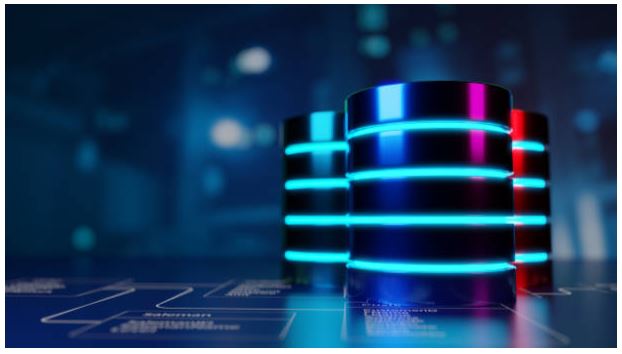
Addressing common challenges and obstacles in implementing end-to-end analytics, such as data silos and privacy concerns
Implementing end-to-end analytics can be challenging, requiring addressing various technical and organizational issues. Here are some common challenges and obstacles that businesses may face when implementing end-to-end analytics and some strategies for addressing them:
- Data Silos: One of the biggest challenges in implementing end-to-end analytics is the presence of data silos. Data silos refer to the situation where different departments or business units store data separately, making it difficult to integrate and analyze. To address this challenge, businesses should consider implementing data integration tools and establishing data governance policies that ensure that data is captured and stored in a consistent and standardized manner.
- Privacy Concerns: With the increasing importance of data privacy regulations such as GDPR and CCPA, businesses need to ensure that they are collecting and analyzing data in a way that complies with these regulations. Companies should consider implementing privacy-by-design principles that guarantee that data is collected and analyzed in a way that respects user privacy.
- Lack of Data Literacy: Another common challenge in implementing end-to-end analytics is employees need more data literacy. To address this challenge, businesses should invest in data literacy training programs that provide employees with the skills and knowledge they need to work with data effectively.
- Technical Challenges: Implementing end-to-end analytics can be technically challenging, particularly when integrating different data sources and analyzing data at scale. To address these challenges, businesses should consider working with experienced data professionals who can help them design and implement the necessary infrastructure and processes.
- Organizational Resistance: Finally, end-to-end analytics can sometimes face resistance from employees who are comfortable with existing working methods. To address this challenge, businesses should consider implementing change management processes involving communication, training, and collaboration to ensure all stakeholders are aligned and invested in the project’s success.
Addressing these common challenges and obstacles requires a strategic approach involving collaboration, technology and training investment, and a commitment to data governance and privacy principles. By following these strategies, businesses can overcome these challenges and unlock the full potential of end-to-end analytics to drive growth and success.
Final Thoughts
End-to-end analytics is a powerful approach to data analysis that can help businesses gain valuable insights and optimize their operations.
By following best practices such as identifying relevant data sources, developing a data strategy, building an analytics infrastructure, analyzing data, and communicating results, businesses can leverage the insights hidden within their data to drive growth and success.
However, implementing end-to-end analytics can be challenging. It requires addressing various technical and organizational issues such as data silos, privacy concerns, lack of data literacy, technical challenges, and organizational resistance.
By addressing these challenges with a strategic approach that involves collaboration, investment in technology and training, and a commitment to data governance and privacy principles, businesses can overcome these obstacles and unlock the full potential of end-to-end analytics.